Abstract
Purpose
Understanding the supply network of construction materials used to construct shelters in refugee camps, or during the reconstruction of communities, is important as it can reveal the intricate links between different stakeholders and the volumes and speeds of material flows to the end-user. Using social network analysis (SNA) enables another dimension to be analysed – the role of commonalities. This is likely to be particularly important when attempting to replace vernacular materials with higher-performing alternatives or when encouraging the use of non-vernacular methods. This paper aims to analyse the supply networks of four different disaster-relief situations.
Design/methodology/approach
Data were collected from interviews with 272 displaced (or formally displaced) families in Afghanistan, Bangladesh, Nepal and Turkey, often in difficult conditions.
Findings
The results show that the form of the supply networks was highly influenced by the nature/cause of the initial displacement, the geographical location, the local availability of materials and the degree of support/advice given by aid agencies and or governments. In addition, it was found that SNA could be used to indicate which strategies might work in a particular context and which might not, thereby potentially speeding up the delivery of novel solutions.
Research limitations/implications
This study represents the first attempt in theorising and empirically investigating supply networks using SNA in a post-disaster reconstruction context. It is suggested that future studies might map the up-stream supply chain to include manufacturers and higher-order, out of country, suppliers. This would provide a complete picture of the origins of all materials and components in the supply network.
Originality/value
This is original research, and it aims to produce new knowledge.
Keywords
Citation
Copping, A., Kuchai, N., Hattam, L., Paszkiewicz, N., Albadra, D., Shepherd, P., Sahin Burat, E. and Coley, D. (2022), "Understanding material and supplier networks in the construction of disaster-relief shelters: the feasibility of using social network analysis as a decision-making tool", Journal of Humanitarian Logistics and Supply Chain Management, Vol. 12 No. 1, pp. 78-105. https://doi.org/10.1108/JHLSCM-01-2020-0007
Publisher
:Emerald Publishing Limited
Copyright © 2021, Alex Copping, Noorullah Kuchai, Laura Hattam, Natalia Paszkiewicz, Dima Albadra, Paul Shepherd, Esra Sahin Burat and David Coley
License
Published by Emerald Publishing Limited. This article is published under the Creative Commons Attribution (CC BY 4.0) licence. Anyone may reproduce, distribute, translate and create derivative works of this article (for both commercial and non-commercial purposes), subject to full attribution to the original publication and authors. The full terms of this licence may be seen at http://creativecommons.org/licences/by/4.0/legalcode
1. Introduction
According to UNHCR (United Nations High Commissioner for Refugees) and UNOCHA (United Nations Office for the Coordination of Humanitarian Affairs), at the end of 2018, there were almost 71 million displaced persons worldwide (UNOCHA, 2018; UNHCR, 2018; UNIDSR, 2019), and on average, every two seconds one new person is displaced somewhere around the world. UNHCR also identified that there were in excess of 300 major temporary settlements (camps) for the displaced around the world. There are 59 refugee camps recognised by UNRWA (United Nations Relief and Works Agency) in Jordan, Lebanon, Syria, the West Bank and the Gaza Strip alone (UNHCR, 2018). According to RAPTIM (2018), some of the world's largest refugee camps and their approximate population are Kutupalong in Cox's Bazaar (almost 1 million); Bidi in North-western Uganda (285,000); Dadaab Refugee Complex, Kenya (235,000); Kakuma in North-western Kenya (148,550); Nyarugusu in Kigoma, Tanzania (119,486); Zaatari and Azraq camps in Jordan (116,000) and Jabalia, on the Gaza Strip (113,990).
Unfortunately, the core infrastructure in the majority of refugee-hosting countries is poor. This has resulted in displaced living in extremely difficult conditions (Chu et al., 2014). Many live in temporary shelters that are either self-built or provided by external shelter agencies (Fayazi and Lizarralde, 2018). In internally displaced populations, the situation is similar, with a mix of agencies and citizens building the shelters with limited funds and materials.
It is known that the architectural form and materials used to construct such shelters is less than ideal in combating the environment in which the shelters are sited or in providing adequate social conditions. For example, surface temperatures inside metal clad shelters can be in excess of 48°C and the air temperature in excess of 45°C (Albadra et al., 2017). The results of a social survey carried out by Albadra et al. (2017) indicate that, for example, 85% of the surveyed families were unsatisfied or very unsatisfied in Zaatari camp, and in Azraq camp, 100% of the survey participants were unsatisfied or very unsatisfied with the thermal conditions in their shelters in summer. In addition, 55% of the families were unsatisfied or very unsatisfied with the building materials used in their shelters, and 87% of respondents had adapted their shelter (Albadra et al., 2017). This suggests there is the need to find alternative architectural designs and materials that better provide for the displaced.
1.1 Motivation for the study
This initial review of the academic literature triggered two theoretical questions. Firstly, through a better understanding of material supply networks, is it possible to accurately visualise pre-construction scenarios for particular country contexts? Secondly, could such visualisations be used to optimise decision-making for the shelter design and material usage, thereby potentially speeding up the delivery of novel solutions?
The literature identifies the humanitarian aid sector as being extremely challenging and unpredictable. The issue of time, location, scale and geography of disaster and displacement makes it even more complex (Carrasco and O'Brien, 2018). Traditional approaches to supply chain and project management in most cases are not effective options and humanitarian aid workers are forced to push the boundaries of standard project and supply chain management to deliver assistance for needy and affected populations in a timely manner (Van Wassenhove and Pedraza Martinez, 2012). Opdyke et al. (2017) identify the need for research to better understand the knowledge sources and material supply networks that communities rely on during reconstruction. This lack of understanding of hazard resistant construction knowledge and material selection options were also detailed in studies conducted by Ashdown (2011); Parrack et al. (2014) and Tran (2015).
The two initial theoretical questions, coupled with the call from the literature for the need for research, acted as the motivation for his study. Specifically, the objectives of this paper are (1) to see whether social network analysis (SNA) can provide some understanding of the diversity of where displaced people obtain their materials for shelter construction and from whom. There is a need to appreciate the degree of independence householders have in sourcing materials for themselves and the extent to which this influence is imposed by their neighbours, and aid agencies and governments. (2) To better understand the diversity of construction knowledge and design decisions of the displaced people. For example, in a particular context, do the displaced rely on their own knowledge of construction, their relatives, commercial builders or the aid agencies?
This paper addresses the objectives by reporting on a survey of four different disaster-relief situations to gain an understanding of material usage, material flows and of the current construction knowledge amongst households. The outcomes from this paper do not provide guidance on strategy development but simply illustrate what is currently happening on the ground. It is only with knowledge of the supply chains that it will be possible to influence the actors involved and to provide guidance on the use of better performing materials. This has to be coupled with a clear understanding of construction knowledge networks in order to improve the resilience of communities.
2. The context
2.1 Challenges
Lack of preparedness, shortage of time, insufficient funding, procurement and lack of tracking/tracing are noted as the biggest challenges in supply chain management within the humanitarian aid context (Seifert et al., 2018; Kolaczyk, 2009). Additionally, an inefficient supply chain can adversely affect preparedness (Costa et al., 2012). The criticality of lead-time in natural disaster scenarios is also highlighted by Helbing (2013), adding more pressure. Disasters, when they strike, can cripple communities, vital infrastructure and communication, which means the humanitarian aid workers also face distribution challenges (Chen et al., 2013; Pandey and Okazaki, 2005). Therefore, the supply chain partners and social network organisations need to work together in order to decrease distribution failures (e.g. shortage or duplication of aid assistance) or quality control aspects (e.g. reducing counterfeiting opportunities) (Soto-Viruet et al., 2013).
2.2 Supply chain, supply networks and sustainability in the humanitarian aid context
In general, there has been limited innovation in the field of supply chain management in the aid sector (Behl and Dutta, 2019; Ramsden, 2014); however, there has been a number of prominent studies conducted recently looking at material supply and recommending how the field can move forward. Celentano et al. (2019) applied a multi-scale approach considering the population in need of shelter to study the material choice and improve the reconstruction process. A different and recent perspective proposed by the same author (Celentano et al., 2020) attempted to look at the material selection in informal settlements through an integrated assessment. The authors explored the possible links between technical housing features, supply chains and knowledge flows, concluding that supply chain mapping can enhance the material selection. Other studies have focused on the issue of sustainable practices. Pomponi (Pomponi et al., 2019) examined the sustainability of post-disaster and post-conflict sheltering in Africa, introducing the use of a mixed-method framework based on the Delphi technique and the analytical hierarchy process (AHP). By applying this methodology, the authors aimed to forecast the importance of the four sustainability dimensions examined, and material selection was one of the factors influencing the four dimensions and the results. A sustainability criterion is also mentioned by Alshawawreh et al. (2020) as a possible approach for material selection in order to speed up the transition to a sustainable shelter solution. Lin et al. (2020), in their work, presented a study on relief supplies for flood disasters. The study predicted the relief material demand and supply using a big data-driven dynamic estimation model. Hendriks and Opdyke (2020) focused on the views of stakeholders. They conducted an analysis of stakeholders' perspectives during the reconstruction phase in the aftermath of Typhoon Haiyan. The authors observed the strong association between material suppliers and level of trust, suggesting that knowledge dissemination may drive material selection. Omer and Takafumi (2020) investigated the complexity of the relationship between building materials and the UN Sustainable Developing Goals. They aimed to understand how the selection of the right type of building materials can help achieve the “Sustainable Development Goals” and the alternative building materials to achieve long-lasting sustainability in the building environment. Omer and Takafumi (2020), citing from Celentano et al. (2019, 2020), described the advantages of the use of local materials (e.g. reducing poverty and vulnerability). However, on the other hand, Pomponi et al. (2019) highlighted the lack of consideration regarding shelter sustainability, and they applied an Analytical Hierarchy Process to establish the impact of technical and sustainability indicators in shelter construction. Their study highlighted the better environmental performances and lower costs of local materials and underlined the importance of speed in material delivery. Similarly, Alshawawreh et al. (2020) underlined the lack of consideration of sustainability dimensions in the provision of shelter, and they aimed to promote sustainable sheltering by identifying best practices. A similar issue was highlighted by Matard et al. (2019), where they presented an environmental impact assessment tool for shelters and assessed 81 shelter cases globally.
The application of supply networks as a decision tool in the humanitarian aid sector is also in the early stages of evolution, and focus has been placed on supply chain management in the quest to improve the quality of aid delivery according to (The) Fritz Institute (2005), who carried a large scale post-tsunami survey in south Asia and Africa. It is noticed that connections between stages in the aid supply chain are often weak (Taylor and Pettit, 2009), which can lead to wastage of up to 30% (Beresford, 1996, 1998). Given the rising scale of relief operations and the growing challenges climate change will present, the humanitarian sector and governments need to prepare and adopt improved supply systems (UNIDSR, 2019), and hence, efficiency is crucial in the distribution of emergency relief during disasters (Costa et al., 2012). This requires having a clear understanding of the networks involved. Questions such as are there enough connections?; are some of the majority of the members isolated (disconnected)?; are the right connections present or are key connections absent?; and who are the central members? all need to be asked (Dershem et al., 2011).
2.3 Network analysis
2.3.1 Supply networks
Any complex structure where organisations and their various operations are cross-linked is a network. A network enables two-way exchanges between the stakeholders or the producer and the consumers. Within a network, a chain is simply the sequential set of links. This means that a supply chain network is a series of organisational processes linked to form a chain (Day et al., 2012). Such networks are very important in the fields of logistics and business since it allows organisations to look at the big picture and give them a better understanding of the flow of materials and information. This allows organisations to not only focus on production and reception by the end-user but the whole supply chain network, thereby enabling an organisation to look at the entire movement of information and materials throughout the process. This not only allows them to create better partnerships but also makes sure that the best value is delivered to the users (Lyons et al., 2012). When networks of people or organisations are better connected, they are more cohesive, productive and resilient (Dersham et al., 2011), and a system of interconnected buyers and suppliers is better modelled as a network rather than as a linear chain (Kim et al., 2011). There are several methods to analyse and understand networks, one of the most practical being Social Network Analysis (SNA), which is adopted in this research.
2.3.2 Social network analysis
The fundamentals of social network analysis date back to 1936 in the work of Kurt Lewin, who combined mathematical characteristics with interpretative characteristics of sociology and established the core of the mathematically based field theory, the predecessor of the graph theory. In SNA, a social network can be represented by a series of nodes connected with lines. The nodes may represent a country, a community, an organisation, a team, a family or an individual who can act as a knowledge holder, transmitting emotions, values, ideas, experiences, advice, etc. others. The lines show the relation between two nodes (Sandru, 2012; Raúl Rodríguez, 2016) that each node has the capacity to transfer. SNA has been used to study community or friendship structures (Kumar et al., 2006; Wallman, 1984) and communication patterns (Koehly et al., 2003; Zack and McKenney, 1995), or to study disease spread patterns (Klovdahl, 1985). The methodological potential of SNA is also highlighted by Choi et al. (2001), such as approaching the study of supply networks from the social network perspective and by Choi and Kim (2008) and Choi and Hong (2002) in their structural embeddedness studies. However, the application of SNA in post-disaster reconstruction or in the aid sector is fairly new and evolving (Varda et al., 2009). The evidence from this literature provided the foundation for the adoption of SNA as a tool to visualise material and information flow in this paper.
3. Methodology
This study deploys a mixed-methods approach. Qualitative interviews/surveys were used to gather in-depth information about specific actors' positions, relationships and interactions. At the same time, a quantitative SNA enabled an understanding of the network's structure and components. Most social network scholars stress the considerable value that mixed-method studies can bring to the field of social network analysis and conclude that the two approaches can be mutually informative (Amos et al., 2014).
3.1 Data source
Data was gathered from 272 face-to-face interviews/surveys conducted in the four countries (Afghanistan, Bangladesh, Nepal and Turkey) during 2018, as detailed in Table 1 and Figure 1. Principally, the questionnaire established the size of the shelters, the number of occupants, what materials were used in the construction of their shelters and where and how they were acquired. The level and sources of construction knowledge were also determined. Each interview took approximately 15–25 min to complete, and they were carried out in a local language, with simultaneous translation into English. The survey questions can be found at https://researchdate.bath.ac.uk/id/eprint/707. A translated version of the questionnaire was used in the case of Afghanistan.
The details of households were anonymised, and each interviewed family was assigned a number (code) that were used as IDs for the following analysis. No rewards or incentives were paid to the survey respondents, and participation was completely voluntarily. The participants were chosen at random from those willing to take part. They were not asked about their age, gender or other personal questions (they were deemed unnecessary to achieve the objectives of the research). None of the surveys were abandoned due to incompleteness (the surveys were conducted in person, which made the response rate 100%). Each interviewer hired a local interpreter, who asked the survey questions, and the interviewers recorded the answers on survey forms in English simultaneously, except in the Afghanistan case, where a former UNHCR remote implementation partner carried out the survey on behalf of the authors. In Nepal, the research was facilitated by local NGOs, including Partnership for Sustainable Development – Nepal and local freelance researchers. In Turkey, the surveys were conducted in partnership with Mersin University.
As the intention of the survey was to test the feasibly of SNA as a visualisation tool, the samples are not intended to be representative of whole displaced populations in each country but representative (via a power calculation) of the camp/village in question.
3.2 Data analysis
Social network analysis is the study of how agents within a social network interact. These behaviours are illustrated using nodes and edges/links, which signify agents and their relationships, respectively. To perform social network analysis on the survey data, firstly a weighted adjacency matrix
From
Next, using
Degree centrality focuses on the number of edges extending from a node. An agent with many connections within a network has increased power over other agents' behaviours, and therefore, high degree centrality is associated with influential nodes. To compute this metric for node
Closeness centrality is another measure of centrality, which assesses the distance between a certain node and all other agents within the graph. High values of closeness are interpreted as a node's ability to widely spread its influence throughout the entire network. This metric for node
Betweenness centrality looks at whether, say, node
4. Results
4.1 Supplier and materials
The camps studied illustrate four different disaster self-build forms and support mechanisms (see Table 2). In Afghanistan, the families are “refugee returnees” returning to their own country but being resettled in a different part of the country. They are given some government support and have constructed temporary shelters. They wish to move back to their place of origin in Afghanistan at an appropriate time (therefore, they are mostly referred to as IDPs or returnees). In Bangladesh, Rohingya refugees from Myanmar are building emergency shelters which then become temporary shelters in refugee camps in the Upazila district of Bangladesh. The Nepalese families have rebuilt their homes after the 2015 earthquake, and in Turkey, Syrians leaving Syria across the northern border with Turkey find themselves settling in informal camps around the market gardens outside Mersin. Their shelters are temporary tent structures. The shelters studied had the summary statistics shown in Table 3.
From Table 3, it is clear that the homes in Nepal and Afghanistan are much larger and house extended families. The Syrian refugees in Turkey have stayed the longest yet their shelters are the most temporary compared to other locations studies.
4.1.1 Afghanistan
In Afghanistan, thousands of families, after returning from Pakistan and Iran, are still unable to go back to their place of origin. Displacement has become a familiar survival strategy for many Afghans and, in some cases, an inevitable part of life for two or more generations. The situation is further complicated by widespread unemployment, poverty, landlessness and a lack of basic services. Afghanistan faces one of the world's most acute internal displacement crises due to several factors, including protracted conflict, ongoing insecurity and natural hazards, with drought, floods, earthquakes, storms and avalanches causing additional displacement throughout the country each year. The Internal Displacement Monitoring Centre (IDMC), in their mid-year report of the year, 2019 recorded almost 435,000 new displacements due to natural disasters and 372,000 displacements due to armed conflict and violence between 01 Jan 2018 to 31 Dec 2018. New displacements combined with the previous ones results in about 2.6 million IDPs in total (IDMC, 2019). Therefore, camps have been established, and the government and the aid agencies support some by providing both temporary and permanent land ownership documents. The aid agencies provide standardised building plans for their shelters and some funding to support the building of the shelters. Other families are left to procure their own materials.
For the first couple of months, the newly arrived families live in those emergency shelters (tents) provided by the aid agencies. Then through a beneficiary selection committee, the eligible/qualifying families are selected to be provided with a semi-permanent two room shelter. The beneficiaries start constructing their shelters under technical supervision by the aid agencies or their partners. The beneficiaries themselves erect walls, complete the excavation and foundation masonry as part of their contribution to the shelter project – as per the UNHCR standard shelter guidelines and shelter design. Once the walls are erected, the main materials for the roof such as I-beams, T-beams, Chowka brick tiles and plastic sheets all provided to the beneficiaries by the aid agencies.
If not assisted by the aid agencies, the families choose wherever they feel safe to live in the location, but they have to either obtain the lease to the land or purchase the land to build their shelters. In this case, the procurement of materials and construction is done solely by the displaced families themselves. In these cases, the designs are mainly inspired by information from neighbours or relatives and the availability of local construction materials and skills.
Four locations were studied: (1) Sheikh Mesri Camp in Nangarhar province (zone 1), located approximately 14 km southwest of Jalalabad city, in a barren desert. The majority of the population are Pashtuns. There are many brick making factories next to this settlement; therefore, the majority of the shelters are built from baked soil bricks; however, the poorer families use mud walls or sun-dried brick walls. Beneficiaries usual purchase their other construction materials from nearby Kabul-Jalalabad highway junction or Jalalabad city centre.
(2) Shali camp in Khas Kunar district in Kunar province (zone 2) is located on the border with Pakistan. Rockets and artillery shells reach the village, causing civilian casualties and destroying villages/houses and forcing the affected families to be displaced to neighbouring villages or provinces (BBC News, 2012). Most of the displaced families have settled on state land; some live with their relatives or have constructed shelters on the land of relatives in neighbouring villages/provinces. It is a mountainous area, and most of the houses/shelters are built using dry stone masonry techniques, although some displaced families use concrete blocks because there is enough sand available in the banks of the nearby river, which has created a good local market for concrete blocks as a replacement to the traditional dry stone masonry. There are forests in the surrounding mountains, which has boosted the carpentry workshop business.
(3) Bela village and the surrounding area in Behsud district of Nangarhar province (zone 3). Following the closure of the Jalozai camp in Pakistan in April 2008, an increased number of Afghan refugees have returned to the Eastern Region. While most returnees have managed to return to their places of origin, some returnees have spontaneously settled in several locations in Nangarhar. Bela village, Tangi 1, 2 and 3 are located in a valley between Nangarhar and Kunar provinces approximately 10 km from Jalalabad city in Behsud district. Tangi 1, 2, and 3 are in barren desert-like locations with limited access to drinking water in part due to the water table being 100 meters beneath the surface. While Bela village is on flat and fertile land with irrigation water from the Kunar River. Because of its closeness and proximity to Jalalabad city, the majority of the construction materials are procured from there. Therefore, the design of shelters is also very diverse – except for those families who have benefited from aid agencies support. UNHCR and other aid agencies have completed the construction of semi-permanent two-room shelters for thousands of families in these locations.
(4) Baba Sahib Camp in Laghman province (zone 4) is located next to Jalalabad-Kabul highway, around 47 km northwest of the city of Jalalabad. It is a barren desert location. Almost all of the shelters are made of concrete blocks (Plate 1). The availability of free sand in the nearby river and in the desert has boosted the use of concrete blocks in the area. There are several small-scale concrete block making factories around the settlement.
The survey revealed there were 120 suppliers supplying 20 materials across the four locations (Figure 3). The materials range from manufactured components (windows, doors, etc.) to raw materials such as sand and cement. The refugee families have options in terms of purchasing, which allows for an open competitive market.
Computing the degree, closeness and betweenness centrality scores characterises the networks that exist between families and shelters (Table 4). However, using force-directed graphs gives a clearer, visual, understanding of what families have in common with respect to the source of materials (supplier), knowledge, or materials. Such graphs replace geographic spatial relationships with ones based on what the families have in common. Each dot represents a family/shelter. The closer the dots, the more they have in common. Each edge (line) is a different supplier, material or knowledge source. The size of a node is proportional to the number of connections/links it has (Figure 2 top graph). Therefore, a large node represents a very well-connected household with many suppliers, materials or knowledge sources in common with the other households. The graphs shown here are of the source of supply of materials the occupants used, so each line represents a common supplier or source.
In the graphs, different colours indicate households located in different villages/camps. To produce a force-directed map, an adjacency matrix must be computed first (Figure 4). This was calculated using the supplier information (see Figure 4). In the case of Afghanistan, there is an obvious clustering associated with village/zone allocation, which represents the real village locations (approximately).
In the case of Afghanistan (Figure 2), there is an obvious clustering based on the village/zone, indicating a strong commonality and possible influence from neighbours on the buying habits of others. Residents of zone 3 (Behsud village; yellow) are possibly a key influence (or share an influence) on neighbouring villages buying behaviour as they have a high betweenness and closeness score (Table 4). Zone 1 (Shekh Mesri camp; green) is highly interconnected between immediate neighbours (because the majority of them have received partial support (in kind) from the aid agencies such as roofing materials, and it is located next to brick-making factories, so the majority of them use burnt bricks to construct their shelter). Zone 2 (Shali camp; red) residents are more independent in their buying behaviour (because this is a mountainous area located next to the forest, residents can get very cheap construction wood. Stone or mud is used for construction here as well, which is almost freely available, and concrete blocks are cheap. Additionally, this zone is connected to Jalalabad city, which is a regional hub for construction materials and commerce). Although, zone 4 (Baba Sahib Camp, cyan) residents are the most independent, with low betweenness and closeness scores. However, it has a reasonably high degree centrality – so, within this village, families do share common suppliers, whereas, in zone 2°, centrality is quite low, and the families are more independent in their buying behaviour (Table 4).
These sourcing patterns can be partially explained by reviewing the locations (see Figures 2 and 3). Zone 2 (red), which is remote from the other three villages, has its own material suppliers and is assessed as having the most independent purchasing patterns. Zone 1 (green) is between zone 4 (cyan) and 3 (yellow) and has the most influence on (or commonality with) the buying behaviours of residents in other zones. An interesting point is that Zone 1 (Sheikh Mesri camp) and Zone 3 (Behsud village) share suppliers, as does zone 3 (Behsud village) and zone 4 (Baba Sahib IDP camp), as would be expected from the results shown in Figure 2, but zone 1 (Sheikh Mesri camp) and zone 4 (Baba Sahib IDP) do not share suppliers. This might seem surprising considering they are geographically close; however, because there is a large mountain and a river in between the two zones they cannot share suppliers, see map in Figure 5.
The adjacency matrices are shown below (Figure 4) are constructed such that row i corresponds to household/shelter i and column j corresponds to household/shelter j, where A(i,j) = w and w = number of wood-related items (left) and cement-related items (right) that households i and j have in common. The colour bar represents the integer w.
When focusing on selected materials, it can be shown that timber is purchased less frequently in zone 3 and 4 (Behsud village and Baba Sahib IDP camp, respectively), while cement is a commonly purchased material in zone 4 because shelters are made of concrete blocks in this location, and sand is freely available from the nearby river. However, in zone 2 (Shali camp Kunar province), this is not a common purchase, possibly because most of the shelters are made of either dry stone masonry or mud wall in this mountainous region (see Figure 5).
For the locations studied in Afghanistan, it is clear that (1) there is an open market for some building materials but a monopoly on specific materials such as steel; (2) the geographical location of the camps can mirror the influencing factor of families on the purchasing preference of both immediate and nearby village neighbours except for zones 1 and 4. It is found that all villages/zones buying behaviours are largely influenced by their geographic location.
4.1.2 Bangladesh
The families surveyed in Bangladesh were Rohingya refugees who have fled from ethnic and religious persecution in neighbouring Myanmar. The refugees are now living in the Kutupalong refugee camp (in various blocks) and the surrounding makeshift camps at Ghumdum and Balukhali in the Upazila district. As can be seen in Plate 2, the shelters are constructed from a bamboo structural frame, lattice bamboo walls and pitched roof generally covered in UNHCR plastic sheeting. A range of other materials is used to create shading and screens for privacy. The bamboo structural frame is crudely anchored into the ground. UNHCR and its partners work with community leaders to advise families on positioning and constructing their houses; however, the construction is left to the families and friends to complete. While some families have been provided with construction kits from the aid agencies consisting of four 7.5 m structural bamboo poles, a set of thin bamboo poles to construct the lattice walls as well as tarpaulin and a tool kit of hammers, nails and plastic ties, other families have had to procure all building materials for themselves.
The survey found that 24 different materials are listed by participants as being used in their shelter construction, supplied from 20 different suppliers. A force-directed graph shows there to be no apparent clustering (Figure 6 and Table 5). The refugee families appear to be strongly influenced by (or have a commonality with) all their neighbours buying behaviours – this is why the network structure ends up as one big cluster. Besides a few outliers, they all share the same suppliers. They clearly have a limited network of suppliers from which to purchase their materials, with Kutupalong Bazaar being the supplier location for 80% (in terms of the number of items) of building materials while NGOs generally provided the structural bamboo and tarpaulin.
The overall camp (Kutupalong) is split into approximate villages (blocks): Kutupalong block A (Red), block B (green); various neighbouring areas (cyan, yellow) such as Balukhali.
An analysis of the influence of shelter size was also completed (Figure 7). Again, each dot is a family/shelter, but the colour now indicates the size of the shelter. We see a highly uniform arrangement with little clustering (just one big lump of nodes), indicating that the size does not dictate material source/material choice.
In Bangladesh, 20 different materials were used in total for the 104 houses/shelters. From the results, it is apparent that the households are extremely interconnected. No significant clustering is observed and no apparent relationship between shelter size (sq. m) and materials used is witnessed. This picture, of limited variation in the material network, is also illustrated by taking a single material – cement (Figure 8). The legend shows the weight (the number of items that shelters
The key observations from the families studied in Bangladesh are (1) there is a very restricted and controlled market; (2) a strong influence of aid agencies and their partners in supplying key material items i.e. bamboo or plastic sheet; (3) In addition, it was observed while conducting the surveys that there are restrictions of “delivery sizes” due to the steep terrain.
4.1.3 Nepal
Families in three main locations in Nepal (the Kathmundu valley, Gorkha and Langtang) were interviewed to gain an understanding of how they undertook a self-rebuild approach to recovery after the 2015 earthquake. The government of Nepal encouraged self-recovery by offering loans in three instalments; technical support units were established in each municipality, and a catalogue of approved earthquake resilient houses was produced. In order to qualify for the loan, people had to own the land they intend to construct the house on and had to choose one of the pre-approved designs. However, these designs do not follow the traditional layout; they are only intended for living, and no space for keeping livestock or storing grains was included. In some locations, people used their old emergency shelters or built extensions to their new houses for storage. The government, in the initial response phase (after the earthquake), distributed the emergency shelters (with iron sheet roofs) (Plate 3). Various construction techniques are evidenced in Nepal (see Plates 4 and 5).
Construction techniques, supply chains and methods for delivery of materials varied across the different regions. In the Kathmandu valley, all sites are accessible by road, and the vast majority of houses were built using fired bricks and concrete frame constructions. Several fired bricks factories are visible in the valley. In Gorkha, the villages visited are more difficult to access; a jeep was necessary for part of the road and then on foot. Langtang is only accessible on foot after a 2–3 days walk. As such, the dominant building material was stone collected on site, the remaining materials used in the construction such as cement, steel and plywood were bought from Kathmandu, and after an eight-hour drive, they had to be either carried by labourers and donkey or dropped off by a helicopter. It is not surprising that the disaster-struck families in Nepal want to build their houses better than before, treating the disaster as an opportunity, and this is where the notion of “build back better” comes to play. Build Back Better (BBB) refers to the post-disaster recovery and reconstruction phases. The BBB principle is usually applied alongside disaster risk reduction, with the goal of reducing the built environment's vulnerability and enhancing the resilience of communities affected by disasters (UNISDR, 2015). The BBB principles are as follows: the process is owner-driven; it employs a harmonized approach; it reflects local contexts; it is based on multiple tranches of grant assistance with verification; and rebuilding aims to achieve a greater resilience than the pre-disaster situation (Moullier et al., 2015). Communities in more remote areas are more likely to lead on their own recovery and draw on the locally available resources, traditional knowledge and skills. Disasters tend to be followed by price inflation of construction materials, with prices of basic materials often doubling in the first six months following a catastrophic event. In the case of Nepal, the authors noted a loss of faith in traditional materials, which were not seen as robust enough to withstand earthquakes and floods. The communities expressed an intention to invest in construction materials perceived as both “stronger” and “modern”, such as reinforced concrete. This implies higher financial costs and causes a delay in the reconstruction process, accompanied by social vulnerability and the demise of traditional architectural patterns. In focusing on understanding material and supplier networks, we can obtain a fuller picture of Build Back Better practices, with their advantages and challenges.
The force-directed graph for the Nepalese suppliers (Figure 9) does show some loose clustering associated with the village locations but this is not as strong as that shown in Afghanistan. However, similar outcomes (to the Afghanistan cases) are observed when degree, betweenness and closeness are analysed (Table 6).
In Nepal, thirty households were surveyed and twenty suppliers were identified. As seen in Figure 9, the camps are split ino various villages/areas such as Langtang (red), Teksar (green), Kavere (cyan), neighbouring villages (yellow) and Bungamati (purple). Network visualisation in Figure 9 helps us see an apparent clustering associated with village/zone allocation.
For Nepal, we see that (1) the remoteness of the locations and the cost and complexity of delivery has encouraged natural local materials to be used (principally stone and pop up factories created to manufacture compressed Earth bricks). (2) Families have bypassed the transitional shelter construction phase moving from emergency shelters into permanent houses.
4.1.4 Turkey
33 Syrian refugee families living in temporary camps outside the city of Mersin in eastern Turkey were interviewed. The camps are located within market gardens and set up around a central permanent house belonging to the landowner (Plate 6). The camps range in size from 20 to one hundred tents. Each camp is managed by a “gangmaster” (or sergeant). The “gangmasters” all originate from the town of Urfa, a five-hour drive east from Mersin, just north of the Syrian border. It appears that they recruit the migrating Syrians as they cross into Turkey and transport them to the camps outside of Mersin. They provide a complete tent (steel frame and manufactured cover) for each family from a tent supplier in Urfa. Although there are tent manufacturers in Mersin all families we interviewed received their tent via their gangmaster from the same supplier in Urfa. The gangmaster also provides one biomass stove per tent. Each family pays rent plus a fee for electricity to the gangmaster who in, turn, pays a fee to the landlords. From speaking to the families, it appears that the gangmasters play a central role in the refugees' lives. Some refugees move between farming areas under the control of the gangmaster. The families are provided accommodation in the official shelters (i.e. the container towns). However, these families preferred to work and earn their own living. They left the container towns voluntarily or did not look for shelter in those official camps.
Once the families have settled some start to adapt their tents. The majority of tents have a second layer of blue tarpaulin over the original white manufactured tent cover. This may be because the original one starts to fail. In some cases, locally found materials are used in creative ways to improve the insulation, water resistance and general living conditions of the tents.
As the great majority of the tents comes from one supplier, the network of material supplies relates only to additional material purchased to enhance and adapt the shelters. The force-directed graph (Figure 10) for these material purchases show there to be no clustering associated with the village locations. Degree, betweenness and closeness found for the camps in Turkey are presented in Table 7.
In the case of Turkey, the camp is split into three villages (as seen in Figure 1): Adanalioglu (green); Limonlu (red) Kulak (black) and neighbouring villages (cyan). However, as seen in Figure 10 there is no apparent clustering associated with village/zone allocation and (Figure 11), neither in relation to the size of shelters (The size of shelters include wet areas such as kitchen, bathroom and toilets. Some of the shelters in this location are made two or three rooms).
In the case of Turkey. The results of the analysis of materials that households have in common are presented in Figure 10, and an almost exactly similar pattern is observed when selection of materials with respect to the shelter size is studied (Figure 11). From the results, it is obvious that there is no apparent pattern/clustering associated with the size. However, the network appears highly interconnected (showing influence on immediate neighbours), meaning that resources in these locations are almost equally available or perhaps (as mentioned earlier) the presence of those “gangmasters” has influenced the decision of households. Again, an overall observation of this case highlights that the availability of resources (information, materials and skills), geographical location and the nature or stage of displacement seems to be influential when it comes to the decision of households in relation to suppliers or materials choice.
The key observations for the Turkey study are (1) some refugee families have declined to stay in the official camps, even though they are entitled to; (2) A single supplier provides the tent structure. A monopoly on supply has been established; (3) supplier options are used in the purchase of additional materials to adapt the main structure, but no clustering is shown; (3) some refugees have been very creative in using plant boxes and other materials discarded from the market gardens bypassing the need for purchasing materials from suppliers, as shown in Plate 7.
4.2 Construction knowledge
The refugees were also asked about their design and construction knowledge. Figure 12 shows where they gained ideas for the design of their shelters. It can be seen that in Afghanistan, the great majority (42%) decided the design of their shelter by themselves, while in contrast, in Bangladesh, an almost similar fraction of families (44%) copied their neighbours' designs, which highlights how neighbours and aid agencies can influence the design decision of the displaced families (in the latter case). The level of social connectivity in the locations studied can be understood from the amount of support given to the displaced families. One example of social connectivity could be getting their shelter design ideas from their relatives (in Bangladesh only 3%, in Nepal 9%, in Afghanistan 22%, while in Turkey, such support from relatives did not exist at all).
5. Summary and conclusion
The literature in the supply chain management sector highlights that a system of interconnected buyers and suppliers is best modelled as a network rather than as a linear chain. In this study (for the first time), the supply networks of construction materials of disaster-relief shelters and reconstruction have been investigated. The data were collected using a questionnaire. 272 displaced families from four different counties (Afghanistan, Bangladesh, Nepal and Turkey) were sampled. The data (after careful tabulation) were analysed using social network analysis (SNA).
It was found that in post-disaster relief/reconstruction, the choice of materials supply was significantly influenced by neighbouring families and villages. In this study, we used the concept of degree centrality to establish how shared buying behaviour was between immediate neighbours. Betweenness centrality identified how shared buying behaviour was between neighbouring villages, and closeness centrality revealed the extent of the shared buying behaviour within entire networks.
When bringing together the results for each of the four case study countries (as shown in Table 8), a number of observations can be made. Firstly, the data from Bangladesh and Turkey show there to be a much higher degree centrality, compared to the situation in Afghanistan and Nepal, meaning the immediate neighbours are highly interconnected and have a high buying commonality with immediate neighbours. This may be explained by the fact that there are few options available to the families and because the aid agencies or gangmasters play a central role. This is in contrast to particularly the construction of the shelters in Nepal, which show the highest average betweenness centrality score. Here, influence from neighbouring villages is highly possible because of the advice and guidance provided by the Government – which is disseminated successfully between communities. Finally, the highest average closeness centrality score is for the shelters in Turkey. Clearly, as discussed in the Turkish case study, the influence and control of the gangmasters extends throughout the entire material supply network of the refugee families.
This study represents the first step in theorizing and empirically investigating supply networks using SNA concepts in a post-disaster reconstruction context. It successfully demonstrates the value and applicability of SNA in such a context. In particular, it shows that SNA has the power to help distinguish the different settings in such a way that would allow reasonable case-specific interventions to be designed, for example, the introduction of better materials. Then, predictions need to be made of whether the use of such materials might spread between populations. As other work (Ajam, 1998; Albadra et al., 2017; Al-Ghamdi, 1993; Attia, 2014; Cornaro et al., 2015; Crawford et al., 2005; Manfield, 2000; Obyn et al., 2014) has shown that the use of alternative materials (for example insulation or thermal mass) has the potential to improve living conditions and reduce morbidly and mortality, it would seem SNA can play an important role in shelter design and reconstruction methodologies, and the results and methods demonstrated here will be of practical utility to the aid community.
It is acknowledged that this study has limitations (if viewed as a survey), these primarily being the fact that only four disaster-relief situations were studied and that the sample sizes were unbalanced between the case studies. Nevertheless, the paper does empirically illustrate the usefulness of SNA in the supply of materials in a post-disaster reconstruction context, as well as providing a uniquely visceral visualisation of the purchasing patterns of displaced families which have not been previously studied in this context. It is suggested that future studies might map the upstream supply chain to include manufacturers and higher-order, out of country, suppliers. This would provide a more complete picture of the origins of all materials and components in the supply network.
It is only with a comprehensive picture of county-specific material and information networks that the two theoretical questions posed in the introduction regarding the simulation and optimisation of shelter design begin to be addressed.
Figures
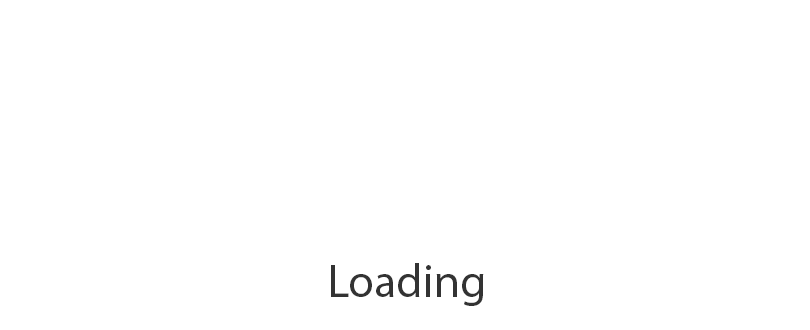
Figure 2
Force-directed graph showing commonalities in the source of materials (suppliers) in Afghanistan. Information collected using columns 2 and 3 of Q.3 from the questionnaire was used to construct this graph. The size of the nodes is proportional to the number of connections they have in the graph on the left; this is removed in the graph on the right for clarity
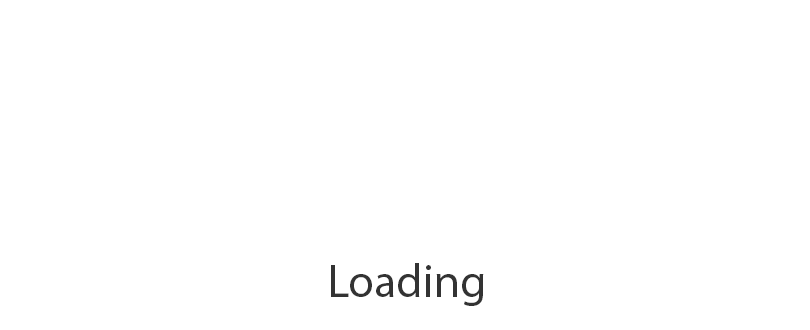
Figure 3
Geographic location of the four villages (colours are as for Figure 2)
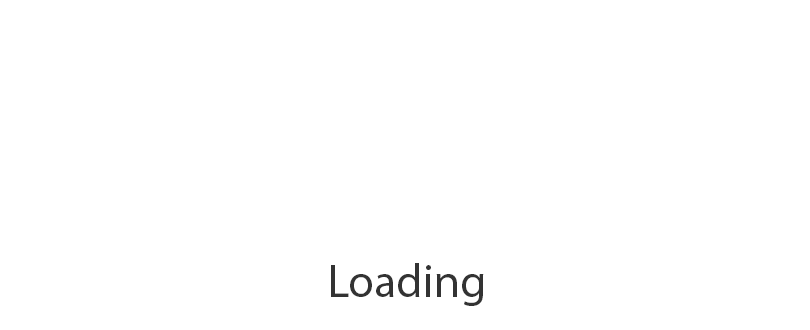
Figure 6
Force-directed graph showing commonalities in the source of materials (considering suppliers) in Bangladesh. Information collected via Q.3, column 2 and 3 from the questionnaire was used to construct this graph. The different colours indicate households located in the different villages, which form the camp
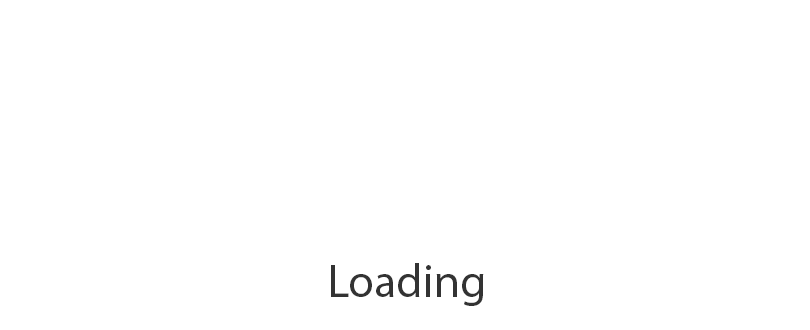
Figure 12
Source of knowledge for the design. Q.5 from Appendix was used to produce these charts
Survey sample
Location | Details | Code name | Number of families interviewed |
---|---|---|---|
Afghanistan | 25 Families in Sheikh Mesri camp | Zone 1 (green) | 100 |
25 Families in Shali camp | Zone 2 (red) | ||
25 Families in Behsud village | Zone 3 (yellow) | ||
25 Families in Baba Sahib IDP camp | Zone 4 (cyan) | ||
Bangladesh | 69 Families in Kutupalong refugee camp block A | – | 104 |
26 Families from Kutupalong block B | |||
9 Families from Balukhali Bazar and neighbouring villages | |||
Nepal | 10 Families in Kavre village | – | 35 |
6 Families in Bungamati and neighbouring villages | |||
7 Families in Teksar village | |||
12 Families in Langtang village | |||
Turkey | 22 Families in Adanalioglu village | – | 33 |
5 Families in Limonlu village | |||
6 Families in Kulak village and neighbouring villages | |||
Total | 272 |
The four self-build forms
Case | Reason for displacement | Type of rebuild | Support provided |
---|---|---|---|
Afghanistan | Armed conflict | Temporary/semi-permanent | Government loans and technical guidance |
Bangladesh | Armed conflict | Emergency/temporary | UNHCR and partners support and guidance |
Nepal | Natural disaster | Emergency/permanent | Government loans and technical guidance |
Turkey | Armed conflict | Temporary | Support from the Turkish government declined |
Summary statistics of the shelters (Q.1 and Q.2 from the questionnaire was used to collect this data)
Country | Stats | Size of house (m2) | Number of rooms per house | Length of stay (months) | Number of people per family | Number of families surveyed | Average covered area per person |
---|---|---|---|---|---|---|---|
Afghanistan | Min | 17.5 m2 | 1 | 1 | 4 | 100 | 5.80 m2 |
Max | 180 m2 | 6 | 18 | 31 | |||
Mean | 73.9 m2 | 2.7 | 8.9 | 12.7 | |||
Median | 56 m2 | 2 | 9 | 11 | |||
Bangladesh | Min | 12 m2 | 1 | 1 | 1 | 104 | 1.85 m2 |
Max | 27 m2 | 5 | 192 | 16 | |||
Mean | 11.4 m2 | 1.3 | 14.2 | 6 | |||
Median | 11 m2 | 1 | 5 | 5 | |||
Nepal | Min | 14 m2 | 1 | 1 | 2 | 35 | 5.76 m2 |
Max | 122 m2 | 10 | 108 | 27 | |||
Mean | 42.02 m2 | 3.3 | 9.9 | 7.3 | |||
Med | 40 m2 | 2 | 4 | 5 | |||
Turkey | Min | 6.8 m2 | 1 | 1 | 2 | 33 | 4.35 m2 |
Max | 100 m2 | 3 | 72 | 11 | |||
Mean | 27.8 m2 | 1.7 | 22.9 | 6.5 | |||
Median | 20 m2 | 2 | 12 | 6 |
Degree, betweenness and closeness found for the camps in Afghanistan (considering suppliers) Information collected using Q.3 from the questionnaire was used to produce this table
Zone/location | Mean degree % | Mean betweenness % | Mean closeness % |
---|---|---|---|
1 | 7.15 | 0.92 | 0.49 |
2 | 3.6 | 1.06 | 0.45 |
3 | 5.8 | 1.53 | 0.48 |
4 | 5.5 | 0.98 | 0.41 |
Total | 5.5 | 1.12 | 0.46 |
Degree, betweenness and closeness found for the camps in Bangladesh (considering suppliers). Q.3 from the questionnaire was used to produce this table
Village | Mean degree % | Mean betweenness % | Mean closeness % |
---|---|---|---|
Kutupalong block A | 13.5 | 0.74 | 0.71 |
Kutupalong block B | 17.8 | 0.31 | 0.63 |
Balukhali and others | 15.8 | 0.43 | 0.68 |
Total | 15.65 | 0.38 | 0.63 |
Degree, betweenness and closeness found for the camps in Nepal (considering suppliers). Q.3 from the questionnaire was used to produce this table
Locations | Mean degree % | Mean betweenness % | Mean closeness % |
---|---|---|---|
Teksar | 7.5 | 4.9 | 1.56 |
Kavere, Bungamati and others | 11.3 | 1.1 | 1.7 |
Langtang | 8.2 | 1.7 | 1.65 |
Total | 8.9 | 2.7 | 1.65 |
Degree, betweenness and closeness found for the camps in Turkey (considering suppliers). Information collected using Q.3 from the questionnaire was used to produce this table
Locations | Mean degree % | Mean betweenness % | Mean closeness % |
---|---|---|---|
Adanalioglu | 17.3 | 1.82 | 2.04 |
Limonlu and others | 25.8 | 1.34 | 1.94 |
Kulak | 21.3 | 1.61 | 2.00 |
Total | 17.9 | 1.80 | 2.00 |
Degree, betweenness and closeness found for all camps/villages (considering suppliers). Q.3 from the questionnaire was used to produce this table
Locations | Mean degree % | Mean betweenness % | Mean closeness % |
---|---|---|---|
Afghanistan | 5.50 | 1.12 | 0.46 |
Bangladesh | 15.65 | 0.38 | 0.63 |
Nepal | 8.90 | 2.70 | 1.65 |
Turkey | 17.90 | 1.80 | 2.00 |
The appendix contents are available in online for this article.
References
Ajam, R. (1998), Thermal Comfort in Low-Cost Refugee Shelters; a Computer Simulation Study in Waqas, a Lower Desert Valley Area in Jordan, UNRWA-HQ, Amman.
Al-Ghamdi, M.S. (1993), “Assessment and improvement on thermal conditions inside pilgrimage tents at Makkah, Saudi Arabia”, Doctoral dissertation, Newcastle University.
Albadra, D., Vellei, M., Coley, D. and Hart, J. (2017), “Thermal comfort in desert refugee camps: an interdisciplinary approach”, Building and Environment, Vol. 124, pp. 460-477, doi: 10.1016/j.buildenv.2017.08.016.
Alshawawreh, L., Pomponi, F., D'Amico, B., Snaddon, S. and Guthrie, P. (2020), “Qualifying the sustainability of novel designs and existing solutions for post-disaster and post-conflict sheltering”, Sustainability, Vol. 12 No. 3, p. 890.
Amos, A., Collin, J. and Weishaar, H. (2014), “Capturing complexity: mixing methods in the analysis of a European tobacco control policy network”, International Journal of Social Research Methodology, Vol. 18 No. 2, pp. 175-192, doi: 10.1080/13645579.2014.897851.
Ashdown, P. (2011), “Humanitarian emergency response review”, available at: www.dfid.gov.uk/emergency-response-review.
Attia, S. (2014), “Assessing the thermal performance of bedouin tents in hot climates”, in ASHRAE Energy and Indoor Environment for Hot Climates.
(The) BBC News (2012), Afghanistan-Pakistan Border Fighting Erupts in Kunar, The British Broadcasting Corporation, available at: https://www.bbc.co.uk/news/world-asia-19254623 (accessed 8 January).
Behl, A. and Dutta, P. (2019), “Humanitarian supply chain management: a thematic literature review and future directions of research”, Annals of Operations Research, Vol. 283, pp. 1001-1044.
Beresford, A. (1996), Evaluation of the Transport Sector in Rwanda, Rwanda Transport Sector Review and Action Plan, RWANDA: African Development Bank Group, Geneva.
Beresford, A. (1998), Re-Evaluation of the Transport Sector in Rwanda: an Assessment of Policy Options (Draft Report), Vol. 25, UNCTAD, Geneva.
Carrasco, S. and O'Brien, D. (2018), “The role of humanitarian agencies in reconstruction and development of disaster affected communities in Japan and the Philippines”, Procedia Engineering, Vol. 212, pp. 606-613, doi: 10.1016/j.proeng.2018.01.078.
Celentano, G., Escamilla, E.Z., Göswein, V. and Habert, G. (2019), “A matter of speed: the impact of material choice in post-disaster reconstruction”, International Journal of Disaster Risk Reduction, Vol. 34, pp. 34-44.
Celentano, G., Göswein, V., Magyar, J. and Habert, G. (2020), “The informal city as a socio-technical system: construction management and money distribution in the informal and upgraded communities of Bangkok”, Journal of Cleaner Production, Vol. 256, 120142.
Chen, J., Chen, T.H.Y., Vertinsky, I., Yumagulova, L. and Park, C. (2013), “Public-private partnerships for the development of disaster resilient communities”, Journal of Contingencies and Crisis Management, Vol. 21 No. 3, pp. 130-143, doi: 10.1111/1468-5973.12021.
Choi, T.Y., Dooley, K.J. and Rungtusanatham, M. (2001), “Supply networks and complex adaptive systems: control versus emergence”, Journal of Operations Management, Vol. 19 No. 3, pp. 351-366.
Choi, T.Y. and Hong, Y. (2002), “Unveiling the structure of supply networks: case studies in Honda, Acura, and DaimlerChrysler”, Journal of Operations Management, Vol. 20 No. 5, pp. 469-493.
Choi, T.Y. and Kim, Y. (2008), “Structural embeddedness and supplier management: a network perspective”, Journal of Supply Chain Management, Vol. 44 No. 4, pp. 5-13.
Chu, T.J., Chang, A.P., Hwang, C.L. and Lin, J.D. (2014), “Intelligent and green buildings project management scope definition using project definition rating index (PDRI)”, Advanced Materials Research, Vols 945-949, pp. 3008-3011, doi: 10.4028/www.scientific.net/AMR.945-949.3008.
Cornaro, C., Sapori, D., Bucci, F., Pierro, M. and Giammanco, C. (2015), “Thermal performance analysis of an emergency shelter using dynamic building simulation”, Energy and Buildings, Vol. 88, pp. 122-134, doi: 10.1016/j.enbuild.2014.11.055.
Costa, S.R.A.D., Campos, V.B.G. and de Mello Bandeira, R.A. (2012), “Supply chains in humanitarian operations: cases and analysis”, Procedia – Social and Behavioral Sciences, Vol. 54, pp. 598-607, doi: 10.1016/j.sbspro.2012.09.777.
Crawford, C., Manfield, P. and McRobie, A. (2005), “Assessing the thermal performance of an emergency shelter system”, Energy and Buildings, Vol. 37 No. 5, pp. 471-483, doi: 10.1016/j.enbuild.2004.09.001.
Day, J.M., Melnyk, S.A., Larson, P.D., Davis, E.W. and Whybark, D.C. (2012), “Humanitarian and disaster relief supply chains: a matter of life and death”, Journal of Supply Chain Management, Vol. 48 No. 2, pp. 21-36.
Dershem, L., Dagargulia, T., Saganelidze, L. and Roels, S. (2011), “NGO network analysis handbook: how to measure and map linkages between NGOs”, in NGO Network Analysis Handbook–Save the Children, Save the Children, Tbilisi, Georgia, Vol. 3, p. 3.
Fayazi, M. and Lizarralde, G. (2018), “Conflicts between recovery objectives: the case of housing reconstruction after the 2003 earthquake in Bam, Iran”, International Journal of Disaster Risk Reduction, Vol. 27, pp. 317-328, doi: 10.1016/j.ijdrr.2017.10.017.
(The) Fritz Institute (2005), Logistics and the Effective Delivery of Humanitarian Relief, Fritz Institute, San Francisco, California.
Helbing, D. (2013), “Globally networked risks and how to respond”, Nature, Vol. 497 No. 7447, pp. 51-59, doi: 10.1038/nature12047.
Hendriks, E. and Opdyke, A. (2020), “Knowledge adoption in post-disaster housing self-recovery”, in Disaster Prevention and Management, ahead-of-print.
IDMC. (2019), Mid-Year Figures (January to June 2019), Internal Displacement Monitoring Centre (IDMC), available at: http://www.internal-displacement.org/sites/default/files/inline-files/2019-mid-year-figures_for%20website%20upload.pdf (accessed 5 October).
Kim, Y., Choi, T.Y., Yan, T.T. and Dooley, K. (2011), “Structural investigation of supply networks: a social network analysis approach”, Journal of Operations Management, Vol. 29 No. 3, pp. 194-211.
Klovdahl, A.S. (1985), “Social networks and the spread of infectious diseases: the AIDS example”, Social Science and Medicine, Vol. 21 No. 11, pp. 1203-1216.
Koehly, L.M., Peterson, S.K., Watts, B.G., Kempf, K.K., Vernon, S.W. and Gritz, E.R. (2003), “A social network analysis of communication about hereditary nonpolyposis colorectal cancer genetic testing and family functioning”, Cancer Epidemiology and Prevention Biomarkers, Vol. 12 No. 4, pp. 304-313.
Kolaczyk, E.D. (1999), “Bayesian multiscale models for poisson processes”, Journal of the American Statistical Association, Vol. 94 No. 447, pp. 920-933, doi: 10.2307/2670007.
Kolaczyk, E.D. (2009), “Sampling and estimation in network graphs”, Statistical Analysis of Network Data, Springer, New York, NY, pp. 1-30.
Kumar, R., Novak, J. and Tomkins, A. (2006), “Structure and evolution of online social networks”, Proceedings of the 12th ACM SIGKDD International Conference, Philadelphia, Pennsylvania, pp. 611-617.
Lin, A., Wu, H., Liang, G., Cardenas-Tristan, A., Wu, X., Zhao, C. and Li, D. (2020), “A big data-driven dynamic estimation model of relief supplies demand in urban flood disaster”, International Journal of Disaster Risk Reduction, Vol. 49, 101682.
Lyons, A.C., Adrian, E., Mondragon, C., Frank, P. and Poler, R. (2012), “Glass pipelines: the role of information systems in supporting customer-driven supply chains”, in Customer-Driven Supply Chains, Springer, London, pp. 45-70.
Manfield, P. (2000), “Modelling of a cold climate emergency shelter prototype and a comparison with the United Nations Winter Tent”, Unpublished thesis, University of Cambridge, available at: http://www.shelterproject.org.
Matard, A., Kuchai, N., Allen, S., Shepherd, P., Adeyeye, K., McCullen, N. and Southwood, E. (2019), “An analysis of the embodied energy and embodied carbon of refugee shelters worldwide”, International Journal of the Constructed Environment, Vol. 10 No. 3.
Moullier, T., Krimgold, F. and Moullier, T. (2015), Building Regulation for Resilience: Managing Risks for Safer Cities, World Bank, Washington, District of Columbia.
Obyn, S., Van Moeseke, G. and Vincent, V. (2014), “The thermal performance of shelter modelling: improvement of temporary structures”, Mobile and Rapidly Assembled Structures IV, Vol. 136, p. 83.
Omer, M.A. and Noguchi, T. (2020), “A conceptual framework for understanding the contribution of building materials in the achievement of sustainable development goals (SDGs)”, Sustainable Cities and Society, Vol. 52, 101869.
Opdyke, A., Lepropre, F., Javernick-Will, A. and Koschmann, M. (2017), “Inter-organizational resource coordination in post-disaster infrastructure recovery”, Construction Management and Economics, Vol. 35 Nos 8-9, pp. 514-530, doi: 10.1080/01446193.2016.1247973.
Pandey, B.H. and Okazaki, K. (2005), “Community-based disaster management: empowering communities to cope with disaster risks”, Regional Development Dialogue, Vol. 26 No. 2, p. 52.
Parrack, C., Flinn, B. and Passey, M. (2014), “Getting the message across for safer self-recovery in post-disaster shelter”, Open House International, Vol. 39 No. 3, pp. 47-58, available at: https://radar.brookes.ac.uk/radar/items/b0bd437a-4aec-4849-a849-fb3f8fc2f0ce/1/.
Pomponi, F., Moghayedi, A., Alshawawreh, L., D'Amico, B. and Windapo, A. (2019), “Sustainability of post-disaster and post-conflict sheltering in Africa: what matters?”, Sustainable Production and Consumption, Vol. 20, pp. 140-150.
Ramsden, G.P. (2014), Managing the Humanitarian Supply Chain: A Collaborative Approach?, PhD thesis, University of Lincoln.
RAPTIM (2018), World's Largest Refugee Camps in 2018, Raptim Humanitarian Travel, available at: https://www.raptim.org/largest-refugee-camps-in-2018/ (accessed 6 November 2019).
Rodríguez, R. (2016), “Social network analysis and supply chain management”, International Journal of Production Management and Engineering, Vol. 4, p. 35, doi: 10.4995/ijpme.2016.4425.
Sandru, C. (2012), “Epistemic and methodological aspects of network analysis”, Bulletin of the Transilvania University of Braşov, Vol. 3, pp. 63-74.
Seifert, L., Kunz, N. and Gold, S. (2018), “Humanitarian supply chain management responding to refugees: a literature review”, Journal of Humanitarian Logistics and Supply Chain Management, Vol. 8, pp. 398-426.
Soto-Viruet, Y., David Menzie, W., Papp, J.F. and Yager, T.R. (2013), “An exploration in mineral supply chain mapping using tantalum as an example”, in Open-File Report, Reston, Virginia.
Taylor, D. and Pettit, S. (2009), “A consideration of the relevance of lean supply chain concepts for humanitarian aid provision”, International Journal of Services Technology and Management, Vol. 12 No. 4, pp. 430-444, doi: 10.1504/Ijstm.2009.025817.
Tran, T.A. (2015), “Post-disaster housing reconstruction as a significant opportunity to building disaster resilience: a case in Vietnam”, Natural Hazards, Vol. 79 No. 1, pp. 61-79, doi: 10.1007/s11069-015-1826-3.
UNHCR (2018), Global Trends: Forced Displacement in 2018, United Nations High Commissioner for Refugees, Geneva.
UNISDR (2015), “Sendai framework for disaster risk reduction 2015–2030”, Third World Conference on Disaster Risk Reduction, Sendai, March 14-18, 2015, pp. 1-25, available at: org/A/CONF.224/CRP.1.
UNISDR (2019), Disaster Statistics, UN Office for Disaster Risk Reduction, available at: www.unisdr.org (accessed 10 February).
UNOCHA (2018), Datatrends 2018: Global Forced Displacements, United Nations Office for the Coordination of Humanitarian Affairs, available at: http://interactive.unocha.org/publication/datatrends2017/ (accessed 12 December 2018).
Van Wassenhove, L.N. and Pedraza Martinez, A.J. (2012), “Using OR to adapt supply chain management best practices to humanitarian logistics”, International Transactions in Operational Research, Vol. 19 Nos 1-2, pp. 307-322, doi: 10.1111/j.1475-3995.2010.00792.x.
Varda, D.M., Forgette, R., Banks, D. and Contractor, N. (2009), “Social network methodology in the study of disasters: issues and insights prompted by post-Katrina research”, Population Research and Policy Review, Vol. 28 No. 1, pp. 11-29, doi: 10.1007/s11113-008-9110-9.
Wallman, S. (1984), Eight London Households, Tavistock, London.
Zack, M.H. and McKenney, J.L. (1995), “Social context and interaction in ongoing computer-supported management groups”, Organization Science, Vol. 6 No. 4, pp. 394-422.
Acknowledgements
The authors are thankful to the fund provided by EPSRC (EP/P029175/1) under the Healthy Housing for the Displaced project (HHfTD), dCarb (EP/L016869/1) University of Bath. DOI for the data is https://doi.org/10.15125/BATH-00707. We thank all those who participated in the surveys and provided valuable feedback and information.