Abstract
Purpose
In Morocco, universities have opted for online courses as a national effort to contain the coronavirus since the early stage. This article aims to analyze university students’ satisfaction with their distance learning experience during the COVID-19 pandemic.
Design/methodology/approach
This article is based on the statistical analysis of a survey conducted in 2020 at the national level among 800 university students from all disciplines enrolled in open access and regulated access institutions in Morocco. Econometrically, the authors used the ordinal logistic regression model after checking several conditions justifying its use.
Findings
Findings from analysis reveal that students lacking basic computer skills have a negative perception of this new mode of learning. The results also show a certain sensitivity of the students to the way in which the courses are taught. The satisfaction of the learners largely depends on the duration of the courses, the interactivity, and the teaching methods adopted. In designing, developing, and delivering distance education courses, students’ needs should be taken care of.
Originality/value
This article suggests that for public policies, professional training for teachers should equip them with the pedagogical skills so that they can adjust their teaching according to students’ levels. Training in digital tools must also be provided to bridge the digital divide and accelerate positive change and adaptation to this new mode of training. In addition, this article highlights the role of interactivity in increasing adaptation to distance learning.
Keywords
Citation
Zouiri, L. and Kinani, F.E. (2022), "An analysis of students’ satisfaction with distance learning in Moroccan universities during the COVID-19 pandemic", Public Administration and Policy: An Asia-Pacific Journal, Vol. 25 No. 3, pp. 293-309. https://doi.org/10.1108/PAP-08-2022-0102
Publisher
:Emerald Publishing Limited
Copyright © 2022, Lahboub Zouiri and Fatima Ezzahra Kinani
License
Published in Public Administration and Policy. Published by Emerald Publishing Limited. This article is published under the Creative Commons Attribution (CC BY 4.0) license. Anyone may reproduce, distribute, translate and create derivative works of this article (for both commercial and non-commercial purposes), subject to full attribution to the original publication and authors. The full terms of this license may be seen at http://creativecommons.org/licences/by/4.0/legalcode
Introduction
Apart from the severe impact on public health and well-being, the chain effect resulting from the COVID-19 pandemic is a profound disruption for various other sectors, notably in education. COVID-19 has driven massive transformation in many aspects of the educational landscape, particularly as teaching and learning shifted to online mode due to school closure. As an example, the job loss in the aftermath of pandemic has brought difficulty for low-income households. This group is perceived to have low resources for loss recovery and may not prioritize health and education in rebuilding after a health crisis. Closures of learning spaces affected 94 percent of the world’s school-age population, and up to 99 percent in low- and lower-middle-income countries (UNSDG, 2020).
At the same time, the pandemic has stimulated innovation in the education sector. In Morocco, universities have adopted online courses as a national effort to contain the Coronavirus at an early stage. Many universities have had to convert what remains of their normally face-to-face classes into online courses. However, far from being a miracle solution, distance learning may exacerbate certain social inequalities. Such a mode of education cannot succeed by ignoring the disparities of supply (public/private), environment (urban, peri-urban, rural, and remote rural), and gender (boy/girl). For this purpose, the shortage of equipment should not be underestimated (lack of essential computer equipment, difficulty in accessing the Internet or massive influx of connections, etc.). In addition, the immediate and rapid switch to digital technology reveals the limits of teacher training, the degree of complementarity between school textbooks and online resources, and finally, the case of students who are disoriented because they are left to their own devices and lack the support of educated adults.
The objective of this article is to identify the determining factors of the distance learning student’s satisfaction in Moroccan universities. The authors approached this question through an analysis of the satisfaction of 800 students in Moroccan universities during 2020. Satisfaction, often presented as a sign of the quality of distance education, will be measured by different indicators particularly pedagogical quality of the courses, availability of teachers, guidance, and assistance. An econometric study using the ordered logit model to determine the factors influencing students’ satisfaction with distance education in Morocco is conducted.
The conceptual framework of distance education
Open and Distance Learning has gained a new breath with the turn of the 21st century with more and more courses delivered through distance education models worldwide. The impact of the new media, particularly digital connective technologies to deliver courses from a distance has triggered a new interest towards open and distance learning opportunities including the advent of Open Education Resources (OER) and Massive Online Open Courses (MOOCs) that attempt to provide learning access to a wider audience. Distance education has been engineered and reengineered by the techno-social changes in the society. Besides, the practices, philosophies and cultures of people developing open and distance learning have impacted how it is designed and conducted (Saykili, 2018).
Generally speaking, research studies struggle to find a positive effect of distance education on learner satisfaction. It depends not only on the characteristics of the learners, but also on the types of courses which are offered. Carr (2000) indicates, for example, lower student satisfaction with distance learning can be attributed to the fact that it takes them much longer than full-time students to complete their training. Rivera et al. (2002) point out that students who are least satisfied with distance learning are particularly those who have less mastery of the tool’s technologies. Summers et al. (2005) also report lower satisfaction based on feedback from student evaluation surveys and exchanges between students. Other studies have found positive effects of distance education under certain conditions depending on the motivation for this type of teaching and expertise in online training.
Although technology is an integral part of distance education, any successful program must focus on the instructional needs of the students, rather than on the technology itself. It is essential to consider their age, cultural and socioeconomic backgrounds, interests and experiences, educational levels, and familiarity with distance education methods.
According to Sherry (1995), the most important factor for successful distance learning is a caring, concerned teacher who is confident, experienced, comfortable with the equipment, uses the media creatively, and maintains a high level of interactivity with the students.
Public policy: a response
The shock to education systems has had, and will continue to have, significant effects beyond the educational sphere. The COVID-19 pandemic threatens education progress worldwide through two major shocks: the near-universal school closures at all levels and the economic recession sparked by pandemic-control measures. The health crisis is exacerbating existing educational disparities, with many of the most vulnerable children, youth, and adults at risk of not returning to school. The lost learning time not only harms the current generation but could also reverse decades of progress. To counteract this heavy fallout, distance learning options have been developed through the rapid intervention of governments and partners around the world committed to ensuring educational continuity.
In Morocco, the High Commission for Planning conducted a household survey in April 2020 to monitor the adaptation of household lifestyles under the constraint of confinement. The results show that the platforms set up by higher education institutions are used by 37 percent of households with higher education students. As for satisfaction with this new education landscape, 59 percent of higher education students are moderately or not at all satisfied (HCP, 2020).
Other experts focused on the pedagogical integration of Information and Communication Technology (ICT). The pandemic revealed the importance of ICT and the multiple benefits it could bring to the education system. The pedagogical use of ICT has developed and affected the entire learning process and pedagogical communication. The pandemic has forced the students to develop autonomy and self-discipline in the absence of the physical presence of the teachers (Rechidi and Bennani, 2020).
For other experts in Morocco, the failure of distance education is obvious, as evidenced by the government’s decision to consider as the only effective learning that took place before the schools closed. Thus, the baccalaureate exam, the symbol of the system, is maintained, but its scope is limited to lessons taught before the schools closed. A colossal pedagogical effort is required of the actors in education, and first and foremost the teachers, to remotivate the students and bring them to a level of learning that will reassure them again throughout their training (Naji, 2020). Also, the implementation of an effective ‘distance learning’ system remains difficult to date despite the significant efforts that have been made. According to the survey conducted by Elmendilia and Saaidi (2020), 30 percent of the respondents seem dissatisfied with the distance learning experience.
Research methodology
The first part of this section will briefly present the characteristics of higher education in Morocco and then describe the survey which is the basis of this study. The rest of the section will present the econometric model with its characteristics and assumptions.
Presentation of the survey
a. Higher education in Morocco
The higher education system in Morocco consists of 423 establishments, including 148 public universities. In terms of students’ population, the total number of students in the whole system has increased from 2020 to 2021 by approximately 7.5 percent growing from 1,009,596 to 1,085,064 students. Public universities are the majority with more than 90 percent of the students. In terms of teaching staff, the higher education system in Morocco has 20,771 teachers, including 15,325 in public universities.
b. Methodology of the survey
This study is based on the statistical analysis of a survey conducted by Zouiri, El Marhoum, and Ezzahid (2020). The survey is a sample survey, and similar to any survey of this kind, it requires a sampling frame which is for the case of this survey, the list of all students enrolled in Moroccan institutions. Given the difficulty of data collection, the authors opted for quota sampling method. The quota method is a non-probability sampling method which is considered as the best substitute for probability methods in the case where the latter would encounter sampling frame constraints. Quota sampling involves studying the structure of the population according to empirically chosen criteria (quotas). The sample is then constructed in such a way to constitute a miniature reproduction of the population.
The proposed sampling plan involves representing the population of students according to a certain number of criteria in order to ensure a certain representativeness of the population, each of the criteria is applied according to the weight of its modalities in the population. The selected criteria are the type of establishment, type of access, and number of students by university. The weights used correspond to the structure of the student population in Morocco for the 2018/19 academic year.
The collection method used is online self-administration by email and by sharing on university social networks. A daily follow-up of the answers was ensured to check the representativeness of the sample according to the three fixed criteria (type of establishment, type of access, and type of university). In the event of underrepresentation of a category, a follow-up is carried out to straighten the sample. All data analysis was performed using Statistical Product and Service Solutions (SPSS) software. The size of the sample surveyed was stopped once the desired representativeness was reached, which is a total sample of 3,358 students. The survey was conducted between 22 May and 5 July 2020.
c. Main results of the survey
Overall, the students surveyed are familiar with basic computer tools. Only a very small percentage, i.e., 9 percent of students reported low familiarity with these tools (Figure 1).
The majority of students (83 percent) report taking courses remotely during the lockdown. These students primarily use smartphones or personal computers. Indeed, 65.9 percent and 60.3 percent of respondents report using these two tools respectively. It should be noted that during the COVID-19 lockdown, the use of distance learning was not a choice; it was the only option offered to students to continue their learning and complete their academic year (Figure 2).
The proportion of dissatisfied students exceeds 45 percent for the five aspects on which they are questioned. The two areas in which students are most dissatisfied are interactivity and the amount of work with teachers (Figure 3). When asked about their overall satisfaction, only 42.77 percent of students said they were satisfied or very satisfied (Figure 4).
Only 40 percent of students reported that they were accompanied by their institution in distance learning (Figure 5). In case of problems, students manage on their own in 64 percent of cases.
More than 56 percent of students report that they are poorly or not at all adapted to distance learning (Figure 6). This is related to their overall dissatisfaction.
50.42 percent of the students prefer face-to-face teaching and 42 percent opt for hybrid teaching. Only 7.53 percent prefer distance learning (Figure 7). More than 65 percent think that distance learning cannot replace face-to-face teaching (Figure 8).
Presentation of the econometric model
a. Selected variables
One of the methods used to evaluate the effectiveness of distance education includes evaluating the satisfaction of students (Burns, 2013). The authors aim to explain students’ satisfaction with their distance learning experience during the COVID-19 pandemic as a function of several demographic and social characteristics such as gender and place of residence, and as a function of cognitive characteristics such as the degree of mastery and familiarity of students with the different distance learning tools. This study also links the students’ perception of distance learning to educational practices deployed by the institution, such as pedagogical support for the appropriation of the different tools used. Responses were collected from 800 university students from all disciplines enrolled in open access and regulated access institutions in Morocco. Indeed, the model constructed in ordinal logistic regression is a proportional odds model, requiring the respect of the condition of parallel regression or proportionality of odds. The above condition is sensitive to large sample sizes and subsequently rarely verified.
As for the variable to be explained, the dependent variable measures overall student satisfaction with distance learning, on a scale from 1 to 4 as follows:
1: the student is very dissatisfied,
2: the student is dissatisfied,
3: the student is satisfied,
4: the student is very satisfied.
For the independent variables, the explanatory variables used in the model are presented below and can be distinguished between binary and polytomous variables:
− Gender: binary variable (1: male, 0: female).
− Place of residence: binary variable (1: student lives in urban area, 0: otherwise).
− Type of institution: binary variable (1: student is enrolled in an open access institution, 0: otherwise).
− Level of education: polytomous variable (1: the student is in the first year, 2: the student is in the second year, 3: the student is in the third year, 4: the student is in the fourth year (Master 1), 5: the student is in the fifth year or above (e.g., Master’s, PhD)).
− Training follow-up: binary variable (1: the student has already followed a distance learning training before the lockdown, 0: otherwise).
− Cost of connection: polytomous variable (1: the student judges that the cost of connection is not high at all, 2: the student judges that the cost of connection is low, 3: the student judges that the cost of connection is high, 4: the student judges that the cost of connection is very high).
− Degree of familiarity to distance learning tools: polytomous variable (1: low, 2: medium, 3: high, 4: very high).
− Level of mastery of distance learning tools by the student: polytomous variable (1: low, 2: medium, 3: high, 4: very high).
− Support by the university: binary variable (1: the student is supported by the institution when he encounters technical problems during distance learning, 0: otherwise).
− Pedagogical content: polytomous variable (1: the student is very dissatisfied with the pedagogical content, 2: the student is dissatisfied with the pedagogical content, 3: the student is satisfied with the pedagogical content, 4: the student is very satisfied with the pedagogical content). This variable reflects the theoretical knowledge that a learner can acquire on a given subject.
− Teaching methods: polytomous variable (1: student is very dissatisfied with teaching methods, 2: student is dissatisfied with teaching methods, 3: student is satisfied with teaching methods, 4: student is very satisfied with teaching methods). This variable reflects the principles and methods used by teachers.
− Interactivity: polytomous variable (1: the student is very dissatisfied with the interactivity, 2: the student is dissatisfied with the interactivity, 3: the student is satisfied with the interactivity, 4: the student is very satisfied with the interactivity). This variable concerns student-teacher interaction during class.
− Course length: polytomous variable (1: student is very dissatisfied with the course length, 2: student is dissatisfied with the course length, 3: student is satisfied with the course length, 4: student is very satisfied with the course length).
b. Presentation of the ordinal logistic regression
For the multivariate approach adopted in this study, the authors applied ordinal logistic regression using STATA software. The ordered logit model is a variant of the logit models. It estimates the relationship between an ordinal dependent variable and several independent variables. The condition required for the use of the ordinal logit model is that the modalities of the dependent variable must have an orderly relationship between them and be classified according to a given order. Apart from the ordinal character, another condition must be fulfilled for the ordered logit to be applied. The number of terms must be greater than two, otherwise the ordered logit model will be confused with the binary logit model.
The ordered logit model is similar to the multinomial logit model. The only difference between the two is the nature of the dependent variable. The dependent variable is ordinal for the former and polytomous for the latter. The use of a multinomial logit model, when the dependent variable is ordinal, introduces a bias in the results by the fact that the multinomial logit does not take into account the ordinal character of the modalities of the dependent variable.
The dependent variable reflects the degree of satisfaction of students who took distance education courses during the lockdown period. Thus, to meet the requirements of the model, the authors have classified its modalities according to a numerical scale: “very dissatisfied = 1”, “dissatisfied = 2”, “satisfied = 3”, “very satisfied = 4”.
Assume that the underlying process to be characterized is:
Where y* is the exact but unobserved dependent variable; x is the vector of independent variables, ε is the error term, and β is the vector of coefficients of the regression to estimate. Suppose further that, although y* cannot be observed, the response categories can be observed.
Thus, the probability of realizing the event y ∈ {1, 2, 3, 4} of the dependent variable corresponds to the probability of belonging to the interval bounded by estimated intersection points corresponding to each of the modalities of the dependent variable (the u ends). After simplification, the probability of y is given by:
The error term Ui is assumed to be logistically distributed.
The coefficients of the linear combination β cannot be consistently estimated using ordinary least squares. They are usually estimated using maximum likelihood.
Apart from the coefficients, the “ordinal logit” model provides, among other things, the Chi-square statistic, the Chi-square probability attached to the model, the pseudo R-square, and the significance level of the parameters.
The interpretation of the results of the ordinal logistic modeling concerns two series of elements: the validity and likelihood of the model on the one hand, and the respective influences of the explanatory variables on the explained variable on the other. The likelihood of the model is assessed using the significance of the Chi-square statistic attached to it. The degree of adequacy of the model is given by the coefficient of determination, which is also termed the pseudo R-square.
Results and interpretations
One of the assumptions underlying ordered logistic regression is that the relationship between each pair of outcome groups is the same. In other words, ordered logistic regression assumes that the coefficients that describe the relationship between, for example, the lowest and all higher categories of the response variable are the same as those that describe the relationship between the next lowest category and all higher categories, and so on. This is called the proportional odds hypothesis or the parallel regression hypothesis. Since the relationship between all pairs of groups is the same, there is only one set of coefficients (one model). If this were not the case, different models would be needed to describe the relationship between each pair of outcome groups.
Thus, the authors need to test the proportional odds hypothesis. The insignificant value (p > 0.05) of the Chi-square test shows that this model does meet the condition of parallel regression or proportional odds (see Appendix 1). In this respect, the use of the ordinal model is well justified.
For this model, the results of the likelihood ratio tests show a very significant critical probability (p-value) at the 1 percent level, allowing the authors to reject the null hypothesis of equality of the coefficients (see Appendix 2). The model is hence globally significant and can therefore be used in the analyses. The criterion of the pseudo R-square is close to 23 percent which shows that this model brings 23 percent more improvements compared to the null model. The pseudo R-square of the logistic models are not expected to reach high levels similar to the R-square of the linear regression. Indeed, a pseudo R-square between 20 percent and 40 percent reflects that the goodness of fit of the model is very good.
The below summarizes all the outputs obtained by the STATA software; it includes the estimated results taking into account the variables likely to be main determinants of distance learning students’ satisfaction (Table 1).
Demographic and social characteristics
The results of the ordered logistic model estimations show that demographic or social characteristics do not appear to impact distance learning student’s satisfaction. The variables of gender and place of residence are not significant in this model. The insignificance of those variables can be explained by the changing profile of the distance learning student with the evolution of training methods and technologies. If the studies converge on a typical profile of the distance learning student (older face-to-face students, more frequently female, married with children, and employed), it seems to have evolved quite noticeably these recent years (Dabbagh, 2007). This audience is becoming much more heterogeneous, younger, and more responsive to new information technologies and communication.
On the other hand, the level of education attained by the students seems to have an impact on their satisfaction. Indeed, students in their fifth year or higher are more likely to be satisfied with distance education than students in their first year of university. They have acquired a certain level of autonomy with respect to time management and organization which allows them to appreciate distance learning.
Digital tools and technology
Regarding the financial context of the student, the variable, cost of connection is considered as a variable reflecting the financial difficulties encountered by the student. The modeling results show that this variable is insignificant. This can be explained by the collaborations made with the telephone operators and competitive offers given by the latter to ensure access to education. It should be noted that the variables reflecting the financial conditions of the students may not be significant since Morocco has offered financial aid to support the different categories of people whose purchasing power and living conditions have been impacted by state of health emergency measures.
The results of the survey show that the majority of students surveyed (83 percent) reported taking courses remotely during the lockdown. These students primarily use smartphones or personal computers. The distance learning mode requires special training technologies, course design, and communication means based on electronic or other technology. Indeed, students who do not master digital tools had difficulties learning, and hence might not be enthusiastic about taking online courses (Xie et al., 2006).
The variable, degree of familiarity had a negative coefficient. This result, which is not supported by the literature, can be explained by the conditions in which the distance learning was carried out. The time lost by teachers adapting to digital tools and solving technical problems can frustrate students who are already familiar with digital tools and thus dampen satisfaction.
Educational methods
The “support” variable is significant in this model. The institution’s pedagogical assistance, which is based on the singularity of people in their individual and collective dimensions which reinforces the students’ satisfaction with this new learning mode. Unfortunately, the results of the survey and the source of this study, reveal that only 40 percent of the students declared that they were supported technically by their institution in distance learning. When problems arise, most students manage to resolve the technical problems on their own (64 percent) or use the internet. The work of Giret (2020) shows that distance learning students are a little less satisfied compared to their face-to-face counterparts. This lower satisfaction is attributed to two reasons, the availability of teachers and assistance with orientation and professional integration. Support is thus a key variable differentiating between face-to-face teaching and distance education.
The results of the ordinal logistic regression also show sensitivity of the students to the way in which the courses are taught. The satisfaction of the learners largely depends on the duration of the courses, interactivity, and teaching methods adopted. In designing, developing, and delivering distance education courses, students’ needs, and perceptions are of paramount importance. A course failing to meet student expectations and needs may lead to low levels of student involvement (Sahin and Shelley, 2008).
The coefficients calculated using ordinal logistic regression do not reflect the magnitude of the relationship between target variable and independent explanatory variables. These coefficients only capture the direction of the relationship. Thus, the authors used Odds Ratio (OR) which is a statistical measure expressing the degree of dependence between qualitative random variables. They are used in Bayesian inference and logistic regression, measuring factor effects (Table 2).
Demographic and social characteristics
The above results show that students in their fifth year or beyond are about 2.3 times more likely to be satisfied with distance learning than first year students who find themselves disoriented, unprepared for a new learning system, and in need of guidance and assistance in setting their pace within the academic world. The distance becomes an obstacle to good teaching delivery for first-year students at university.
Digital tools and technology
The success of distance learning depends on the mastery of adequate technological equipment and the student’s degree of familiarity with the world of distance learning. Indeed, students who declare to have mastered ICT of distance learning are twice as likely to be satisfied with this mode. In addition, learners who have already taken a distance education course are 1.4 times more likely to be satisfied with distance learning than those who have never had the opportunity to undertake a distance education course.
Educational methods
Distance learning requires a comprehensive team of teachers, administrators, and technicians who operate at teacher-student interfaces to facilitate learning. To this end, the support and assistance provided by institutions have a significant impact on students’ satisfaction. The authors estimate that learners who have been supported by their institutions are 1.9 times more likely to experience satisfaction with distance learning.
Relative to the teaching methods variable, students who reported high satisfaction with educational practices are 3 times more likely to rate distance learning favorably. Indeed, the task of designing a distance course was recognized by some as the most critical part related to students’ success. In face-to-face settings, designing a course is already a complex process with various grey areas. In distance learning, the job is even more difficult. The teacher is therefore no longer just a ‘programmer’ or a communicator, but also a learning facilitator and must take particular care in the sequencing of courses.
As for the interactivity variable, the lack of feedback, isolation, and anxiety may hinder the success of distance learning during lockdowns due to the absence of teacher-learner interaction, which is a demotivating factor. Indeed, students who were able to interact with teaching staff were very satisfied and 9 times more likely to appreciate the distance learning system.
The course must allow for sufficient time and duration to facilitate learners to consolidate their understanding and assimilation of course content. Satisfaction with this variable strongly influences the appreciation of distance learning. Students who were very satisfied with the length of the course were 5 times more likely to adapt well to distance learning and subsequently experience a high degree of satisfaction.
Conclusion and recommendations
Education is not only the foundation on which egalitarian and inclusive societies are built, but also one of the main drivers of development. Ensuring educational continuity during school closures has become a priority for governments around the world. Understanding students’ satisfaction regarding distance learning is the first step in developing an efficient online learning environment.
The results of the econometric modeling highlight the important role of interactivity in addition to efficient teaching methods, and institutional support is one of the main key factors conducive to satisfactory experience of distance learning. National policies must therefore pay special attention to how courses are designed and delivered. In fact, according to Martins (2011), the continuous training of teachers and academic advisers in distance higher education has an impact on the transmission of knowledge and skills. From the outset of the pandemic, the health crisis revealed that pre-service and in-service teacher training should be revised to better prepare teachers for new forms of teaching.
The comparison between the choices adopted by Morocco and the international trends in terms of teacher training, make it possible to observe, on the one hand, that Morocco is part of these international trends by adopting the principle of “universitarization” of initial teacher training and on the other hand, the country is also committed to applying the principle of professionalization of training (Lahchimi, 2015). According to Lahchimi, despite the difference noted between the objectives of the initial training and professional qualification, 82 percent of trainees claim a redundancy of activities between initial university training and professional qualification. The trend towards face-to-face lectures seems to continue to predominate in modular training (according to 87 percent of teachers surveyed). Thus, teachers must be trained in digital tools and receive a deep professional development in order to bridge the digital divide, accelerate positive change, and manage to adapt their pedagogical skills to various levels of students.
With regard to student support, national policies should incorporate counselling into their plans, manuals, and guides for teachers and students to provide additional support. Without such support, the pressure can lead to burnout, which increases absenteeism and dropout, and may even cause some to leave their jobs or institutions. This may in turn undermine efforts to build institutional resilience of the education system.
As for the interactivity variable, without connectivity, the student may become autonomous and isolated. He/she may even procrastinate and eventually drop out. Interactivity takes many forms. It is not just limited to audio and video formats, nor solely to teacher-student interactions. It represents the perceived connectivity between students and distance learning teachers, local teachers, aides, facilitators, and peers (Sherry, 1995). The desire to succeed in distance learning has led to various higher education initiatives to create mechanisms for interaction and support among peers, sometimes just by suggesting and sometimes imposed on students, especially in the form of cooperative or collaborative learning.
The logistic regression used in this article reveals that the lack of basic computer skills in some students may make it difficult for them to ensure their own professional development, which may lead to a negative perception of this new form of learning. Students who use digital tools in their personal and professional lives are more comfortable and familiar with the online learning environment. Policymakers and managers must work to remove barriers to technology by investing in digital infrastructure and lowering the costs of accessing networks.
From this point of view, the appropriation of techno-pedagogical devices by teachers and learners, accompanying effects of these devices on pedagogical options, practices, professional development, and performance of learning are without doubt new indicators of competences that should be integrated into an innovative reference system.
The unprecedented health crisis has also highlighted the need for a flexible education system based on hybrid learning. These hybrid models ensure not only strong articulation between different levels and types of education, but also have the capacity to implement other learning models. Hybrid learning, which offers students flexible and quasi-individualized learning pathways, combines various pedagogical approaches, and makes use of alternative learning resources.
This study helped understand the determinants of students’ satisfaction, which is a perceived indicator of quality of distance education. However, it needs to be further enhanced based on the comparison between the success rates of students in distance education and those of face-to-face education. To control or confounding variables, such comparisons should be made based on comparable populations, same examinations, same lessons, and same teachers. This could capture the internal efficiency of distance education.
Figures
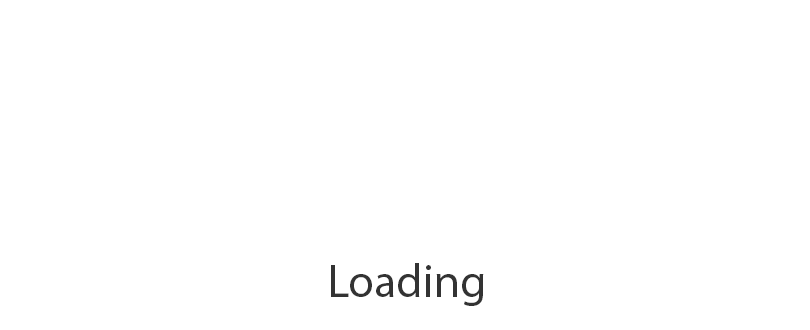
Figure 1
Level of familiarity with basic computer tools
Source: Adapted from the student survey on distance education in Morocco by Zouiri et al. (2020)
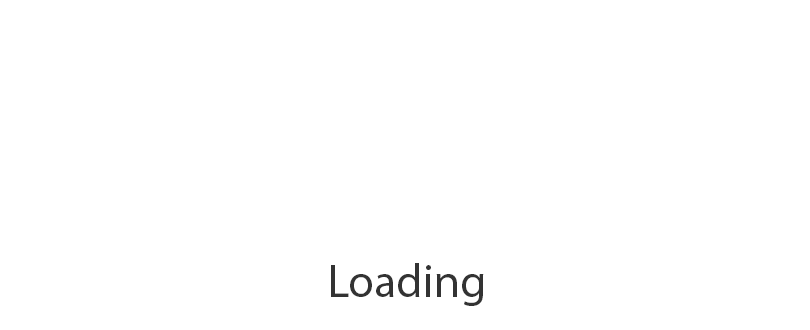
Figure 2
Students who received distance learning during lockdown
Source: Adapted from the student survey on distance education in Morocco by Zouiri et al. (2020)
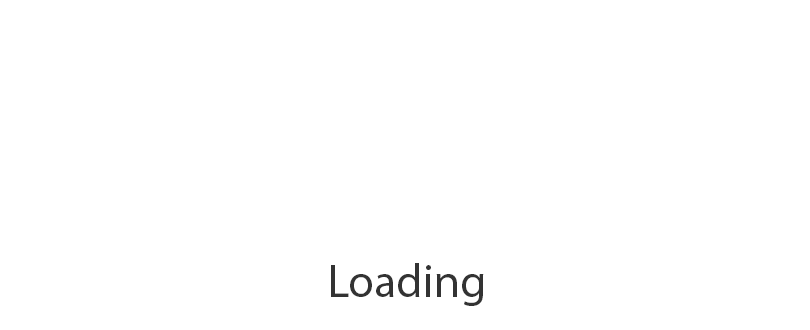
Figure 3
Dimensions of distance learning and students’ satisfaction
Source: Adapted from the student survey on distance education in Morocco by Zouiri et al. (2020)
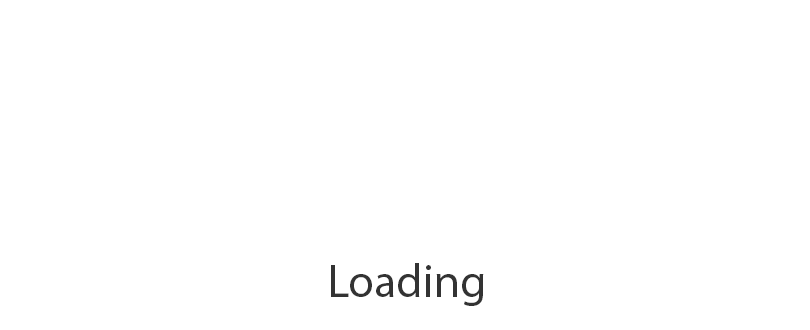
Figure 4
Degree of overall satisfaction
Source: Adapted from the student survey on distance education in Morocco by Zouiri et al. (2020)
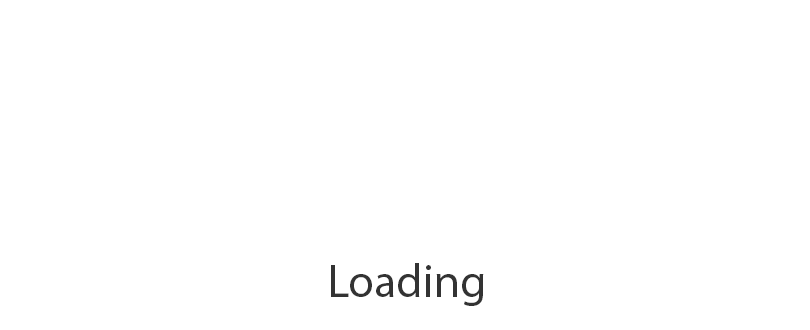
Figure 5
Accompanied by the university in the distance learning
Source: Adapted from the student survey on distance education in Morocco by Zouiri et al. (2020)
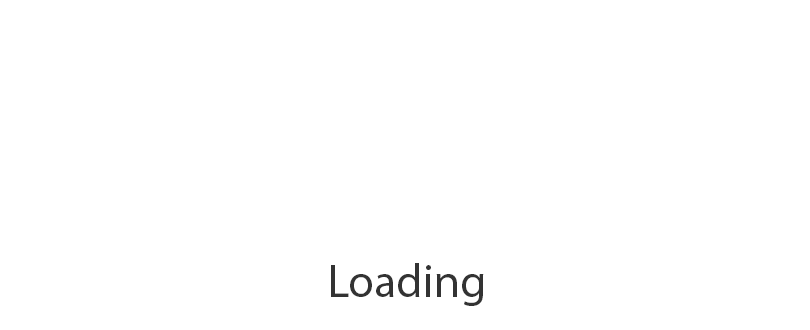
Figure 6
Adaptation to distance learning
Source: Adapted from the student survey on distance education in Morocco by Zouiri et al. (2020)
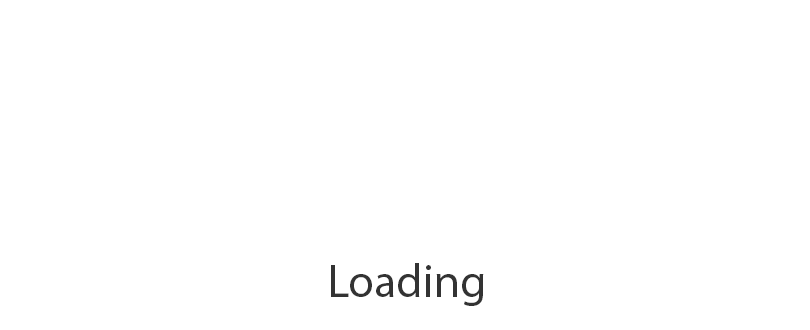
Figure 7
Preferred mode of education
Source: Adapted from the student survey on distance education in Morocco by Zouiri et al. (2020)
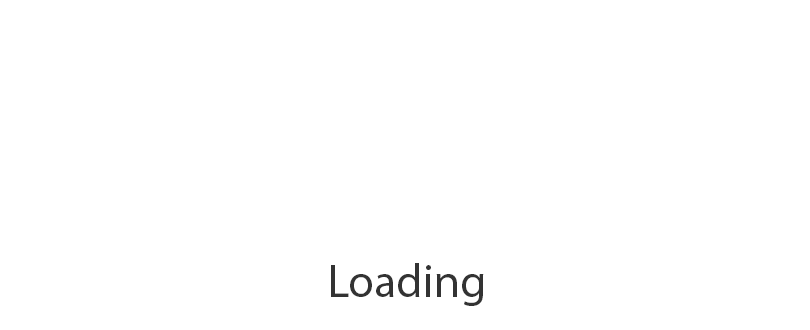
Figure 8
Possibility of replacement of face-to-face teaching by distance learning
Source: Adapted from the student survey on distance education in Morocco by Zouiri et al. (2020)
Results of the ordinal logistic regression
DEGREE OF SATISFACTION | COEF. | STD. ERR. | Z | P>|Z| | [95 PERCENTCONF. INTERVAL] | |
---|---|---|---|---|---|---|
GENDER | -0.277 | 0.152 | -1.820 | 0.069 | -0.576 | 0.022 |
PLACE OF RESIDENCE | -0.083 | 0.200 | -0.410 | 0.678 | -0.475 | 0.309 |
TYPE OF INSTITUTION | 0.012 | 0.202 | 0.060 | 0.951 | -0.384 | 0.409 |
LEVEL OF EDUCATION | ||||||
2 | -0.132 | 0.268 | -0.490 | 0.622 | -0.657 | 0.393 |
3 | 0.559 | 0.248 | 2.260 | 0.024 | 0.074 | 1.045 |
4 | 0.330 | 0.241 | 1.370 | 0.171 | -0.142 | 0.802 |
5 | 0.825 | 0.255 | 3.230 | 0.001 | 0.325 | 1.325 |
FORMATION FOLLOW-UP | 0.355 | 0.155 | 2.290 | 0.022 | 0.052 | 0.659 |
COST OF CONNEXION | 0.016 | 0.077 | 0.210 | 0.836 | -0.134 | 0.166 |
DEGREE OF FAMILIARITY | -0.219 | 0.107 | -2.050 | 0.040 | -0.428 | -0.010 |
MASTERY OF DISTANCE-LEARNING TOOLS | 0.726 | 0.120 | 6.060 | 0.000 | 0.491 | 0.961 |
SUPPORT BY THE UNIVERSITY | 0.642 | 0.152 | 4.240 | 0.000 | 0.345 | 0.939 |
PEDAGOGICAL CONTENT | ||||||
2 | -0.145 | 0.261 | -0.560 | 0.578 | -0.658 | 0.367 |
3 | 0.342 | 0.287 | 1.190 | 0.234 | -0.221 | 0.904 |
4 | 1.103 | 0.549 | 2.010 | 0.045 | 0.027 | 2.179 |
TEACHING METHODS | ||||||
2 | 0.426 | 0.233 | 1.830 | 0.068 | -0.031 | 0.883 |
3 | 1.643 | 0.294 | 5.580 | 0.000 | 1.065 | 2.220 |
4 | 1.180 | 0.533 | 2.220 | 0.027 | 0.136 | 2.224 |
INTERACTIVITY | ||||||
2 | 0.589 | 0.226 | 2.610 | 0.009 | 0.147 | 1.032 |
3 | 0.960 | 0.283 | 3.390 | 0.001 | 0.405 | 1.514 |
4 | 2.187 | 0.685 | 3.190 | 0.001 | 0.845 | 3.529 |
COURSE LENGTH | ||||||
2 | 0.015 | 0.259 | 0.060 | 0.953 | -0.493 | 0.524 |
3 | 0.269 | 0.275 | 0.980 | 0.328 | -0.270 | 0.808 |
4 | 1.624 | 0.440 | 3.690 | 0.000 | 0.761 | 2.488 |
---------------- | ------------ | ------------ | ------------ | ------------ | ------------ | ------------ |
/CUT1 | 1.586 | 0.441 | 0.721 | 2.450 | ||
/CUT2 | 4.470 | 0.472 | 3.544 | 5.395 | ||
/CUT3 | 8.388 | 0.556 | 7.298 | 9.478 | ||
---------------- | ------------ | ------------ | ------------ | ------------ | ------------ | ------------ |
Odds-Ratios of the ordinal logistic regression
DEGREE OF SATISFACTION | ODDS.RATIOS | STD. ERR. | Z | P>|Z| | [95 PERCENT CONF. INTERVAL] | |
---|---|---|---|---|---|---|
GENDER | 0.758 | 0.116 | -1.820 | 0.069 | 0.562 | 1.022 |
PLACE OF RESIDENCE | 0.920 | 0.184 | -0.410 | 0.678 | 0.622 | 1.362 |
TYPE OF INSTITUTION | 1.013 | 0.205 | 0.060 | 0.951 | 0.681 | 1.505 |
LEVEL OF EDUCATION | ||||||
2 | 0.876 | 0.235 | -0.490 | 0.622 | 0.519 | 1.481 |
3 | 1.750 | 0.433 | 2.260 | 0.024 | 1.077 | 2.842 |
4 | 1.391 | 0.335 | 1.370 | 0.171 | 0.868 | 2.229 |
5 | 2.282 | 0.582 | 3.230 | 0.001 | 1.384 | 3.762 |
FORMATION FOLLOW-UP | 1.426 | 0.221 | 2.290 | 0.022 | 1.053 | 1.932 |
COST OF CONNEXION | 1.016 | 0.078 | 0.210 | 0.836 | 0.874 | 1.181 |
DEGREE OF FAMILIARITY | 0.803 | 0.086 | -2.050 | 0.040 | 0.652 | 0.990 |
MASTERY OF DISTANCE-LEARNING TOOLS | 2.067 | 0.248 | 6.060 | 0.000 | 1.634 | 2.615 |
SUPPORT BY THE UNIVERSITY | 1.900 | 0.288 | 4.240 | 0.000 | 1.412 | 2.557 |
PEDAGOGICAL CONTENT | ||||||
2 | 0.865 | 0.226 | -0.560 | 0.578 | 0.518 | 1.444 |
3 | 1.407 | 0.404 | 1.190 | 0.234 | 0.802 | 2.469 |
4 | 3.012 | 1.654 | 2.010 | 0.045 | 1.027 | 8.834 |
TEACHING METHODS | ||||||
2 | 1.531 | 0.357 | 1.830 | 0.068 | 0.969 | 2.418 |
3 | 5.168 | 1.522 | 5.580 | 0.000 | 2.902 | 9.205 |
4 | 3.256 | 1.734 | 2.220 | 0.027 | 1.146 | 9.248 |
INTERACTIVITY | ||||||
2 | 1.802 | 0.407 | 2.610 | 0.009 | 1.158 | 2.806 |
3 | 2.611 | 0.739 | 3.390 | 0.001 | 1.499 | 4.546 |
4 | 8.905 | 6.097 | 3.190 | 0.001 | 2.327 | 34.073 |
COURSE LENGTH | ||||||
2 | 1.015 | 0.263 | 0.060 | 0.953 | 0.611 | 1.688 |
3 | 1.309 | 0.360 | 0.980 | 0.328 | 0.763 | 2.244 |
4 | 5.074 | 2.235 | 3.690 | 0.000 | 2.140 | 12.031 |
---------------- | ------------ | ------------ | ------------ | ------------ | ------------ | ------------ |
/CUT1 | 1.586 | 0.441 | 0.721 | 2.450 | ||
/CUT2 | 4.470 | 0.472 | 3.544 | 5.395 | ||
/CUT3 | 8.388 | 0.556 | 7.298 | 9.478 | ||
---------------- | ------------ | ------------ | ------------ | ------------ | ------------ | ------------ |
References
Burns, B. (2013), “Students’ perceptions of online courses in a graduate adolescence education program”, MERLOT Journal of Online Learning and Teaching, Vol. 9 No. 1, pp. 13-25.
Carr, S. (2000), “As distance education comes of age, the challenge is keeping the students”, Chronicle of Higher Education, Vol. 46 No. 23, pp. 39-41.
Dabbagh, N. (2007), “The online learner: characteristics and pedagogical implications”, Contemporary Issues in Technology and Teacher Education, Vol. 7 No. 3, pp. 217-226.
Elmendilia, S. and Saaidi, S. (2020), “Distance learning practices in Moroccan universities in the era of the coronavirus: the case of Mohammed V University in Rabat”, The Journal of Quality in Education, Vol. 10 No. 16, pp. 71-102.
Giret, J.F. (2020), “What satisfaction for distance and face-to-face students in French universities?”, Institute for Research in the Sociology and Economics of Education, Dijon Cedex, 10 July.
HCP (2020), “Survey on the impact of Coronavirus on the economic, social and psychological situation of the households: summary of main results”, available at: https://www.hcp.ma/Enquete-sur-l-impact-du-coronavirus-sur-la-situation-economique-sociale-et-psychologique-des-menages-Note-de-synthese_a2506.html (accessed 4 October 2021).
Lahchimi, M. (2015), “The reform of teacher training in Morocco”, International Journal of Education of Sèvres, Vol. 69, pp. 21-26.
Martins, O.B. (2011), “The continuing education of teachers and academic advisors in distance higher education. Pedagogical mediation and transformative practices”, Distances and Knowledge, Vol. 9 No. 2, pp. 257-268.
Naji, A. (2020), “The repercussions of Covid-19 on the field of education”, The Journal of Quality in Education, Vol. 10 No. 16, pp. 1-21.
Rechidi, N. and Bennani, H. (2020), “The pedagogical integration of ICT in the test of the crisis covid-19: What lessons to draw?”, International Journal of the Researcher, Vol. 1 No. 2, pp. 1-24.
Rivera, J.C., McAlister, M.K. and Rice, M.L. (2002), “A comparison of student outcomes & satisfaction between traditional & web-based course offerings”, Online Journal of Distance Learning Administration, Vol. 5 No. 3, pp. 1-11.
Sahin, I. and Shelley, M. (2008), “Considering students’ perceptions: the distance education student satisfaction model”, Educational Technology & Society, Vol. 11 No. 3, pp. 216-223.
Saykili, A. (2018), “Distance education: definitions, generations, key concepts and future directions”, International Journal of Contemporary Educational Research, Vol. 5 No. 1, pp. 2-17.
Sherry, L. (1995), “Issues in distance learning”, International Journal of Educational Telecommunications, Vol. 1 No. 4, pp. 337-365.
Summers, J.A., Hoffman, L. Marquis, J. Turnbull, A. Poston, D. and Nelson, L.L. (2005), “Measuring the quality of family-professional partnerships in special education services”, Exceptional children, Vol. 72 No. 1, pp. 65-81.
UNSDG (2020), Policy Brief: Education during COVID-19 and Beyond, United Nations Sustainable Development Group, New York, NY.
Xie, K. Debacker, T.K. and Ferguson, C. (2006), “Extending the traditional classroom through online discussion: the role of student motivation”, Journal of Educational Computing Research, Vol. 34 No. 1, pp. 67-89.
Zouiri, L., El Marhoum, A. and Ezzahid, E. (2020), “Distance learning in Morocco: perceptions of students during the Covid-19 confinement period from a national survey”, paper presented during the Study Day as part of the 50th anniversary of the Francophonie, Rabat, 19 May.
Acknowledgements
This paper forms part of a special section Teaching and Learning in Higher Education: Opportunities, Challenges, and Policy Implications, guest edited by Dr Stephanie Wing Lee.
Corresponding author
About the authors
Lahboub Zouiri is a teacher-researcher at the Faculty of Law, Economics and Social Sciences (FSJES), Agdal-Rabat and a member of the Laboratory of Applied Economics (LEA) accredited within the FSJES, Agdal. He obtained a PhD in Economic Sciences from CEMAFI University of Nice-Sophia Antipolis.
Fatima Ezzahra Kinani is an engineer in statistics and applied economy and a PhD candidate in the Laboratory of Applied Economics (LEA) within the Faculty of Law, Economics and Social Sciences, Agdal-Rabat. She works on immaterial capital and its link with economic growth.