Abstract
Purpose
In the current dynamic business environment, Internet of Things (IoT) is employed by a number of companies in the logistics industry to achieve intelligent sorting, network optimization, real-time tracking and simplifying last-mile service. Although logistics entities are trying to introduce IoT into their business areas, users' perception of this new technology is still limited. This paper aims to develop a research model for the factors influencing the user adoption of IoT technology in the logistics industry.
Design/methodology/approach
In this study, based on the major theories on the application of new technologies such as technology acceptance model (TAM), technology–organization–environment (TOE) and innovation diffusion theory (IDT), a new research model was established to identify factors affecting customers' behavioral intention (BI) to adopt IoT technology provided by logistics companies. In addition, the authors surveyed unspecified customers of Cainiao Logistics Network, which is in charge of the logistics operation of Alibaba Group, China's largest e-commerce company, and tested the causality between the latent variables presented in the model using the structural equation model (SEM).
Findings
This empirical study shows that the support system of a logistics company and users' innovative propensity significantly affect perceived ease of use (PEOU) and BI for logistics services to which IoT technology is applied. It also presents that users' perceived security and enjoyment significantly affect perceived usefulness (PU) and BI. In addition, it was possible to confirm that the causal structure between variables suggested by TAM that PEOU has a significant effect on PU and BI, and PU has a substantial effect on BI.
Practical implications
Logistics companies should expand and upgrade technical support systems so that customers can flexibly accept logistics services with IoT technology and make efforts to alleviate customers' concerns about personal information leakage. In addition, it is necessary to find customers with an inclusive attitude toward using new technologies, to induce them to become leading users of logistics devices with IoT technology and to find various ways to amplify their enjoyment. Through a strategic approach to these technical and individual factors, it will be possible to boost customers' intention to use IoT logistics services.
Originality/value
As far as the authors know, this paper is the first study to set significant factors that affect users' BI to use IoT technology-applied logistics services provided by logistics companies and empirically analyze the causal relationships between proposed latent variables.
Keywords
Citation
Zhang, X.Y. and Lee, S.Y. (2023), "A research on users' behavioral intention to adopt Internet of Things (IoT) technology in the logistics industry: the case of Cainiao Logistics Network", Journal of International Logistics and Trade, Vol. 21 No. 1, pp. 41-60. https://doi.org/10.1108/JILT-11-2022-0067
Publisher
:Emerald Publishing Limited
Copyright © 2023, Xin Yue Zhang and Sang Yoon Lee
License
Published in Journal of International Logistics and Trade. Published by Emerald Publishing Limited. This article is published under the Creative Commons Attribution (CC BY 4.0) licence. Anyone may reproduce, distribute, translate and create derivative works of this article (for both commercial and non-commercial purposes), subject to full attribution to the original publication and authors. The full terms of this licence may be seen at http://creativecommons.org/licences/by/4.0/legalcode
1. Introduction
With the coming of the information era and the development of artificial intelligence (AI), modern logistics operation through computers, information data communication, Internet of Things (IoT) and other technologies is the general trend. Under the premise of world economic integration, goods circulation and tracking have become critical issues worldwide. Using traditional technology to track and identify express parcels is inefficient and difficult to handle if problems arise. However, the development of the IoT has caused a revolution in the logistics industry. Besides providing new technology for modern logistics, it also improves the outdated logistics process and management model.
The IoT was first introduced in 1991 by Kevin Ashton. In 1999, MIT set up the Automatic Identification Center and advocated that everything can be connected through the network, which explained the IoT's basic meaning. The IoT is described as an extension of the Internet consisting of unique addressable equipment with sensing, networking and propulsion capabilities, facilitating the information exchange between things and humans, human and machine and machine and machine in heterogeneous environments (Patel et al., 2016).
In 2018, driven by AI technology and the development of global smart manufacturing, the application of IoT technology began to explode. The infinite potential of IoT is gradually disrupting the current business model by opening information dialogue between objects, creating more intelligent and diverse products and services, gathering the energy of big data and bringing new operational management and strategic challenges (Pribyl et al., 2019). In China, according to IiMedia Research, the IoT market in logistics exceeded $60 bn in 2018. By 2025, this value is projected to exceed $150 bn. Several IoT technologies are already in large-scale use, achieving intelligent positioning and navigation, device-to-device communication, visual perception and environmental awareness. Their disruptive innovation will significantly change people's lives. Meanwhile, it is widely believed in the industry that the IoT is closely related to logistics. IoT sensing technology mainly includes RFID (Radio frequency identification devices) technology, and the RFID warehouse logistics management system can realize the identification, management and tracking of goods (Liu et al., 2020). In addition, IoT technologies have contributed to the emergence of robots and drones that can solve the delivery problem for logistics companies. These technologies are connected to warehouse systems, thus accelerating the integration of intelligent logistics (Orji et al., 2020). In the current dynamic environment, IoT is needed to achieve intelligent sorting, network optimization, real-time tracking location and simplifying the last mile (Jiao, 2020). Although many logistics companies have used IoT technology, users' perception of this technology is still limited. More and more logistics companies hope IoT technology will bring industrial upgrading, reduce logistics costs and improve customer satisfaction.
The subject of this paper, Cainiao Network, was created by Alibaba Group in 2013. Cainiao provides integration and optimization services to many businesses, including e-commerce companies, warehouses and third-party logistics service providers. It is dedicated to developing a far-sighted logistics management system using IoT technologies to improve express speed, accuracy and safety. Under the co-creation and construction of the whole system, as of 2019, Cainiao has covered 250 cities in China, with more than 90,000 distribution routes and more than 40,000 terminal distribution stations. In June 2020, the Cainiao Network indicated that it should accelerate digital infrastructure construction to face the post-epidemic era. The logistics field continues to promote the digitalization process by using IoT technology. Its latest release includes new logistics computers, new generation of ultra-simple PDAs (Personal digital assistants), intelligent distribution machines and other new IoT products. Not only does it promote the optimization of logistics cost efficiency but it also brings personalized logistics experience to consumers.
The adoption of information technology has generated new models and approaches over time, and how users can better adopt information technology has become a meaningful focus of academic research. Enterprises must understand how users accept and propose corresponding measures to promote IoT technology. Therefore, this study intends to select the technology acceptance model (TAM) and integrate some related technical and individual characteristics factors derived from the technology–organization–environment (TOE) and innovation diffusion theory (IDT) model to construct a preliminary research model. Taking Cainiao's Logistics Network as the research sample, it attempts to develop a research model of the factors influencing user adoption of IoT technologies in the logistics industry and then suggests how to achieve intelligent upgrading, improve logistics services and optimize the efficiency of logistics enterprises. In the following, the paper uses pre-designed questionnaires to explore event factors, to search definitions and to record opinions. In this method, most questionnaires were sent by email or questionnaire platforms. Next, the structural equation model (SEM) method was employed to construct, evaluate and test the causality. It allows greater flexibility in measurement models and tests the relationship between variables by conducting path analysis. This paper utilized IBM SPSS 27.0 and Amos 24.0 to perform related tests and analyze the results.
The remainder of this paper is organized as follows. Section 2 contains a literature review. Section 3 contains the model construction theories, which provides a conceptual model of user acceptance behavior of IoT in the logistics industry and the related hypotheses. Section 4 consists of the empirical research, which uses statistical regression methods to conduct a path analysis and effect test. Section 5 presents the findings of this study and provides policy implications.
2. Literature review
2.1 Overview of the IoT in the logistics industry
With society's development, the requirements of the logistics industry are becoming higher and higher. Due to the emergence and development of AI, unmanned vehicles, big data and blockchain, the logistics industry is transforming into intelligent logistics (Wanganoo and Patil, 2020). Many researchers have explored the use of IoT in logistics companies. Chen et al. (2021) cites a series of applications of IoT technology, such as intelligent transportation systems, warehousing automation, unmanned cargo delivery and e-commerce applications, which improve the production and transportation of companies. De Vass et al. (2021) argues that the thematic analysis using NVivo finds that IoT ameliorates cargo transportation visibility, data collection, partner sharing and commercial intelligence. Poenicke et al. (2019) found in a study on the co-distribution model of logistics zones that the IoT can optimize the logistics delivery process, significantly improve the integration of enterprise and social logistics, and boost the level of supply chain management. Furthermore, IoT and large-scale data analysis platforms can improve the logistics industry's asset management, service distribution and transportation route planning capabilities. These lead to shorter delivery times and provide customers with real-time tracking of cargo delivery status and mobile delivery (Lin and Lin, 2019).
2.2 Research related to the theory of technology adoption behavior
Technology adoption is the decision of a company or a person to begin using a technology that is influenced by diverse factors, such as the technology itself, the external environment and the internal elements of the company. Theories of technology adoption at the individual level include the theory of reasoned action (TRA), the theory of planned behavior (TPB), the TAM and the IDT. Besides, the organizational level mainly includes TOE model.
Davis (1989) put forward the TAM. This model is more effective in explaining and predicting users' adoption behavior. Through a series of causal relationships such as belief, attitude, intention and behavior, the TAM can analyze why external variables, some more dependent on the specific context and vary from various studies, such as differences in user characteristics, other environmental and technological variables, can affect individuals' use of new information systems (Riyadh et al., 2009). As shown in Figure 1, in TAM theory, external factors influence the intention to use and actual behavior, in which perceived usefulness (PU) and perceived ease of use (PEOU) play a mediating role. However, behavioral adoption intention is influenced by an individual's attitude toward system use and perceived system utility (Chaveesuk et al., 2020). The TAM model maintains that a user's behavior in using new information technology is mainly motivated by user intention, which is simultaneously governed by PU and PEOU. If the PU and PEOU are high, the user's intention to use the information technology will also increase. Davis did further research and found that PEOU affects PU. If the user feels the information system is easy to use, their efficiency will increase.
In 1990, Tonatzky and Fleischer proposed the TOE model, in which they argued that when an organization adopts new technology, it should consider the technical, organizational and environmental factors. The model provides a research framework that allows researchers to determine the variables of each dimension according to the actual research question.
American communication theorist Everett M. Rogers (1983) developed IDT. In his view, this theory examines the predictable diffusion of innovations in society. The theory suggests that some people are more willing to embrace innovation than others under the same conditions because they are more open-minded and more likely to perceive the enjoyment nature of new technology. Chen (2013) cites two control variables from the IDT model, i.e. user innovation acceptance and user experience enjoyment, in investigating user adoption of mobile IoT devices about the hedonic benefits of user adoption.
Under this context, the TAM alone is insufficient to explain potential adopters' behavioral intentions. A TAM-based approach, combined with TOE and IDT, helps to develop integrated models and provides a basis for studying user adoption of up-and-coming technologies, such as IoT and cloud computing. Besides TAM, earlier studies have suggested that acceptance of IoT generally involves technological and behavioral aspects for personal use. Gangwar et al. (2014) advocates the integration of the TAM and the TOE to increase their explanatory power. While the influence of technology, organization and external variables are significant, the TAM's tests proved that PU and PEOU explain 40% of the users' intention to accept the technology (Legris et al., 2003). Chatterjee et al. (2021) employed the TAM-TOE model to explain employee intentions toward IoT in digital manufacturing and tested it using survey-based data collected from 340 employees in small, medium and large companies. The results show that complexity, compatibility and competitive advantage influence on PEOU or PU, further on users' adoption intentions. Karahoca et al. (2018) used TAM and IDT to build an integrated model, using a partial least squares model and identified the factors related to technology acceptance and diffusion of innovations, such as technological innovativeness, compatibility, image and perceived severity. These factors significantly influenced individuals' decisions to adopt IoT products in healthcare industry.
3. Model construction and research hypothesis
3.1 Model construction
This chapter adopts the TAM, TOE and IDT models, based on a review of theoretical literature, in conjunction with the practical application of IoT technology to develop a research model of user adoption of IoT in the logistics industry. First, the TAM model has an influencing factor of external variables. Since the IoT is an information technology used by users and primarily influenced by human-object interactions, this study focuses on the users' willingness to adopt rather than the enterprises. Therefore, external factors mainly consider the technology variables in the TOE model and do not fully consider the organizational and environmental influencing variables. Second, the proposed model takes into account the individual characteristics of users based on IDT. The primary motivation for users to seek operational experience is the pleasure it brings them, which influences their adoption intentions (Alalwan et al., 2017). By referring to technical influence variables from TOE and individual innovation differences from IDT theory, research hypotheses are formulated regarding technical factors, personal characteristic dimensions and PU and PEOU in the TAM, trying to build a theoretical model of influence factors.
Regarding technical factors, firstly, it is considered that users' IoT access is based on having convenient facilities to support them and comprehensive customer service. In addition, IoT as an information technology, privacy disclosure and property loss risks need to be considered. In terms of individual characteristics, according to the IDT theory, adopters with innovation characteristics are more likely to be exposed to new technologies and take the initiative to learn about them. Second, as a novelty, the IoT brings many new changes. Users will be entertained by using it, experiencing pleasure, enjoyment and other psychological feelings that will influence their intention to adopt IoT technology (Park et al., 2014).
In conclusion, this paper chooses the support system, perceived security as the technical factors and individual innovation and perceived enjoyment as the individual characteristic factors, combined with PU, PEOU and behavioral intention (BI) in the classical TAM model. Since IoT is a newly introduced technology, there is a lack of sufficient data on whether users actually use it. For this reason, the current paper only examines whether users have the corresponding BIs. These ultimately act on the impact of the user's attitude to IoT.
3.2 Research hypothesis
The hypotheses of this study were established according to the causal structure among the latent variables described below.
3.2.1 Support system
The provision of IoT-related support systems for users will create an easy-to-use environment. As IoT applications in the logistics industry have a higher threshold for device configuration, users also need to have a better ability and more complete conditions of use, so the support system factor is essential. Venkatesh and Davis (2000) define a support system in a new way, the level of support from others, the organization and other basic resources to use technology. The variables mainly include the IoT infrastructure that is considered to be provided by logistics companies for users, such as the number and popularity of unmanned terminal delivery vehicles and intelligent express cabinets and whether remote areas can be delivered using drones. Other support systems include customer service experts who can answer questions and solve users' problems (Yang, 2011). In addition, the accessibility, convenience and interactivity of IoT-related facilities affect user acceptance and satisfaction with IoT (Ding, 2020). Excellent support systems for IoT in the logistics industry can improve the logistics system by improving its information integration degree, and the ubiquitous IoT devices will also make it less complicated for users to use these new technologies (Chen et al., 2017).
Including the support system item in the TAM model is more relevant to the situation. Cainiao Logistics needs to invest in infrastructure to make more users enjoy the benefits of IoT technology. When customers have problems, it is essential to have professional staff to solve them. A better support system will enable more users to accept IoT technology. Hence, the hypotheses below are proposed.
Support system positively impacts users' PEOU.
Support system positively impacts users' BI to adopt IoT technologies in logistics.
3.2.2 Perceived security
The IoT collects a lot of information by making things work together to provide better services to meet user preferences. With the current misuse of personal information, protecting the security of the collected data is an issue that needs to be solved (Chen et al., 2014). Although access to the data is centralized and controlled, the cloud-supported IoT is susceptible to security and privacy issues (Arpaci et al., 2015). The emergence of security issues will directly reduce users' trust in the service or the enterprise. Muangmee et al. (2021) found that customers were very concerned about the security of the new technology when investigating the factors of BI to use food delivery applications in Thailand during the COVID-19 pandemic. In general, protecting customer data security will be one of the top long-term priorities for developing new technologies. Cho et al. (2016) believed perceived insecurity tends to be the main problem preventing consumers from using IoT technologies because users are worried about their data being leaked, especially their credit card data. Logistics companies can improve their reliability and user satisfaction by providing security services, and it also will increase users' PU (Al-Qaseemi et al., 2017). If the application provided by the enterprise has highly perceived security to protect users' information, users will trust the IoT application more and adopt it (Karahoca et al., 2018). The following hypotheses are consequently put forward.
Perceived security positively impacts users' PU.
Perceived security positively impacts users' BI to adopt IoT technologies in logistics.
3.2.3 Individual innovation
Rogers (2002) believed that the timing and motivation to adopt new technologies vary with the willingness of individual users to embrace innovation. Innovation can be self-measured in attitudes and personal characteristics (Strobl et al., 2020). This personality trait is something that we all possess, just to different degrees. In the research on innovation awareness, some people think it directly impacts user adoption, while others believe it plays a moderating role. If users have strong individual innovation abilities, they will try to adopt the new technology even if they encounter difficulties. Thus, they will think of the IoT technology as easy to use and intend to use this new technology (Yi et al., 2006).
Individual innovation positively impacts users' PEOU.
Individual innovation positively impacts the users' BI to adopt IoT technologies in logistics.
3.2.4 Perceived enjoyment
IDT and related theories indicate that enjoyment motivation is essential in technology use scenarios (Lee, 2019). Perceived enjoyment is the degree of pleasure in using a system without considering performance outcomes (Venkatesh and Bala, 2008). The positive impact of perceived enjoyment on user adoption behavior of various information technologies has been confirmed (Mavlanova et al., 2012). Thong et al. (2006) statistically proved the significant influence of fun on customer intention to adopt IoT applications, and enjoyable information technology will significantly improve users' PU. In the current study, perceived enjoyment refers to the user's perception that using the IoT in the Cainiao Logistics Network will give them a pleasurable experience besides usefulness. At the same time, IoT applications have a link between things and people, so users will also find it fun and valuable. This experience creates a relationship between perceived enjoyment and the user's BI. Therefore, the following hypotheses are proposed in this study.
Perceived enjoyment positively impacts users' PU.
Perceived enjoyment positively impacts users' BI to adopt IoT technologies in logistics.
3.2.5 Perceived ease of use
PEOU is the degree to which a person's perception that adopting a specific system or product will be easy to use (Davis, 1989). The relationship between PEOU and PU was interpreted as follows. If people use any technology product or system as simple and easy to use, they will perceive it as more practical (Venkatesh and Davis, 2000). In the meantime, it will have a positive impact on PU. Rahman et al.(2019) argues that although the relationship between PU and BI is more significant, PEOU and PU, considered a whole, have a more considerable weight in predicting users' willingness to develop IoT. Based on the TAM complete model results, it concluded that PEOU positively impacts the users' BI to adopt IoT products (Waheed and Jam, 2010). Therefore, the following hypotheses are proposed in this study.
Users' PEOU positively influences users' PU.
Users' PEOU positively affects users' BI to adopt IoT technologies in logistics.
3.2.6 Perceived usefulness
Researchers often refer to PU as the extent to which users believe that using specific information technology will improve their performance. The PU of TAM is similar to performance expectations. Patil (2016) argues that PU significantly impacts employees' attitudes toward IoT adoption. Silva-Treviño (2021) finds that the repeated use of information technology is mainly affected by users' PU, and improving PU is very important for enhancing user stickiness. PU is the main factor in BI to use, which motivates users to adopt more efficient and practical technologies. Therefore, the greater the PU of an IoT product in the logistics industry, the more people are motivated to use it.
PU positively impacts users' BI to adopt IoT technologies in logistics.
3.2.7 Behavioral intention
In the user adoption model, BI refers to users' positive or negative options when approaching new technology (Ajzen, 1985). BI connects the user's attitude to use to actual use. All internal and external factors ultimately act on the user's intention, which eventually translates into the user's actual behavior toward the IoT technology.
Figure 2 shows the empirical research model including the research hypothesis presented above.
4. Research process
4.1 Questionnaire design and data collection
The present research takes a multi-perspective on groups related to Cainiao logistics, such as employees of Cainiao courier station, couriers, students in the logistics industry and ordinary users, to process a questionnaire survey. The first part is the preface, divided into questionnaire intent and application scenarios. The second part is the respondents' information, specifically gender, age and whether they have been in touch with IoT applications. The data from this part will be used for descriptive statistics to lay a foundation for the accuracy of follow-up research. The third part includes 26 specific questions, using a five-point Likert scale to measure the influencing factors and user BI for IoT in the Cainiao Logistics Network. The final items and their sources are listed in Appendix.
After forming a formal questionnaire, the official research started by distributing the questionnaire link and scanning the quick response (QR) code for the online filling to record the opinions and suggestions of the research respondents. Finally, 317 questionnaires were collected, excluding 54 invalid questionnaires and received 263 valid questionnaires. The effective rate of questionnaires was 83%. The results of the descriptive analysis of the 263 valid data by SPSS 27.0 are summarized in Table 1.
4.2 Reliability and validity analysis
The reliability of the research design scales is usually measured by analyzing Cronbach's α coefficients, with Hatcher and O'Rourke (2013) suggesting that 0.6 is the lowest acceptable level. The questionnaire's Cronbach's α coefficients and seven variables were measured and analyzed using SPSS 27.0. As shown in Table 2, the outcome presents the overall Cronbach's α coefficient is 0.919, and the coefficients of each subscale are above 0.8. These results indicate that the overall questionnaire design is satisfactory.
The validity analysis is based on the KMO (Kaiser-Meyer-Olkin) and Bartlett's sphericity test to show how well the measure fits the desired target. The overall KMO of the questionnaire was 0.890, and Bartlett's sphericity test was less than 0.05, indicating high correlation and good structural validity. Next, the construct validity of the model scale was checked by confirmatory factor analysis (CFA) as presented in Figure 3. This test is intended to verify whether the data fit the hypothetical measurement model.
The maximum likelihood estimation technique was carried out, and the specific indicators are as follows. The first is the chi-square test, which is tested by the weight of χ2/df. The smaller the value, the better the fit. The second is the root mean square index of error approximation (RMSEA). The sample size influences it less, but it is easy to detect the model error. The closer the value of RMSEA is to 0, the better the model fits. Then comes the standardized residual root mean square (SRMR). Marsh (2004) state that the SRMR is one of the indicators that directly assesses the residuals, where the value ranges between 0 and 1. When the value is less than 0.08, the model fit is ideal. Finally, the incremental fit index (IFI), the comparative fit index (CFI) and the Tucker–Lewis index (TLI) are presented. Among them, CFI means the extent of the discrepancy between the independent and hypothetical models. The closer the values of these indicators are to 1, the better the hypothesis of model fitness. As shown in Table 3, the results of CFA satisfy all the suggested goodness of fit criterion.
Through CFA analysis of the overall model, standardized factor loadings, construct reliability (CR) and average variance extracted (AVE) can be obtained. The standardized factor loadings should be greater than 0.5 and less than 0.95, and the CR values represent the internal consistency of the constructs, which are usually more than 0.7. The measures of each variable and their validation results are shown in Table 4.
Table 4 shows that the factor loadings of seven variables in the model, including support system, perceived security, individual innovation, perceived enjoyment, PEOU, PU and BI, are all in the range of 0.701–0.865 (>0.5). The CR is in the field of 0.825–0.894 (>0.6), and the AVE ranged from 0.541 to 0.709 (>0.5), which met the criteria of Hair et al. (2012) and Fornell and Larcker (1981), so all seven constructs had convergent validity.
Discriminant validity means the degree of correlation between items of different variables, measured by the Fornell–Larcker criterion in this paper. The specific results are as follows. According to the Fornell-Larcker criterion, the correlation coefficient between latent variables is lower than the square root of AVE, which indicates good discriminant validity. Table 5 shows that the square root of AVE for each latent variable is greater than the correlation coefficient between it and any other variable, indicating that the measurement model has strong discriminant validity.
4.3 Hypothesis testing
It can be seen from the previous analysis that the data reliability, validity and correlated indicators between the samples all meet the research standards. The goodness-of-fit metric is a way of determining whether the data fit the model and judging whether the model fits the data when examining the following five indicators. In this section, Amos 24.0 was used for path analysis and model determination. The larger the path coefficient, the larger the relationship between the variables and the stronger the significance. From Table 6, χ2/df is 1.688, RMSEA is 0.051, SRMR is 0.075, TLI is 0.944, CFI is 0.951 and IFI is 0.952. The fit indicators all meet the judging criteria, so the model fits well, and the data collected by this study meet the requirements.
The next step is path analysis, which aims to test a hypothesized cause–effect model's reliability and accuracy and gauge the causality's intensity between variables. First, we predicted whether there is a link between multiple variables in the model and then investigated a causal relationship between the variables and determine which are the independent variables and the dependent variables. If one variable affects another, judge whether it exerts a direct or indirect effect through mediating variables. The final result of the proposed SEM is shown in Table 7 and Figure 4.
Six of the 11 proposed hypotheses were significant for the dependent variable at the 95% level, and the other five were substantial for the dependent variable at the 99% level. Due to the high reliability of the selection of influencing factors, there was no need to remove variables and revise the model. This research model may provide some guidance for the behavior of IoT users in the logistics industry, and it is feasible to obtain users' perceptions of IoT through questionnaires. The intermediate effect is to verify whether the independent variable X influences the dependent variable Y through a variable. AMOS 24.0 was used to test the mediating effects in this study. And the deviation-corrected percentile bootstrap method was used to assess the specific mediating effects' importance. We set the bootstrap times to 5,000 and the confidence interval to 95%.
From Table 8, a significant indirect effects of the support system and individual innovation on the PU were noted (p < 0.05), with an indirect effect size of 0.062 and 0.223 respectively. In addition, the indirect effect of perceived security on BI was noted, with an indirect effect size of 0.028. Among the seven indirect paths in the figure, only perceived enjoyment did not pass the significance test of a p-value equal to 0.05 for BI, and the confidence interval contained 0.
5. Conclusions
5.1 Results and implications
The empirical test confirms that support system is a significant factor for the BI of IoT services in the logistics industry for users. If the popularity of IoT devices is high, the users will find them beneficial and easy to use, and then the BI will increase. The mediating effect of the support system positively impacts PU, which shows that the support system factor also affects the user's judgment of whether IoT in logistics is useful in their lives. From the user's perspective, perceived security has a noticeable impact on PU and BI. Security has always been a top priority in all industries, especially for new things. Users may refuse to use an IoT application if they perceive it as less secure, leaking their privacy or creating financial risks. This study presents that innovative personnel would be more prone to use IoT devices. They may easily have confidence in IoT technology and overcome the barriers to using it, reducing their technical efforts and cost perceptions to a certain extent. Individual innovation has a significant mediating effect on PU, indicating that people with high innovation quality also generally influence PU. IoT services with high experience and fun are more likely to be preferred and used by users. The users feel enjoyment when experiencing IoT products, and they will know that the products can help them in their work and life, so they will be enthusiastic about using the technology. In addition, PEOU affects PU and thus influences BI, and PU also directly affects BI. The association between these two variables, which are the core of the TAM model, has been verified on several occasions. This study reconfirms the reliability of this conclusion. It shows that the IoT devices or services of Cainiao logistics should balance PEOU and PU, which is the most basic requirement for designing user-oriented new technology products.
This paper takes the IoT users of Cainiao logistics as the primary research subject, and by analyzing the results, the following insights are proposed. First, according to the empirical study, a support system can improve users' PEOU and BI. Cainiao logistics has invested enormous human and material resources to develop IoT logistics services, and most of China's cities are currently equipped with Cainiao's IoT devices. But in some towns distributed in the mountainous areas of northwest China, the express delivery method is more outdated, and the penetration of IoT devices is lower. Therefore, accelerating the development of IoT infrastructure in poor areas and the replacement of IoT equipment are far-reaching measures. Paying attention to the improvement of relevant knowledge of employees and enhancing user-friendly use conditions are critical paths for Cainiao to develop intelligent logistics. Second, perceived security is a critical factor in the use of logistics IoT products. The indicators include information control, privacy protection, data confidentiality, integrity and availability. The perceived security of a product has a crucial impact on user adoption; consequently, improving the product's security is one of the top priorities of logistics enterprises in developing IoT products. The logistics company should establish perfect usage norms, optimize the product security design process from the source, strengthen the construction of enterprise credit mechanisms, prevent user privacy leakage and improve user trust and perceived security.
Third, individual innovation of users can influence their intention to use IoT in the logistics industry. Cainiao logistics should identify such innovative talents and select them as the first users of IoT applications to take the lead and influence others. Fourth, the current empirical study results indicate that perceived enjoyment positively influences users' PU and BI, which implies that logistics companies should amplify the customer's pleasant experience of various logistics services to which IoT technology is applied.
Last but not least, PEOU positively influences users' PU of IoT products in the logistics industry. Strengthening the operability and visualization of IoT services or equipment will be an important way to succeed for Cainiao logistics. In addition, simplifying the system operation process, adding face recognition and other functions to optimize the operation steps can enhance users' intention to use.
5.2 Limitation
There are three limitations to this study. First, concerning the research samples, the descriptive statistical analysis of the data collected from the questionnaire shows that the proportion of respondents is relatively high among the young group, mainly students. As the connotations of the application of IoT technology are more complex, it should ask for more industry groups' opinions. Second, since IoT is a recently emerged technology and has not been fully adopted by users, it was hard to obtain accurate data through government or industry websites, and the validity of the data obtained from the questionnaire needs to be improved. Finally, the context of this paper is the application of IoT technology in the logistics industry's overall context, the refinement of the research situation is not sufficiently detailed and the selection of influencing factors may not be comprehensive enough.
Figures
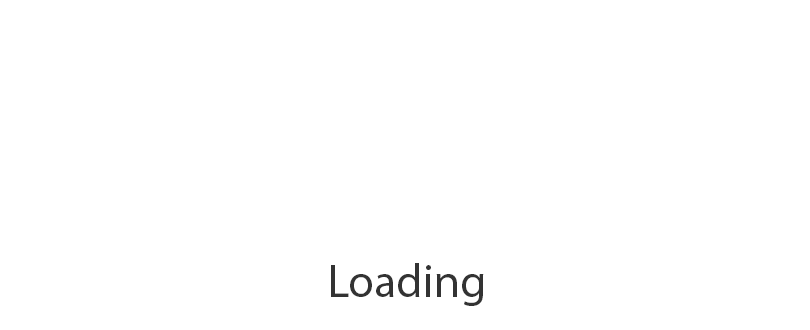
Figure 1
TAM model (Venkatesh and Davis, 2000)
Questionnaire descriptive statistics
Frequency | % | ||
---|---|---|---|
Gender | Male | 144 | 45.4 |
Female | 173 | 54.6 | |
Total | 317 | 100 | |
Age | 0–15 | 3 | 0.9 |
15–25 | 100 | 32.5 | |
25–35 | 75 | 23.7 | |
35–45 | 55 | 17.4 | |
45–55 | 63 | 19.9 | |
More than 55 | 21 | 6.6 | |
Total | 317 | 100 | |
Education | Primary school | 2 | 0.6 |
Junior school | 25 | 7.9 | |
High school | 38 | 12.0 | |
Bachelor degree | 148 | 46.7 | |
Postgraduate and above | 63 | 19.9 | |
Else | 41 | 12.9 | |
Total | 317 | 100 | |
User experience | Yes | 267 | 84 |
No | 50 | 16 | |
Total | 317 | 100 |
Questionnaire reliability
Dimension | Cronbach's alpha | Number of terms |
---|---|---|
Support system | 0.805 | 4 |
Perceived security | 0.887 | 4 |
Individual innovation | 0.869 | 4 |
Perceived enjoyment | 0.858 | 3 |
Perceived ease of use | 0.864 | 4 |
Perceived usefulness | 0.866 | 3 |
Behavioral intention | 0.875 | 4 |
Goodness of fit indicator
Indicator | χ2/df | RMSEA | SRMR | TLI | CFI | IFI |
---|---|---|---|---|---|---|
Criterion | <3.00 | <0.08 | <0.08 | >0.9 | >0.9 | >0.9 |
CFA | 1.512 | 0.044 | 0.0495 | 0.957 | 0.963 | 0.963 |
Assessment of reliability for model construct
Dimension | Item | Factor loading | T-value | CR | AVE |
---|---|---|---|---|---|
Support system | SS1 | 0.736 | 0.857 | 0.601 | |
SS2 | 0.704 | 7.830 | |||
SS3 | 0.815 | 9.899 | |||
SS4 | 0.837 | 9.953 | |||
Perceived security | PS1 | 0.827 | 0.894 | 0.677 | |
PS2 | 0.846 | 16.404 | |||
PS3 | 0.799 | 14.867 | |||
PS4 | 0.819 | 14.392 | |||
Individual innovation | II1 | 0.718 | 0.877 | 0.641 | |
II2 | 0.836 | 12.819 | |||
II3 | 0.828 | 12.900 | |||
II4 | 0.814 | 12.099 | |||
Perceived enjoyment | PE1 | 0.841 | 0.880 | 0.709 | |
PE2 | 0.865 | 15.547 | |||
PE3 | 0.820 | 12.576 | |||
Perceived ease of use | PEOU1 | 0.705 | 0.825 | 0.541 | |
PEOU2 | 0.733 | 15.593 | |||
PEOU3 | 0.743 | 13.276 | |||
PEOU4 | 0.760 | 11.714 | |||
Perceived usefulness | PU1 | 0.787 | 0.845 | 0.646 | |
PU2 | 0.861 | 14.548 | |||
PU3 | 0.760 | 13.565 | |||
Behavioral intention | BI1 | 0.701 | 0.827 | 0.545 | |
BI2 | 0.799 | 14.863 | |||
BI3 | 0.701 | 12.552 | |||
BI4 | 0.747 | 13.058 |
AVE square root and correlation matrix
Support System | Perceived Security | Individual innovation | Perceived enjoyment | Perceived ease of use | Perceived usefulness | Behavioral intention | ||
---|---|---|---|---|---|---|---|---|
Support System | 0.775 | Support System | ||||||
Perceived Security | 0.286** | 0.822 | Perceived Security | |||||
Individual innovation | 0.207** | 0.212** | 0.801 | Individual innovation | ||||
Perceived enjoyment | 0.139* | 0.220** | 0.357** | 0.842 | Perceived enjoyment | |||
Perceived ease of use | 0.246** | 0.440** | 0.472** | 0.365** | 0.736 | Perceived ease of use | ||
Perceived usefulness | 0.283** | 0.429** | 0.275** | 0.343** | 0.523** | 0.804 | Perceived usefulness | |
Behavioral intention | 0.323** | 0.451** | 0.477** | 0.393** | 0.611** | 0.508** | 0.738 | Behavioral intention |
Note(s): *p < 0.1, **p < 0.05 and ***p < 0.01
Fit indices for structural model
Indicator | χ2/df | RMSEA | SRMR | TLI | CFI | IFI |
---|---|---|---|---|---|---|
Criterion | <3.00 | <0.08 | <0.08 | >0.9 | >0.9 | >0.9 |
CFA | 1.688 | 0.051 | 0.075 | 0.944 | 0.951 | 0.952 |
Path analysis
Path | Standardized coefficients | Unstandardized coefficients | SE | CR | P |
---|---|---|---|---|---|
PEOU ← Support system | 0.142 | 0.154 | 0.069 | 2.218 | ** |
PEOU ← Individual innovation | 0.530 | 0.611 | 0.082 | 7.442 | *** |
PU ← Security | 0.261 | 0.316 | 0.077 | 4.084 | *** |
PU ← Perceived enjoyment | 0.152 | 0.131 | 0.055 | 2.374 | ** |
PU ← PEOU | 0.439 | 0.383 | 0.059 | 6.501 | *** |
BI ← Support system | 0.142 | 0.140 | 0.056 | 2.487 | ** |
BI ← Security | 0.127 | 0.159 | 0.075 | 2.131 | ** |
BI ← Individual innovation | 0.180 | 0.187 | 0.075 | 2.483 | ** |
BI ← Perceived enjoyment | 0.133 | 0.119 | 0.054 | 2.218 | ** |
BI ← PEOU | 0.336 | 0.303 | 0.070 | 4.328 | *** |
BI ← PU | 0.181 | 0.188 | 0.073 | 2.585 | *** |
Note(s): *p < 0.1, **p < 0.05 and ***p < 0.01
Mediation test
Indirect path | Mediating variable | Indirect effect | SE | Lower limit | Upper limit | P-value | Results |
---|---|---|---|---|---|---|---|
Support system → PU | PEOU | 0.062 | 0.039 | 0.001 | 0.157 | 0.046 | Supported |
Individual innovation → PU | PEOU | 0.233 | 0.049 | 0.144 | 0.361 | 0.000 | Supported |
Perceived security → BI | PU | 0.028 | 0.036 | 0.006 | 0.152 | 0.032 | Supported |
Perceived enjoyment → BI | PU | 0.023 | 0.021 | 0.000 | 0.089 | 0.056 | Not supported |
Individual innovation → BI | PEOU, PU | 0.220 | 0.056 | 0.126 | 0.352 | 0.000 | Supported |
Support system → BI | PEOU, PU | 0.059 | 0.037 | 0.001 | 0.147 | 0.047 | Supported |
PEOU → BI | PU | 0.080 | 0.043 | 0.004 | 0.174 | 0.042 | Supported |
Appendix Measurement items and their sources
Support system (adapted from Ajzen (1991), Al-Khaldi and Olusegun Wallace (1999), Venkatesh and Davis (2000), Chen et al. (2020) and Routray et al. (2021))
I can access and learn the approaches to using Cainiao's IoT devices and services.
In my city, I often see Cainiao's IoT facilities (intelligent delivery cabinets, delivery robots, etc.).
When I have difficulties using Cainiao's IoT applications, I can find customer service staff to guide me.
I think using Cainiao's IoT applications is easy if I have the necessary prerequisites.
Perceived security (adapted from Arpaci et al. (2015), Al-Sharafi et al. (2016), Conoscenti et al. (2016) and Demir et al. (2019))
I think my personal information will not be leaked when I use Cainiao's IoT application.
I think it's safe to use Cainiao's IoT applications because it uses the invisible express sheet and the information management system backed by Alipay.
I believe the IoT applications and devices provided by Cainiao are safe and reliable.
I think the payment process related to Cainiao IoT is risk-free and can guarantee users' money.
Individual innovation (adapted from Venkatesh and Davis (2000), Straub (2009), Karahoca et al. (2018) and Strobl et al. (2020))
I like to try new things, especially new technologies.
I am ahead of everyone else when it comes to trying new technology.
When I try new technologies, I rarely feel hesitant and doubtful.
I tend to try new technologies by all means, even spending some money and time.
Perceived enjoyment (adapted from Mavlanova et al. (2012) , Koenig Lewis (2015), Al-Gahtani (2016) and Alalwan et al. (2017))
I am satisfied with the overall service of Cainiao's IoT applications.
I feel an intense enjoyment when using Cainiao's IoT devices.
I think unmanned delivery will be more interesting than traditional manual delivery.
Perceived usefulness (adapted from Venkatesh and Davis (1996), Lee (2003), Haans et al. (2016) and Alalwan et al. (2017))
I think using Cainiao's IoT applications can make my life more convenient.
I think using Cainiao's IoT services can improve logistics efficiency.
I think Cainiao Logistics' IoT application makes receiving and sending express goods easier.
Perceived ease of use (adapted from Venkatesh and Davis (1996), Karahanna and Straub (1999), Gounaris and Koritos (2008) and Al-Sharafi et al., 2016)
I think Cainiao's IoT devices or APPs are convenient, and the operation process is straightforward.
I think learning how to operate Cainiao's IoT applications takes little time.
Getting the information I wanted through Cainiao's IoT application is easy.
It is easy to use Cainiao's IoT devices to complete the relevant logistics service.
Behavioral intention (adapted from Venkatesh and Davis (1996), Bozionelos (1996), Lynott and McCandless (2000), Venkatesh et al. (2003) and Messerschmidt and Hinz (2013))
I am willing to learn how to use Cainiao's IoT technologies.
I will use existing Cainiao's IoT technology applications and continue to use its innovative ones.
I will recommend the IoT application of Cainiao logistics to other people and friends.
As my understanding of IoT has deepened, my willingness to use the Cainiao Logistics IoT application has increased.
References
Ajzen, I. (1985), From Intentions to Actions: A Theory of Planned Behavior. Action Control, Springer, Berlin, Heidelberg, pp. 11-39.
Ajzen, I. (1991), “The theory of planned behavior”, Organizational Behavior and Human Decision Processes, Vol. 50 No. 2, pp. 179-211.
Alalwan, A.A., Dwivedi, Y.K. and Rana, N.P. (2017), “Factors influencing adoption of mobile banking by Jordanian bank customers: extending UTAUT2 with trust”, International Journal of Information Management, Vol. 37 No. 3, pp. 99-110.
Al-Gahtani, S.S. (2016), “Empirical investigation of e-learning acceptance and assimilation: a structural equation model”, Applied Computing and Informatics, Vol. 12 No. 1, pp. 27-50.
Al-Khaldi, M.A. and Wallace, R.O. (1999), “The influence of attitudes on personal computer utilization among knowledge workers: the case of Saudi Arabia”, Information and Management, Vol. 36 No. 4, pp. 185-204.
Al-Qaseemi, S.A., Almulhim, H.A., Almulhim, M.F. and Chaudhry, S.R. (2017), “IoT architecture challenges and issues: lack of standardization”, FTC 2016 - Proceedings of Future Technologies Conference, pp. 731-738 December.
Al-Sharafi, M. A., Ruzaini, A. A, Abu-Shanab, E. and Elayah, N. (2016), “The effect of security and privacy perceptions on customers’ trust to accept internet banking services: an extension of TAM”, Journal of Engineering and Applied Sciences, Vol. 11 No. 3, pp. 545-552.
Arpaci, I., Kilicer, K. and Bardakci, S. (2015), “Effects of security and privacy concerns on educational use of cloud services”, Computers in Human Behavior, Vol. 45, pp. 93-98.
Bozionelos, N. (1996), “Psychology of computer use: XXXIX. Prevalence of computer anxiety in British managers and professionals”, Psychological Reports, Vol. 78 No. 3, pp. 995-1002.
Chaveesuk, S., Chaiyasoonthorn, W. and Khalid, B. (2020), “Understanding the model of user adoption and acceptance of technology by Thai farmers”, Proceedings of the 2020 2nd International Conference on Management Science and Industrial Engineering.
Chatterjee, S., Rana, N.P., Dwivedi, Y.K. and Baabdullah, A.M. (2021), “Understanding AI adoption in manufacturing and production firms using an integrated TAM-TOE model”, Technological Forecasting and Social Change, Vol. 170, 120880.
Chen, L. (2013), Research on the Influence of Perceived Value on Mobile Shopping Behavior Intention. Master Thesis, South China University of Technology, Guangzhou.
Chen, S., Xu, H., Liu, D., Hu, B. and Wang, H. (2014), “A vision of IoT: applications, challenges, and opportunities with China perspective”, IEEE Internet of Things Journal, Vol. 1 No. 4, pp. 349-359.
Chen, S., Zhang, T. and Shi, W. (2017), “Fog computing”, IEEE Internet Computing, Vol. 21 No. 2, pp. 4-6.
Chen, J.H., Ha, N.T.T., Tai, H.W. and Chang, C.A. (2020), “The willingness to adopt the Internet of Things (IoT) conception in Taiwan's construction industry”, Journal of Civil Engineering and Management, Vol. 26 No. 6, pp. 534-550.
Chen, J., Wu, H., Zhou, X., Wu, M., Zhao, C. and Xu, S. (2021), “Optimization of Internet of Things E-commerce logistics cloud service platform based on mobile communication”, Complexity, Vol. 10, pp. 1-11.
Cho, C., Yoon, B., Coh, B.Y. and Lee, S. (2016), “An empirical analysis on purposes, drivers and activities of technology opportunity discovery: the case of Korean SMEs in the manufacturing sector”, R and D Management, Vol. 46 No. 1, pp. 13-35.
Conoscenti, M., Vetro, A. and De Martin, J.C. (2016), “Blockchain for the internet of things: a systematic literature review”, Proceedings of IEEE/ACS International Conference on Computer Systems and Applications, AICCSA, Vol. 0, pp. 1-6.
Davis, F.D. (1989), “Perceived usefulness, perceived ease of use, and user acceptance of information technology”, MIS Quarterly: Management Information Systems, Vol. 13 No. 3, pp. 319-339.
Demir, M., Turetken, O. and Ferwom, A. (2019), “Blockchain and IoT for delivery assurance on supply chain (BIDAS)”, Proceedings - 2019 IEEE International Conference on Big Data, pp. 5213-5222.
De Vass, T., Shee, H. and Miah, S.J. (2021), “IoT in supply chain management: opportunities and challenges for businesses in early industry 4.0 context”, Operations and Supply Chain Management, Vol. 14 No. 2, pp. 148-161.
Ding, L. (2020), “A survey of undergraduate English learners’ satisfaction with the flipped classroom and its influencing factors”, Journal of Nanjing University of Posts and Telecommunications, Vol. 20 No. 6, pp. 41-43.
Fornell, C. and Larcker, D.F. (1981), “Evaluating structural equation models with unobservable variables and measurement error”, Journal of Marketing Research, Vol. 18 No. 1, pp. 39-50.
Gangwar, H., Date, H. and Raoot, A.D. (2014), “Review on IT adoption: insights from recent technologies”, Journal of Enterprise Information Management, Vol. 27 No. 4, pp. 488-502.
Gounaris, S. and Koritos, C. (2008), “Investigating the drivers of internet banking adoption decision: a comparison of three alternative frameworks”, International Journal of Bank Marketing, Vol. 26 No. 5, pp. 282-304.
Haans, R.F., Pieters, C. and Zi-Lin, H. (2016), “Thinking about U: theorizing and testing U- and inverted U-shaped relationships in strategy research”, Strategic Management Journal, Vol. 37, pp. 1177-1195.
Hair, J.F., Sarstedt, M., Pieper, T.M. and Ringle, C.M. (2012), “The use of partial least squares structural equation modeling in strategic management research: a review of past practices and recommendations for future applications”, Long Range Planning, Vol. 45 Nos 5-6, pp. 320-340.
Hatcher, L. and O'Rourke, N. (2013), “A step-by-step approach to using SAS system for factor analysis and structural equation modeling”, International Statistical Review, Vol. 83 No. 2, pp. 325-326.
Jiao, Z. (2020), Development of Technology-Driven Intelligent Logistics in China. Contemporary Logistics in China, Springer, Singapore, pp. 205-225.
Karahanna, E. and Straub, D.W. (1999), “The psychological origins of perceived usefulness and ease-of-use”, Information and Management, Vol. 35 No. 4, pp. 237-250.
Karahoca, A., Karahoca, D. and Aksöz, M. (2018), “Examining intention to adopt internet of things in healthcare technology products”, Kybernetes, Vol. 47 No. 4, pp. 742-770.
Koenig Lewis, N., Marquet, M., Palmer, A. and Zhao, A.L. (2015), “Enjoyment and social influence: predicting mobile payment adoption”, The Service Industries Journal, Vol. 35 No. 10, pp. 537-554.
Lee, H.L. (2003), “Aligning supply chain strategies with product uncertainties”, IEEE Engineering Management Review, Vol. 31 No. 2, pp. 26-34.
Lee, S. (2019), “An analysis on the changes of logistics industry using internet of things”, Journal of Advanced Information Technology and Convergence, Vol. 9 No. 1, pp. 57-66.
Legris, P., Ingham, J. and Collerette, P. (2003), “Why do people use information technology? A critical review of the technology acceptance model”, Information and Management, Vol. 40 No. 3, pp. 191-204.
Lin, C. and Lin, M. (2019), “Application of big data in a multicategory product-service system for global logistics support”, IEEE Engineering Management Review, Vol. 47 No. 4, pp. 108-118.
Liu, L., Cheng, Z., Yang, W., Yang, L., Wang, L., Huang, C. and Pu, X. (2020), “Investigation and research on user satisfaction of Cainiao courier station of sichuan university of arts and sciences”, Voice of the Publisher, Vol. 6 No. 3, pp. 150-159.
Lynott, P.P. and McCandless, N.J. (2000), “The impact of age vs. life experience on the gender role attitudes of women in different cohorts”, Journal of Women and Aging, Vol. 12 Nos 1-2, pp. 5-21.
Marsh, H.W. (2004), “In search of golden rules: comment on hypothesis-testing approaches to setting cutoff values for fit indexes and dangers in overgeneralizing Hu and Bentler’s (1999) findings”, Structural Equation Modeling, Vol. 11 No. 3, pp. 320-341.
Mavlanova, T., Benbunan-Fich, R. and Koufaris, M. (2012), “Signaling theory and information asymmetry in online commerce”, Information and Management, Vol. 49 No. 5, pp. 240-247.
Messerschmidt, C.M. and Hinz, O. (2013), “Explaining the adoption of grid computing: an integrated institutional theory and organizational capability approach”, Journal of Strategic Information Systems, Vol. 22 No. 2, pp. 137-156.
Muangmee, C., Kot, S., Meekaewkunchorn, N., Kassakorn, N. and Khalid, B. (2021), “Factors determining the behavioral intention of using food delivery apps during COVID-19 pandemics”, Journal of Theoretical and Applied Electronic Commerce Research, Vol. 16 No. 5, pp. 1297-1310.
Orji, I.J., Kusi-Sarpong, S., Huang, S. and Vazquez-Brust, D. (2020), “Evaluating the factors that influence blockchain adoption in the freight logistics industry”, Transportation Research E: Logistics and Transportation Review, Vol. 141, 102025.
Park, E., Baek, S., Ohm, J. and Chang, H.J. (2014), “Determinants of player acceptance of mobile social network games: an application of extended technology acceptance model”, Telematics and Informatics, Vol. 31 No. 1, pp. 3-15.
Patel, K.K., Patel, S.M. and Scholar, P. (2016), “Internet of things-IOT: definition, characteristics, architecture, enabling technologies, application and future challenges”, International Journal of Engineering Science and Computing, Vol. 6 No. 5, pp. 6122-6131.
Patil, K. (2016), “Retail adoption of internet of things: applying TAM model”, In 2016 International conference on computing, analytics and security trends (CAST), pp. 404-409.
Poenicke, O., Groneberg, M. and Richter, K. (2019), “Method for the planning of IoT use cases in smart logistics zones”, IFAC-PapersOnLine, Vol. 52 No. 13, pp. 2449-2454.
Pribyl, O., Pribyl, P., Lom, M. and Svitek, M. (2019), “Modeling of smart cities based on ITS architecture”, IEEE Intell Transp Syst Magazine, Vol. 11 No. 4, pp. 28-36.
Rahman, S., Ahsan, K., Yang, L. and John, O. (2019), “An investigation into critical challenges for multinational third-party logistics providers operating in China”, Journal of Business Research, Vol. 103 August 2017, pp. 607-619.
Riyadh, A.N., Akter, M.S. and Islam, N. (2009), “The adoption of e-banking in developing countries: a theoretical model for SMEs”, International Review of Business Research Papers, Vol. 5 No. 6, pp. 212-230.
Rogers, E.M. (1983), Diffusion of Innovation, Free Press, Glencoe, IL New York.
Rogers, E.M. (2002), “Diffusion of preventive innovations”, Addictive Behaviours, Vol. 27, pp. 847-1048.
Routray, S.K., Akanskha, E., Sharmila, K.P., Sharma, L., Ghosh, A.D. and Pappa, M. (2021), “Narrowband IoT based support functions in smart cities”, Proceedings - International Conference on Artificial Intelligence and Smart Systems, ICAIS 2021 1459-64.
Straub, E.T. (2009), “Understanding technology adoption: theory and future directions for informal learning”, Review of Educational Research, Vol. 79 No. 2, pp. 625-649.
Strobl, A., Matzler, K., Nketia, B.A. and Veider, V. (2020), “Individual innovation behavior and firm-level exploration and exploitation: how family firms make the most of their managers”, Review of Managerial Science, Vol. 14 No. 4, pp. 809-844.
Thong, J.Y., Hong, S.J. and Tam, K.Y. (2006), “The effects of post-adoption beliefs on the expectation-confirmation model for information technology continuance”, International Journal of Human Computer Studies, Vol. 64 No. 9, pp. 799-810.
Venkatesh, V. and Davis, F.D. (1996), “A model of the antecedents of perceived ease of use: development and test”, Decision Sciences, Vol. 27 No. 3, pp. 451-481.
Venkatesh, V. and Davis, F.D. (2000), “Theoretical extension of the technology acceptance model: four longitudinal field studies”, Management Science, Vol. 46 No. 2, pp. 186-204.
Venkatesh, V., Morris, M.G., Davis, G.B. and Davis, F.D. (2003), “User acceptance of information technology: toward a unified view”, MIS Quarterly, Vol. 27 No. 3, pp. 425-478.
Venkatesh, V. and Bala, H. (2008), “Technology acceptance model 3 and a research agenda on interventions”, Decision Sciences, Vol. 39 No. 2, pp. 273-315.
Wanganoo, L. and Patil, A. (2020), Preparing for the smart cities: IoT enabled last-mile delivery, In 2020 Advances in Science and Engineering Technology International Conferences (ASET), pp. 1-6, IEEE..
Waheed, M. and Jam, F.A. (2010), “Teacher's intention to accept online education: extended TAM model”, Interdisciplinary Journal of Contemporary Research in Business, Vol. 2 No. 5, pp. 330-344.
Yi, M.Y., Fiedler, K.D. and Park, J.S. (2006), “Understanding the role of individual innovativeness in the acceptance of IT-based innovations: comparative analyses of models and measures”, Decision Sciences, Vol. 37 No. 3, pp. 393-426.
Further reading
Al-Sharafi, M.A., Arshah, R.A., Abo-Shanab, E.A. and Elayah, N. (2016), “The effect of security and privacy perceptions on customers' trust to accept internet banking services: an extension of TAM”, Journal of Engineering and Applied Sciences, Vol. 11 No. 3, pp. 545-552.
Das, C. (2011), A Study on Validity of Modified Technology Acceptance Model of Mobile, Master Thesis, RTM Nagpur University, Maharashtra.
Gao, L. and Bai, X. (2014), “A unified perspective on the factors influencing consumer acceptance of internet of things technology”, Asia Pacific Journal of Marketing and Logistics, Vol. 26 No. 2, pp. 211-231.
Ginters, E. and Martin-Gutierrez, J. (2013), “Low cost augmented reality and RFID application for logistics items visualization”, Procedia Computer Science, Vol. 26 December, pp. 3-13.
Liu, G. (2018), “Logistics service innovation based on demand”, In International Conference on Mechatronics and Intelligent Robotics, pp. 220-224.
Silva-Treviño, J.G., Macías-Hernández, B.A., Tello-Leal, E. and Delgado-Rivas, J.G. (2021), “The relationship between service quality, customer satisfaction, and customer loyalty: a case study of a trading company in Mexico”, CienciaUAT, Vol. 152 No. 2021, pp. 85-101.
Yan, J., Xin, S., Liu, Q., Xu, W., Yang, L., Fan, L. and Wang, Q. (2014), “Intelligent supply chain integration and management based on cloud of things”, International Journal of Distributed Sensor Networks, Vol. 10 No. 3, 624839.
Yang, J. (2011), Research on Acquisition Model of Enterprise IoT RFID Technology, Master Thesis, Xi’an Polytechnic University, Shaanxi.
Acknowledgements
This work was supported by INHA University research grant.