Abstract
Purpose
The purpose of this study is to explore the process of initial sensemaking that organizational members activate when they reflect on AI adoption in their work settings, and how the perceived features of AI technologies trigger sensemaking processes which in turn have the potential to influence workplace learning modes and trajectories.
Design/methodology/approach
We adopted an explorative qualitative and interactive approach to capture free fantasies and imaginative ideas of AI among people within the industry. We adopt a conceptual perspective that combines theories on initial sensemaking and workplace learning as a theoretical lens to analyze data collected during 23 focus groups held at four large Swedish manufacturing companies. The data were analyzed using the Gioia method.
Findings
Two aggregated dimensions were defined and led to the development of an integrated conceptualization of the initial sensemaking of AI technology adoption. Specifically, sensemaking triggered by abstract features of AI technology mainly pointed to an exploitative learning path. Sensemaking triggered by concrete features of the technology mainly pointed to explorative paths, where socio-technical processes appear to be crucial in the process of AI adoption.
Originality/value
This is one of the first studies that attempts to explore and conceptualize how organizations make sense of prospective workplace learning in the context of AI adoption.
Keywords
Citation
Engström, A., Pittino, D., Mohlin, A., Johansson, A. and Edh Mirzaei, N. (2024), "Artificial intelligence and work transformations: integrating sensemaking and workplace learning perspectives", Information Technology & People, Vol. ahead-of-print No. ahead-of-print. https://doi.org/10.1108/ITP-01-2023-0048
Publisher
:Emerald Publishing Limited
Copyright © 2024, Annika Engström, Daniel Pittino, Alice Mohlin, Anette Johansson and Nina Edh Mirzaei
License
Published by Emerald Publishing Limited. This article is published under the Creative Commons Attribution (CC BY 4.0) licence. Anyone may reproduce, distribute, translate and create derivative works of this article (for both commercial and non-commercial purposes), subject to full attribution to the original publication and authors. The full terms of this licence may be seen at http://creativecommons.org/licences/by/4.0/legalcode
1. Introduction
In this study, we seek to understand the direction and implications of AI for the future of work by analyzing how people in organizations make sense of the transformation driven by AI technologies at the workplace level. In studies about the future of work, one of the main streams of research addresses technology-driven change (Carmel and Sawyer, 2023), paying particular attention to the possibilities associated with the diffusion of artificial intelligence (AI) in organizations.
AI can be defined as “the frontier of computational advancements that references human intelligence in addressing ever more complex decision-making problems” (Berente et al., 2021, p. 1435). Previous research on the future of work enabled by AI has largely emphasized dichotomies and tensions (Carmel and Sawyer, 2023), e.g. human versus algorithmic primacy in decision-making (e.g. Meijerink and Bondarouk, 2022; Santana and Cobo, 2020) and enhancement versus substitution effects between humans and technology in the execution of increasingly sophisticated tasks (e.g. Jarrahi, 2018; Boyd and Huettinger, 2019). Organizations face major challenges in managing these dichotomies and tensions and achieving a synergy between technological infrastructure and organizational processes and behaviors. Understanding the nature, scope, and magnitude of these challenges requires increased effort by researchers (Bednar and Welch, 2020; Canhoto and Clear, 2020; Makarius et al., 2020; Wiesböck and Hess, 2020).
AI’s unique characteristics question the traditional assumptions regarding digital innovation (e.g. Van Giffen et al., 2022; Sagodi et al., 2023). AI-based digital innovations inherently introduce novel uncertainties about what demands will arise related to enhanced organizational sensemaking and collective understanding of AI adoption (Sagodi et al., 2023). To learn something entirely new, humans must first become aware and take notice of the existence of the new phenomenon before being able to direct attention toward, develop an interest in, and learn about it. These processes in the initial stage of sensemaking are, in turn, crucial for the activation of learning paths in the process of AI adoption in organizations (e.g. Wijnhoven, 2022).
Therefore, the purpose of this study is to explore the process of initial sensemaking (Griffith, 1999) that organizational members activate when they reflect on AI adoption in their work settings, whereby the perceived features of AI technologies trigger sensemaking processes (Griffith, 1999; Sagodi et al., 2023), which in turn have the potential to influence workplace learning modes and trajectories (Crossan et al., 1999; March, 1991; Ellström, 2006). The perceived features of AI technologies can be viewed as being concrete, i.e. verifiable, possible to specify and describe, and more directly observable and activable by users. Conversely, so-called abstract features are not directly observable or actionable. They encompass the potential or general properties of the technology that are associated with a scientific dimension but also with ethical, philosophical, or societal dimensions. To capture these perceived features, our first research question (RQ1) is: How do features of AI technology affect the initial sensemaking that organizational members activate when anticipating the adoption of AI in their workplace? Subsequently, we theorize that these initial sensemaking attempts are precursors of workplace learning paths (e.g. Colville et al., 2016; Thomas et al., 2001) and classify them as antecedents of either “feed-forward” flows, which represent the explorative (March, 1991) bottom-up component of workplace learning, or “feed-back” flows, which represent the exploitative (March, 1991) component and are strongly related to top-down processes (Crossan et al., 1999). Therefore, our second research question (RQ2) is: What are the possible consequences of the initial sensemaking process of AI adoption on workplace learning paths?
The empirical material for the present study was collected during 23 focus groups held at four large Swedish manufacturing companies between March and June 2021. The topics of discussion for these groups concerned ideas and representations of AI adoption in their organizational contexts. Each focus group involved four to six employees, for a total of 114 study participants. The data were transcribed and analyzed using the three-step model of the approach developed by Gioia et al. (2013). Two aggregated dimensions were defined and led to the development of an integrated conceptualization of the initial sensemaking of AI technology adoption in connection to subsequent workplace learning paths.
Specifically, our key findings are that sensemaking triggered by the more abstract features of technology mainly points to an exploitative learning path. In other words, AI technology is something that is part of a predefined pool of knowledge that is expected to be taught and then used to frame the learning of new work tasks. On the other hand, sensemaking triggered by concrete features of the technology promotes explorative paths, involving the integration of the social and technical systems in AI adoption.
Our findings contribute to the literature in several ways. First, our research underscores the importance of integrating sensemaking theory (Weick, 1995; Goto, 2022) with organizational learning frameworks to enhance understanding of AI adaptation in organizations. This integration reveals how different perceptions of AI—abstract versus concrete—can significantly influence organizational learning pathways, either reinforcing traditional feedback-driven processes or encouraging proactive, exploratory learning approaches (Weick, 1995; Crossan et al., 1999; Ellström, 2006). Additionally, we propose a revised perspective on Socio-Technical Systems (STS) theory (Trist and Bamforth, 1951) to account for AI’s role not merely as a tool but as an active participant within organizational ecosystems. This expanded view emphasizes the co-evolution of human and AI agents, suggesting a more integrated approach to designing organizational structures and workflows (Makarius et al., 2020; Jarrahi, 2018; Boyd and Huettinger, 2019). Finally, our analysis suggests that traditional workplace technology adoption models (e.g. Davis, 1989; Venkatesh et al., 2003) need expansion to incorporate sensemaking processes, as these initial cognitive reactions to technology crucially predict adoption success.
2. Sensemaking of AI and workplace learning
Workplace learning is a dynamic process wherein individuals and groups acquire additional knowledge, skills, and understandings to enhance efficiency as well as innovation within the workplace. It encompasses participation in work activities, reflection on job experiences, interaction with colleagues, and access to internal and external resources. This ongoing development is essential to keep pace with industry changes, technological advancements, and evolving job requirements (Malloch et al., 2010; Ellström, 2006). Workplace learning consists of two completely different learning logics, contradictory in their character (Ellström, 2006): (1) Learning from instructions, based on cognitive processes in the organization, document structures, and things that have been thought out and decided already (thinking before acting); and (2) Learning from experiences and actions and learning from disturbances of routinized work (acting before thinking).
Crossan et al. (1999) conceptualize these two logics in two flows. The first flow, labeled “feed-forward” and directed from the individual level to the organizational level, represents the explorative component of learning. That is, how learning by individuals feeds into groups and organizations with novel insights. The feed-forward learning flow is strongly related to creative bottom-up processes and developmental learning (Ellström, 2006). It reflects on current practices, supports critical thinking, and explores new knowledge in the workplace. Exploration, in which discovery and innovation are key, is characterized by the unknown, uncertainty, and experimentation (March, 1991). In the second flow, labeled “feed-back,” the knowledge embedded in systems is transferred to groups and then to individuals. This flow is strongly related to top-down processes and adaptive learning logics (Ellström, 2006) and the exploitation of current and existing knowledge in the workplace. Exploitation is characterized by what is already known and executed; in exploitation, rules and agreed-upon procedures must be followed and adopted, and stability, efficiency, and repetition are key (Ellström, 2006; March, 1991). We here view these two logics as dualities (Farjoun, 2010) that might interfere and compete for focus and resources while simultaneously being interdependent and equally important in developing and executing new ways of thinking and working in organizations. If these two logics are treated separately, discrepancies in the organization might cause ineffectiveness and unsuccess with necessary change processes (Farjoun, 2010).
Closely related to learning is the construct of sensemaking. Similarly, it deals with the creation of meaning, the necessity for action to be linked with cognition, and the importance of both subjective and objective knowledge. The processes of sensemaking influence ways of thinking and approaching learning in the organization (Schwandt, 2005). Conflicts and tensions may arise when individuals or organizations are resistant to challenging their assumptions and embracing new perspectives. Effectively navigating these tensions can foster a dynamic learning environment where sensemaking processes enhance different learning logics and vice versa (Schwandt, 2005; Ellström, 2006).
The idea of sensemaking emphasizes the cognitive processes through which individuals within organizations grasp and interpret complex and ambiguous situations (Weick, 1995; Maitlis, 2005). Through sensemaking, individuals construct and frame their environments and enact behaviors in interactions with others. In the context of AI adoption, sensemaking is particularly relevant to understanding how members of organizations perceive the concrete versus abstract features of AI technology. Concrete features can be directly and specifically described and verified (literally on the floor), while abstract features are described more indirectly or generally and tend to take on imaginative forms such as visions or fantasies (Griffith, 1999).
Drawing by analogy on previous literature on technology features and sensemaking (Griffith, 1999; Cheikh-Ammar, 2018), in the case of AI, abstract features encompass the underlying principles, methodologies, and ethical considerations that, while not directly observable, are foundational to AI’s functionality and societal implications. These could include the association with complex algorithms, the processes underlying machine learning that enable AI to derive insights and improve over time, predictive analytics for forecasting based on historical data, the conceptual understanding of AI’s cognitive capabilities akin to human intelligence, and the ethical implications surrounding AI development and deployment, such as data privacy, security protocols, and adherence to ethical AI principles. Conversely, concrete features of AI are tangible, directly observable, or interactable components that users can engage with, showcasing the practical applications of AI in real-world scenarios. Examples of such features could include graphical user interfaces (UIs) of AI systems that facilitate user interaction, like chatbots for customer service that provide real-time communication capabilities; specific, visible outcomes produced by AI, such as the identification of objects in an image by AI-powered image recognition tools; the accuracy of voice recognition in virtual assistants; and predictive text functionalities in messaging apps. Furthermore, concrete applications also involve generative AI software for content creation, the automation of repetitive tasks to enhance efficiency, physical robots deployed in workplace settings for tasks ranging from manufacturing to customer service, and AI-driven chatbots and customer service platforms that improve user experience and operational productivity.
In the context of AI adoption, especially in its very early stages, it is particularly useful to evoke the concept of prospective sensemaking (e.g. Goto, 2022). Initially, sensemaking was conceived as retrospective and focused on interpreting past actions and events (Weick, 1995). Recent research has, however, extended this perspective by acknowledging that sensemaking can also involve contemplating the future (e.g. Stigliani and Ravasi, 2012; Ganzin et al., 2020) by considering how prospective actions or inactions might influence the way both oneself and others interpret events (Gioia et al., 1994; Goto, 2022). Prospective sensemaking is acknowledged as an antecedent of organizational change dynamics, which includes learning in organizations (Goto, 2022; Kaplan and Orlikowski, 2013; Maitlis and Christianson, 2014). The role of (prospective) sensemaking is pivotal in activating learning flows, as it entails the activation of cognitive frames (e.g. Konlechner et al., 2019), which in turn determine what information members of an organization pay attention to and how they prioritize it (e.g. Richter, 1998; Crossan et al., 1999). In the context of workplace learning, this means that the frames individuals and groups use can either highlight or obscure important information (e.g. Balarezo et al., 2023). Once information is noticed, cognitive framing also guides its interpretation. This is critical for workplace learning because it affects the meaning that is ascribed to new information or experiences. It guides the formation of beliefs and heuristics regarding cause-effect relationships and “successful” paths in decision-making and, generally, in organizational action (Nadkarni and Narayanan, 2007; Vuori et al., 2023).
The prospective sensemaking of AI technology adoption can be associated with the enactment of cognitive frames that are both exploratory and exploitative (e.g. Henfridsson and Söderholm, 2000; Randhawa et al., 2021). Exploratory learning is therefore achieved “if technology is viewed as a stranger, rather than as something taken for granted and controlled” (Henfridsson and Söderholm, 2000, p. 36). For example, as argued by Henfridsson and Söderholm (2000, p. 35), citing Ciborra (1997):
Any organization which wants to successfully use the potential that resides in the integration of its activities with new technology should not take newly introduced technology for granted as something fully controlled. Doing so rarely leads to anything other than the exploitation and continuous refinement of existing knowledge. In other words, when prevented from seeing ambiguity relating to new technologies, one misses some potential for learning.
The adoption of AI technology depends crucially on the contextual sociotechnical setting where it takes place (Sagodi et al., 2023; Zammuto et al., 2007), defined by routines, technologies, norms, and social structures. When these aspects intersect with the novelty of AI technology, shared cognition is disrupted, prompting sociotechnical negotiations to restore shared understanding (Sagodi et al., 2023) and fostering a process of sensemaking.
3. Methodology
To capture people’s initial sensemaking of AI adoption, an explorative qualitative approach (Bryman, 2006) to capture free fantasies and imaginative ideas of AI was used. The study was carried out in a project that focused on the organizational aspects of AI transformation, with five manufacturing companies in Sweden actively participating in an interactive and joint learning collaborative research process (Ellström et al., 2020). The two-year project aimed to identify mechanisms that affect organizational readiness for AI. The companies were selected based on the current state of their “AI transformation” journey (all were in the introductory phase) and their history of successful research collaborations. The results in this paper are based on findings from four of the participating companies. The demographic details of the companies are presented in Table 1.
3.1 Data collection
Ellström et al. (2020) concluded that the antecedent to organizational development is most commonly a lack of knowledge production connected to a specific problem. Thus, companies may see research collaboration as beneficial (Ellström et al., 2020), and researchers, in turn, benefit from practitioners’ openness in gaining further insight into an organizational phenomenon. For these reasons, we involved the participating companies in the data collection with the aim of gaining a rich data source and increasing participant engagement. Focus group is an especially appropriate technique, given the aim of our research. The dialogue in focus groups represents socially shared knowledge (Marková et al., 2007), where informants elaborate their collective view and beliefs behind their expression of ambivalence and contradictions (O Nyumba et al., 2018). Focus groups provide access to participants’ own language, concepts, and concerns, thus offering a unique opportunity to observe the process of collective sense-making in action (Lunt and Livingstone, 1996; Wilkinson, 1998). As Belanger (2012) points out, focus group research is recommended for theorizing in the area of information systems, particularly for exploratory research on topics where concepts emerge through interactions among individuals or are initially unclear. The technique has also recently been employed in research on AI adoption challenges (e.g. Dietzmann and Duan, 2022; Misra et al., 2023). Focus groups provide rich data around the complex phenomenon where different experiences and descriptions of the phenomenon can be illuminated and reflected upon, unlike interviews, where the respondent’s statement is never questioned.
The aim of the focus groups was to explore imaginative ideas and fantasies about AI transformation within the participating companies. We designed the focus groups according to Cyr’s (2016) recommendations so that the data collection’s interactive focus group design (1) clearly stated the purpose of the study; (2) identified the unit of analysis—individuals’ shared perceptions at the group level or people’s imaginative ideas and fantasies about AI; and (3) presented questions to be posed for the collection of data. The facilitator of the discussion in our focus groups left participants free to reflect and share ideas without being steered by a set of interview questions (Denscombe, 2017). Thus, the focus group was chosen as the data collection method for this study to capture a large variety of voices concerning the introduction of AI technology in organizations.
The focus group questions were: What is your spontaneous reaction when you hear the concept of AI? What do you think AI is in practice? In what applications can AI be applied within your organization? What can AI be used for near your own work area? What consequences could AI have for your organization or work? How can your organization prepare you in the best way for the application of AI?
The focus group data collection procedure was designed as a pyramid (see Figure 1). The first four focus groups each included four to six company representatives from each of the participating companies and were facilitated by a researcher from the project. The company representatives were purposively sampled based on their leading positions and their involvement in digitalization initiatives within the companies. The focus groups lasted one hour and were conducted using the Microsoft Teams meeting platform. Following each of the first four focus groups, a 30-min training session on how to moderate a focus group was held by the lead researcher. The purpose was to make moderators feel safe enough to conduct a session on their own and to ensure that the upcoming focus groups were conducted in the same way as the earlier focus groups. The session included instruction in basic moderator techniques, as well as awareness of typical pitfalls and how to deal with or avoid them. The participating contact persons and leaders were then asked to run further focus groups within their companies using the exact same method, questions, and structure. They received a Microsoft PowerPoint presentation about how to introduce the focus group and the questions to be asked during the session. The selection process for these focus groups was conducted by each new moderator. The final sample consisted of 114 participants (23 focus groups). Because data collection occurred when COVID-19 restrictions were in place, the focus groups, which lasted between 45 and 60 min, were held online between March and June 2021.
3.2 Data analysis
The focus groups were all recorded. The transcriptions of the recordings were entered into the Nvivo software for data analysis. Recording the focus groups also ensured control of the quality of the focus group interview process. The raw data in Nvivo were analyzed according to the three-step coding procedure for creating rigor in qualitative analysis described by Gioia et al. (2013). This procedure is based on grounded theory methodology, which facilitates the generation of new knowledge and theory and was chosen to allow open coding of the rich material generated from this study (Figure 2).
The first step involved coding the raw data into first-order concepts based on what participants shared in the focus groups without any particular themes or theories in mind (open coding). Hereafter, we refer to the terminology used by the participants when describing the concepts as references (Table 2). This first step in the coding procedure resulted in 164 first-order concepts of interpreted meaning from the participants’ discussions.
The second step involved coding the first-order concepts based on similarities and differences, creating what Gioia et al. (2013) referred to as second-order themes—a second level of abstractions of the first-order concepts. This step was conducted by researchers discussing the central meaning of the first-order concepts and collaboratively deciding how the concepts related to each other. This resulted in nine second-order themes. In our study, these second-order themes are considered the results of our empirical analysis. It was between the second and third steps that the sensemaking theories (Griffith, 1999; Sagodi et al., 2023) were adopted to understand and discuss the themes. We then, as described by Gioia et al. (2013), aggregated the second-order themes into two dimensions. The third step was conducted by collaboratively discussing and theoretically analyzing how the aggregated dimensions relate to theories of workplace learning (Fuller; Crossan et al., 1999; Ellström, 2006).
Finally, it is important to note that this analysis procedure was not linear but dynamic and iterative, inspired by Gioia et al. (2013). First-order concepts and second-order themes were constantly renegotiated according to the research process and discoveries of central meaning in the collected material. Table 2 presents the number of references within the final second-order themes in the coding procedure, and the number of focus groups in which they were included.
4. Results: AI features as triggers of initial sensemaking process
Since our analysis was guided by the identification of abstract versus concrete features of AI technology as triggers of sensemaking (Griffith, 1999), two aggregated dimensions emerged. Each dimension encompasses different sub-themes, capturing sensemaking patterns.
4.1 First aggregated dimension: waiting for directions to determine how we adopt AI in the workplace
The central meaning of the first dimension, which we label “Waiting for Directions to Determine How We Adopt AI in the Workplace,” is based on four of the second-order themes: (1) ambiguous signs of resistance, (2) missing data infrastructure, (3) still on the starting blocks, and (4) unimaginable and remote. Based on the data from the participants in the focus groups, it can be seen that all the themes in this dimension were triggered by the abstract features of AI.
4.1.1 Ambiguous signs of resistance
This theme was generated from 60 references in 17 of the focus groups. Overall, the references point to expressions of a diffuse feeling related to abstract features of AI. AI adoption is taking place “somewhere else” with respect to people’s everyday work, and this “somewhere else” is not defined but rather referred to by generic attributes such as “complexity” or “unmeasurability.” A recurring observation among the participants was that the development of AI brought forth what was expressed as an unpleasant feeling. However, such indications of unpleasantness were commonly combined with the phrase “a little.” For example, common expressions included “a little creepy,” “a little frightening,” “a little scary,” and “a little troublesome.” Some level of fear was also mentioned, although typically referred to as being someone else’s and not one’s own. Some comments were related to a lack of knowledge and a concern that organizations must ensure the safety of employees during AI adoption, pointing also to the abstract features of ethical implications. Other examples of statements made during the focus groups in relation to this theme are: “It is a very big concept that you throw around, and I can imagine that people are perhaps afraid of the concept as such, which we should respect,” and “Maybe not us, who think this is exciting, but many other people.” Overall, statements like: “AI is interesting, but it is not really for us yet,” or “The idea of AI is overwhelming for many,” reflect a cautious, if not resistant, approach to proactively engaging with AI adoption in the workplace.
4.1.2 Missing data infrastructure
The theme that covers missing data infrastructure was generated from 15 references across nine focus groups. This theme revealed that organizations view AI adoption and the future use of AI as currently not present but something that will come after significant effort from the company. The change seems to be perceived as a huge step to transform from manual to automated work. The sentiment expressed in this theme revolves around the perception that current infrastructure and data quality are insufficient for the effective use of AI in decision-making and learning processes. This is mostly connected to the abstract features of AI in terms of generic “machine learning” and “predictive analytics” functionalities. With remarks such as: “We cannot start with AI learning until our data is in order,” and “The leap to AI seems too big with our current setup,” the participants exhibit a somewhat passive attitude, waiting for perfect conditions before beginning to explore AI’s potential. Discussions often revolved around how data that could be used by AI currently are of “bad quality,” making transitions to fully automated AI decision-making almost impossible. There were also several focus group discussions regarding the abstract feature of “interoperability of AI with existing systems,” particularly in connection to the move toward AI-driven operations. It was perceived as a long road to walk, involving steps that were likely bigger than the organizations could understand. The proposed transition from using AI as a decision support tool to using it for fully automated decision-making appeared to be perceived as a great challenge for the participants. Examples of statements to this effect are: “But then, to really go all the way toward an AI-driven company is a step that I believe is much larger than we think,” and “The gaps in our data constitute an uphill battle.” Here, AI is more likely to exist in the mind and is generally described as an abstract feature. The references within this theme indicate that people expect that the organization will create structural preconditions that will subsequently foster the change.
4.1.3 Still on the starting blocks
This theme was generated from 207 references across 23 focus groups. It indicates that the participants perceive their organizations as not having established any methods or courses of action on an organizational level to implement AI in the respective workplace environments. There is a vague perception, again pointing to abstract features, that “other companies have begun their AI journeys” by embracing generically defined technology features such as “Machine Learning,” “Decision Analytics,” and “AI-Assisted Decision Making,” and that nothing can stop this inevitable transformation. Participants expressed the concern that their organization was somehow falling behind and thought that it was time to start acting. However, again, the conditions for AI adoption were expected to be set and initiated “somewhere else.” Examples of relevant statements that emerged during the focus group discussions: “And so it is a journey, but at the same time you have to start the journey, otherwise you will never get anywhere,” and “I do not think we are ready, at all, thinking about that.” Again, the theme suggests that the move towards AI must be organizationally led and that individuals are not expected to explore or learn about AI independently in the context of their workplace.
4.1.4 Unimaginable and remote
Finally, the theme of AI as something that is unimaginable and remote was generated by 142 references across 23 focus groups. It suggests that participants view AI as something uncharted and remote, pointing at abstract features such as “complexity of the algorithms,” “predictive capabilities,” and “potential replacement of human intelligence.” AI adoption in the workplace is something that will happen in the future and change everything, even the very concept of the workplace. When individuals are unknowledgeable about the capabilities of AI, the change is incomprehensible, and the possibilities are limitless. In the focus group, participants considered how new technology is expected to grow and act on information beyond human control. Participants imagined a scenario of total transformation into something that cannot yet be conceptualized, with both infinite possibilities and threatening images. Participants used the phrase “the sky is the limit” and expressed the belief that AI would emerge in several areas that cannot yet be imagined, as a change that was coming but had yet to begin. Examples of statements made during the focus groups are as follows: “Because I do not think we are there, at least my imagination is not good enough to see that,” and “We are not capable, because we are not trained or we are not made to really think outside the box, on how AI will look like tomorrow.” This theme is representative of a change that is perceived as not having defined boundaries, and where people are at risk of being passive recipients of it. AI is perceived as something barely imaginable, something entirely new with highly abstract features.
4.2 Second aggregated dimension: envisioning challenges and potentials of AI in the workplace
The central meaning of the second dimension, which we label “Envisioning Challenges and Potentials of AI in the Workplace,” is based on five second-order themes: (1) AI assisting humans, (2) AI independent of humans, (3) superhuman capabilities, (4) humans as barriers, and (5) trust and control in the relationship, all of which can be viewed as being connected to AI’s concrete features by the respondents in the focus groups.
4.2.1 AI assisting humans
The first theme in the second dimension, AI assisting humans, was generated from 325 references across 23 focus groups in which the participants conveyed their expectations of what AI technology would do for them and their own possible future actions. Here, it was clear that AI was, in some respects, viewed as subordinate to humans. The concrete feature of AI taking over repetitive tasks allows employees to see a direct application of AI in their daily work. Statements like “AI can process data for me” or “AI can relieve us from boring parts of our work” demonstrate how AI is viewed as a tool to assist in existing processes. This tangible understanding of AI’s role can lead employees to explore new ways to integrate AI into their work and be motivated to learn how to work alongside these technologies, enhancing their skills and productivity. All the focus groups captured discussions of how AI will be of huge assistance in making both life and work easier for humans. There was a perception that when AI takes over work tasks that humans do not like, humans will be able to reduce their work hours, develop other skills, or find new work tasks that do not yet exist. Examples of such statements are: “Really, what I do today when processing data, it is like software, it is only numbers in and out. I think it will be very easy to construct algorithms so that AI can do that,” and “If we could get AI that gets us out of the boring parts of our work, that would be nice, right?” AI is interpreted as a concrete—literally on the floor—feature in current work processes, triggering individual initiative in exploring new possibilities.
4.2.2 AI independent of humans
This theme was generated from 84 references across 23 focus groups. Here, AI technology took a more physical form for the participants, who referred to it in comparison to what they had seen on TV and in movies. Movies and other types of media were discussed with respect to associations with AI and its evolution. Many people in the focus groups associated AI with science fiction movies in which (often human-like) robots act independently, as demonstrated by the following examples: “My spontaneous reaction is what you have seen in the movies, robots thinking for themselves,” and “My original association was Terminator, I think, when the machines took control and went to war with the humans.” Despite AI’s depiction in movies as independent entities, AI’s potential to function autonomously in concrete ways explore how such technology could be applied in real-life work scenarios, some of them being already familiar (for example, physical robots in the workplace).
4.2.3 Superhuman capabilities
The theme of “superhuman capabilities” was generated from 158 references across 23 focus groups. AI was discussed as something that is aware of how people act and respond to humans’ choices. This theme provides an additional and contrasting perspective on the relationship between humans and machines, in relation to the themes explained above. In this theme, humans are inferior to AI in certain aspects, mainly in terms of handling data and making decisions free from emotional inference. The participants focused on discussing specific and concrete tasks that AI can perform better than humans, such as conducting simulations, making selections, and engaging in interactions via chatbots with a level of accuracy that many participants found impressive. This stimulated reflections on how these AI capabilities can enhance their own decision-making processes, improve efficiency, and augment their work. For the participants, AI not involving any feelings in decision-making was perceived as a huge advantage because AI can then suggest alternative measures or courses of action based on unbiased facts. Examples of statements referring to concrete features associated with the “superhuman potential” are: “Like, making decisions about welfare payments or care queues and things like that, you can imagine many situations where a computer would probably make many adequate, correct, and statistically secure decisions.”
4.2.4 Humans as barriers
The theme of humans as barriers was generated from 92 references across 21 focus groups. The discussions revolved around the issue of how human characteristics would obstruct AI in terms of both technology development and transformation with respect to concrete workplace tasks. Considering human limitations as potential barriers to AI adoption indicates an implicit understanding of the concrete areas where AI may complement human work. The discussions juxtaposed human emotional intelligence with AI’s rational capabilities and mainly revolved around questions related to what machines and humans would do in the future in relation to specific tasks. Discussions revolved around humans as flexible, empathetic partners, difficult to replace, but who are also irrational and working against the advanced technology of AI. Further emphasis was placed on the necessity of human interaction, the “soft” and emotional aspects of workplace relationships, close encounters, negotiations, and personal chemistry, something technology cannot provide. These limitations to the technology raised several questions regarding consequences for human intelligence with the growth of AI and whether humans are at risk of being dumbed down or whether the new technology will contribute to a higher level of human intellectual capacity. Some examples of statements made during the focus groups are: “The personal exchange and negotiations and all of that, it will never be replaced by AI,” and “I do not think that ‘business feeling’ is possible to capture in an AI robot.” In this category, the participants attributed humans with concrete features with which AI could never compete.
4.2.5 Trust and control in the relationship
The theme of “trust and control in the relationship” was generated from 88 references across 22 of the focus groups. It further addressed the relationship between humans and machines. The participants referred to the concrete features of AI as an actor to which they might delegate their work tasks. Discussions were characterized by a clear need to control the technology to prevent it from “running amok” while simultaneously understanding the need for trust in the technology for the relationships among humans and machines to work. These discussions focused on practical decision-making issues in which human and algorithmic judgments were constantly compared, valued, and assessed. The conversations mostly concerned who or what should be in a decision-making position regarding this relationship and whether it was wise to give total power to AI. The focus groups engaged in many discussions about concrete workplace situations in which human judgment must interact with AI and addressed what situations should be controlled by humans and in what situations AI should be permitted to make its own choices. Thus, trust and control in the relationship were central to the discussions, as were conversations about AI and humans getting to know each other. To develop trust in the new technology, it was perceived as crucial to understand on what basis AI acts. Some examples of related statements are: “The faults could be severe if we give too much responsibility to an AI, and it goes off track of what is reasonable, which may crash into other things as it may not see the whole picture,” and “We must build some kind of respect between each other here.”
5. Possible consequences of the initial sensemaking process of AI adoption on workplace learning paths
The perceived features of AI technologies in the focus groups triggered different initial sensemaking processes that potentially influence workplace learning modes. The themes of the first dimension, revolving around the abstract features of AI in the workplace, can be interpreted as instances of sensemaking fostering a feed-back flow (Crossan et al., 1999) and an exploitative mode of learning (March, 1991), revealing a pattern where employees await directions rather than seeking out learning opportunities themselves, that is, as top-down learning processes (Ellström, 2006). This trend is particularly evident in how AI’s abstract nature seems to necessitate structured guidance for its integration and utilization within organizational settings. The expectation that the organization will set the stage for AI reflects an exploitative approach, where the responsibility for learning and adapting to AI is placed on the company’s existing knowledge systems, rather than on individual or team initiatives. This corresponds well with Crossan et al.'s (1999) understanding of a feed-back flow from the organizational level through groups to individuals.
The first dimension’s abstract features capture a general ambivalence towards AI, stemming from AI’s abstract complexities (Griffith, 1999) and ethical considerations. It contributes to an appreciation of “being on uncertain ground.” Consequently, rather than engaging proactively with AI, there is a tendency among employees to wait for organizational leaders to demarcate the boundaries of its use, ensuring safety and relevance to different roles. The abstractness of AI here prompts a desire for clear, top-down directives that can ease the perceived ethical and operational challenges of AI workplace adoption. The type of prospective inaction (Gioia et al., 1994; Goto, 2022) that the participants in this study give voice to might negatively influence the dynamics of change and learning in the industry, as highlighted also by Goto (2022), Kaplan and Orlikowski (2013), and Maitlis and Christianson (2014). As the role of (prospective) sensemaking is pivotal in activating learning flows (Crossan et al., 1999) and cognitive frames (e.g. Konlechner et al., 2019), the members of the focus groups do not see their own role and responsibility to prioritize learning for change (e.g. Richter, 1998; Crossan et al., 1999). In relation to the abstract features of AI, it seems as though the participants of the focus groups do not clearly comprehend their own roles and responsibilities to prioritize learning for change (see further, e.g.e.g. Richter, 1998; Crossan et al., 1999). This impact on possibilities for (prospective) sensemaking, which is pivotal for the shaping of cognitive frames (e.g. Konlechner et al., 2019) and activation of learning flows, is assumed to lead to inaction and stagnation.
The first dimension of abstract features further strengthens the traditional reliance on a top-down approach to workplace learning where the participants highlight concerns about limitations to the current state of data and the systems not being primed for AI adoption. The anticipation of AI as a tool for the future, once the data infrastructure is deemed ready, underscores a passive stance where the burden is on the organization to lay the groundwork. The abstract notions (Griffith, 1999; Cheikh-Ammar, 2018; Kim et al., 2009) of machine learning and predictive analytics become reasons to delay engagement with AI until the infrastructure is perfected by those at the top. This further reinforces an adaptive learning and exploitative mode (March, 1991; Ellström, 2006). Such an attitude towards learning, where employees follow rather than lead the technological exploration, fosters a “wait-and-see” approach. Moreover, the belief among the participants that other companies are advancing with AI places pressure on organizations to catch up, yet the path to doing so is not seen as the responsibility of the individual but something that requires institutional initiatives and directions, further entrenching the preference for top-down instructions. The sentiment that changes must be initiated “somewhere else” signals a passive expectation for formal direction and structured learning programs as opposed to active, learner-driven engagement with AI.
In the context of workplace learning, the perception of a need for top-down formal approaches to AI-related change can obscure important new information (e.g. Balarezo et al., 2023) in decision-making and organizational action (Nadkarni and Narayanan, 2007; Vuori et al., 2023). With AI’s capabilities and impact seen as boundless yet undefined, employees find it difficult to conceive how it might concretely impact their day-to-day work. The vast, undefined potential of AI is perceived as too distant and complex, which is likely to result in a passive stance towards, and risks leading to anything other than the exploitation and continuous refinement of existing knowledge. This vast unknown makes it seem prudent to await directives from above, reinforcing a pattern where learning and adaptation are seen as something to be dictated by the organization, based on when and how AI becomes tangible in the workplace. As Henfridsson and Söderholm (2000) mentioned, quoting Ciborra (1997), “when prevented from seeing ambiguity relating to new technologies, one misses some potential for learning.”
The second dimension of concrete features (Griffith, 1999; Cheikh-Ammar, 2018; Kim et al., 2009) instead reveals the emergence of an explorative/feed-forward learning mode (March, 1991; Crossan et al., 1999) associated with AI workplace integration. The very nature of AI as a tool that can autonomously process data, predict outcomes, and learn from interactions has a grounding effect on the participants’ perceptions and engagements with the technology. Unlike the abstract features of AI, the concrete features invite curiosity and personal initiative in bottom-up processes (see further, e.g. Ellström, 2006). Here, the participants do not perceive themselves as merely passive recipients of predetermined instructions but become “active explorers,” seeking to understand how AI’s capabilities can be harnessed in their specific work contexts. This exploration stimulates developmental learning (Ellström, 2006) in a feed-forward learning flow (Crossan et al., 1999).
The visibility of AI in tasks such as automating repetitive processes, enhancing decision-making through data analytics, and even acting independently in certain operations, transforms it from a nebulous concept into a tangible asset that employees can interact with, learn from, and influence. This tangibility fosters a sensemaking process where employees feel more empowered to experiment with AI tools, suggest applications, and contribute to the AI integration process.
Moreover, the presence of AI in the workplace as a concrete entity—be it through chatbots, predictive analytics, or automated systems—provides a direct experience that feeds into an exploratory learning mode (March, 1991). Participants engaged with AI witness its impact on their work and how they adapt their working practices accordingly. The focus groups clearly point out the socio-technical system (Sagodi et al., 2023; Zammuto et al., 2007) as crucial for explorative processes. Direct engagement with AI’s functionalities encourages a bottom-up approach to developmental learning (Ellström, 2006), where insights gained from hands-on experience with AI applications inform broader workplace learning and adaptation strategies. By prospectively (e.g. Stigliani and Ravasi, 2012; Ganzin et al., 2020) viewing AI as an assistant to humans, participants emphasize AI’s role in automating routine and repetitive tasks, freeing up human employees to focus on more complex and creative work, and opening curiosity about the technology’s potential. These prospective actions (Gioia et al., 1994; Goto, 2022) encourage a proactive approach to learning, where employees are motivated to discover new ways AI can support their work, leading to an organic, bottom-up exploration of AI capabilities (Goto, 2022; Kaplan and Orlikowski, 2013; Maitlis and Christianson, 2014). When the focus groups discussed advanced AI systems or robots performing tasks without human intervention, they provided concrete features’ (Griffith, 1999) examples of AI’s potential. The respondents in the focus groups talked creatively about how autonomous AI can be applied in their work areas, leading to explorative initiatives aimed at harnessing AI’s independent capabilities for innovative solutions, which helps to envision AI not just as a tool, but as an active agent that can contribute independently, prompting discussions and explorations on the best ways to integrate such technologies into the workflow (Nadkarni and Narayanan, 2007; Vuori et al., 2023). As employees reflect on the capabilities of AI to operate independently, as suggested by references to robots thinking for themselves, they may become curious about how to harness such autonomy in their fields, leading to a pursuit of learning opportunities that help them manage and collaborate with these systems. Similarly, AI’s ability to handle tasks beyond human capabilities (superhuman capabilities), especially in analyzing vast datasets or making predictions with high accuracy, challenges employees to rethink the nature of decision-making, problem-solving, and creative thinking. Recognizing these superhuman capabilities motivates employees to explore how AI can augment their own skills and work processes, shifting the learning mode towards a collaborative exploration where AI’s strengths complement human creativity and intuition (Sagodi et al., 2023). Furthermore, when the focus groups identified humans as barriers in AI workplace integration, it reflects their concerns about adaptability, skill gaps, and resistance to change. However, this recognition also serves as a catalyst for bottom-up learning (Ellström, 2006) initiatives aimed at bridging these gaps and learning exploratively (March, 1991). Finally, concrete features such as user interaction interfaces and error handling connect to a “participatory” learning mode, where employees are involved in setting guidelines, ethical standards, and control mechanisms, and foster a culture of trust in AI by involving those who work alongside AI in the decision-making processes. Ensuring AI’s role in the workplace reflects collective human judgment and ethical considerations. The prospective sensemaking of AI technology adoption here can be associated with an exploratory frame (e.g. Henfridsson and Söderholm, 2000; Randhawa et al., 2021).
Our findings highlight a divide within the prospective sensemaking connected to workplace AI adoption, where abstract AI features trigger exploitative cognitive frames and a top-down, “waiting for directions” thinking—which might lead to an adaptive learning process (Figure 3). Meanwhile, the concrete AI features trigger explorative cognitive frames and a bottom-up feedforward “envisioning challenges and potentials” thinking which might lead to a developmental learning process. Further, our results show that there is a risk of getting stuck in exploitation if the new technology is “taken for granted” and perceived as fully controlled by the managerial “technostructures.” At the same time, our findings indicate that the explorative learning mode is activated within the actual socio-technical work context, where the everyday interaction between AI and humans is envisioned.
6. Discussion and contributions
This study aimed to explore the process of initial sensemaking that organizational members activate when reflecting on AI adoption in their work settings. Thereby, our findings focus on how the perceived features of AI technologies trigger sensemaking processes and in turn influence workplace learning modes and trajectories.
Firstly, employees often interpret AI adoption through an exploitative lens, primarily influenced by the abstract features of AI. This perspective aligns with a “feed-back” learning path where AI is viewed as part of a predefined knowledge pool that must first be imparted and then used to define work tasks and behaviors. This approach tends to foster inaction and reluctance to embrace new methods, preferring instead the continuous refinement of existing knowledge (Henfridsson and Söderholm, 2000).
Secondly, the influence of AI’s concrete features prompts a more explorative interaction between humans and AI. This dimension highlights a dynamic where humans and technology co-evolve, utilizing each other’s capabilities within a socio-technical and collaborative framework (Sagodi et al., 2023; Jarrahi et al., 2023). Here, employees proactively envision a future where they work alongside AI, which aligns with the concept of prospective sensemaking that is critical for successful AI integration (Goto, 2022; Sagodi et al., 2023). This approach challenges traditional digital innovation assumptions and emphasizes the synergy between humans and machines, reflecting a socio-technical perspective that considers how individuals and technology collectively influence outcomes (Van Giffen et al., 2022; Sagodi et al., 2023).
By emphasizing the socio-technical view, the interaction between human and machine capabilities are highlighted. Further, such a view also suggests that evolving organizational practices must accommodate both elements. The human-machine tension observed suggests viewing AI technologies as communicative agents that actively participate in social interactions, thereby fostering a network of connectivity (Lindgren and Holmström, 2020; Sony and Naik, 2020). This socio-technical view also addresses the potential discrepancies between explorative and exploitative learning modes in AI adoption (Figure 3), suggesting a need to balance these approaches to achieve effective integration (Makarius et al., 2020; Jarrahi, 2018; Boyd and Huettinger, 2019). The current learning modes identified—adaptive and developmental—reflect separate and yet interconnected aspects of workplace learning. However, there is a pressing need for a synergy that enhances the relationship between humans and machines, particularly at the intersection of organizational, technical, and personal subsystems. This integration is crucial for moving beyond mere adaptation to a more dynamic engagement where experimentation, exploration, and negotiation become key processes facilitating the shift from passive waiting to proactive action in workplace learning (Crossan et al., 1999; Ellström, 2006).
By leveraging the interactive and integrative phases of learning, where both human and machine agents collaboratively develop new cognitive maps, organizations can foster a more explorative use of AI. This approach not only supports the development of new socio-technical systems within organizations but also recognizes machines in the learning process. This expanded view of workplace learning includes the learning capacities of both humans and machines, suggesting that AI can actively participate in and potentially initiate learning processes, thus adding a new layer to traditional models of workplace learning (Nadkarni and Narayanan, 2007; Vuori et al., 2023).
6.1 Contributions to theory
We believe that our paper makes three main theoretical contributions. First, the integration of sensemaking theory with organizational learning theories via the idea of prospective sensemaking can significantly deepen our understanding of how organizations adapt to AI technologies. In the context of AI adoption, the perceived abstractness or concreteness of AI technologies triggers different sensemaking processes that influence workplace learning pathways—either through feedback-driven or forward-looking mechanisms. This integration is crucial because it allows us to see how the interpretation of AI’s features can lead directly to strategic learning decisions. For instance, the perception of AI as an abstract concept may lead to a more cautious, feedback-driven learning approach where employees rely heavily on organizational cues and directives, as suggested by Crossan et al. (1999). Conversely, when AI is perceived as having more concrete applications, employees might engage in exploratory activities, experimenting and learning through direct interaction with AI technologies (Ellström, 2006). This suggests that sensemaking processes are not merely reactive but play a proactive role in shaping the learning culture within organizations. It challenges traditional views that separate cognition and action in organizational learning, proposing instead a model where they are interdependent. Integrating these theories could lead to new frameworks for understanding organizational change in response to technological disruption.
We also add to the sociotechnical system theory. In particular, the prospective role of AI as a socio-technical agent in our analysis suggests a need for an expanded view of Socio-Technical Systems (STS) theory. Traditionally, STS theory emphasizes the interaction between technology and human systems to optimize organizational outcomes (Trist and Bamforth, 1951). However, AI technologies present a unique challenge as they can act as agents within these systems, not just as tools. Our findings highlight how AI can participate in and influence socio-technical dynamics by providing new capabilities that redefine roles and workflows. For example, AI’s ability to process data and predict outcomes autonomously can shift the balance of decision-making and influence the dynamics of team interactions and organizational structures. This suggests that STS theory needs to consider not just the coexistence but also the co-evolution of human and technological agents within organizations. A revised STS perspective could better address how AI technologies are not merely embedded in social systems but are active participants that reshape these systems. This could lead to new insights into designing organizational structures and workflows that truly integrate the capabilities of both humans and AI technologies.
Finally, as illustrated by our analysis, the initial cognitive reactions to technology are crucial in predicting the success of technology adoption. Traditional technology adoption models primarily focus on perceived usefulness and ease of use (Davis, 1989; Venkatesh et al., 2003). However, our findings suggest that the initial sensemaking about technology—whether it is perceived as an abstract or concrete entity—can significantly influence these perceptions. For example, the abstract perception of AI might lead to uncertainties or fears about its integration, impacting the perceived ease of use and usefulness. This can delay or hinder effective adoption as employees wait for organizational directives rather than experimenting themselves. In contrast, concrete perceptions of AI can enhance perceived usefulness and ease of use, encouraging more active and immediate engagement with the technology. This indicates a need to expand technology adoption models to include sensemaking as a fundamental component of the adoption process. Understanding how employees make sense of new technologies could help predict adoption challenges and guide more effective implementation strategies.
6.2 Implications for practice
To effectively manage the integration of AI within the workplace, it is crucial for organizational leaders to initiate open dialogues with employees about the potential and challenges of AI technologies. Using focus groups as a tool for management can facilitate these discussions, ensuring they are conducted within a psychologically safe environment (Edmondson, 2012), crucial for fostering an innovative and healthy organizational culture. These dialogues are not only essential for initiating sensemaking processes regarding AI (Griffith, 1999) but also serve as a vital source for the exchange and integration of diverse ideas within the company. In practical terms, adopting AI should be approached as the creation of a new socio-technical system where AI collaborates with human efforts, transforming it from a mere technological addition into a fundamental part of the organizational workflow.
Furthermore, our findings make a case for allowing exploratory sensemaking during AI adoption rather than viewing the absence of ambiguity as an unequivocal good. This perspective encourages top executives and managers to embrace ambiguity as an opportunity for learning and innovation rather than a hindrance to progress. In particular, instead of aiming for clarity from the outset, organizations should acknowledge and even embrace ambiguity during AI adoption. This approach allows for a more flexible and adaptive response to the complexities and uncertainties inherent in integrating AI technologies. Encouraging exploratory sensemaking also empowers employees to experiment with AI technologies and test different possibilities for implementation. This freedom to explore fosters a culture of innovation where new ideas can emerge and evolve organically. Finally, in rapidly evolving technological scenarios, exploratory sensemaking enables organizations to remain agile and responsive to emerging opportunities and challenges in AI adoption.
7. Conclusions and future research directions
This study delineates how the perception and application of AI within industrial workplaces significantly shape learning pathways and influence the evolution of work processes. When AI is viewed as an abstract concept, it is typically linked with existing knowledge and processes, predominantly leading to exploitative feedback learning. Conversely, when AI is recognized as a tangible component of work processes, it becomes integral to a socio-technical system, where AI and human actions are intricately linked, thus requiring opportunities for experimentation and exploratory learning. This necessitates a proactive and action-oriented approach to managing workplace transformations, particularly when involving sophisticated technologies. The findings from this study, drawn from discussions with 114 participants across 23 focus groups, present consistent themes that corroborate other research in the domain, underscoring the need for a socio-technical perspective in understanding the dynamics of workplace changes.
Future research should aim to uncover the actual learning processes that follow the initial/prospective sensemaking stage by collecting additional longitudinal data accounting for the during and post AI implementation phases. In this way, it would be possible also to gain a deeper knowledge of the role of AI as both a technological and social agent within workplaces. It is imperative to employ multidisciplinary approaches that foster collaboration between the social and technical sciences to address the evolving demands of Industry 5.0 (Akundi et al., 2022; Nahavandi, 2019). Upcoming studies should consider how AI functions as an actor at the individual level, within organizational routines, and as a pervasive influence across various organizational layers, thereby creating a comprehensive meta-context for workplace learning. Emphasis should also be placed on exploring the ongoing dilemma between explorative and exploitative learning modes and how these can be balanced within group-level socio-technical processes during AI adoption. Finally, while our study presents an emergent finding regarding the agency of AI in the learning process, future research could engage much deeper into this phenomenon. Longitudinal studies examining the long-term effects of AI’s perceived agency on learning outcomes and user behavior could significantly contribute to our understanding and unlocking the transformative potential of AI and for guiding its successful integration into organizational structures and cultures.
Figures
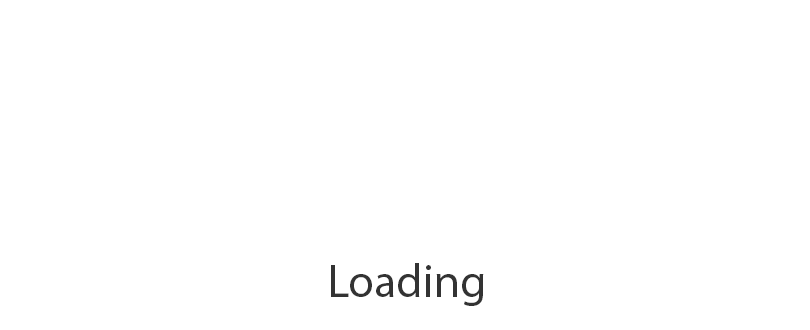
Figure 2
Data analysis step by step (Gioia et al., 2013), from raw data to aggregated dimensions
The companies that participated in the collaborative, interactive research project
Bravo | Charlie | Foxtrot | Sierra | |
---|---|---|---|---|
Year founded | 1977 | 1945 | 1893 | 1992 |
Product | C-parts manufacturer and supplier | Lighting product manufacturer | Sustainable energy products and services | Technology consultancy services |
2020 revenue (Euro) | 465,254 | 178,832 | 1,049,343 | 247,845 |
No. of employees in Sweden | 1,300 | 580 | 2,600 | 2,100 |
Source(s): Authors' own creation/work
Account of the number of references in the coding procedure
No. of references | No. of focus groups | |
---|---|---|
Signs of change is missing | 60 | 10 |
Data infrastructure is missing | 15 | 9 |
Still on the starting blocks | 207 | 23 |
Unimaginable and remote | 142 | 23 |
“Ai assist humans” | 325 | 23 |
AI independent of humans | 84 | 23 |
Superhuman capabilities | 158 | 23 |
Humans as barriers | 92 | 21 |
Trust and control in the relationship | 88 | 22 |
Source(s): Authors' own creation/work
References
Akundi, A., Euresti, D., Luna, S., Ankobiah, W., Lopes, A. and Edinbarough, I. (2022), “State of Industry 5.0—analysis and identification of current research trends”, Applied System Innovation, Vol. 5 No. 1, p. 27, doi: 10.3390/asi5010027.
Balarezo, J.D., Foss, N.J. and Nielsen, B.B. (2023), “Organizational learning: understanding cognitive barriers and what organizations can do about them”, Management Learning, Vol. 0 No. 0, pp. 1-18, doi: 10.1177/13505076231210635.
Bednar, P.M. and Welch, C. (2020), “Socio-technical perspectives on smart working: creating meaningful and sustainable systems”, Information Systems Frontiers, Vol. 22 No. 2, pp. 281-298, doi: 10.1007/s10796-019-09921-1.
Belanger, F. (2012), “Theorizing in information systems research using focus groups”, Australasian Journal of Information Systems, Vol. 17 No. 2, pp. 109-135, doi: 10.3127/ajis.v17i2.695.
Berente, N., Gu, B., Recker, J. and Santhanam, R. (2021), “Managing artificial intelligence”, MIS Quarterly, Vol. 45 No. 3, pp. 1433-1450.
Boyd, J. and Huettinger, M. (2019), “Smithian insights on automation and the future of work”, Futures, Vol. 51, pp. 104-115, doi: 10.1016/j.futures.2019.06.002.
Bryman, A. (2006), “Integrating quantitative and qualitative research: how is it done?”, Qualitative Research, Vol. 6 No. 1, pp. 97-113, doi: 10.1177/1468794106058877.
Canhoto, A.I. and Clear, F. (2020), “Artificial intelligence and machine learning as business tools: a framework for diagnosing value destruction potential”, Business Horizons, Vol. 63 No. 2, pp. 183-193, doi: 10.1016/j.bushor.2019.11.003.
Carmel, E. and Sawyer, S. (2023), “The multi-dimensional space of the futures of work”, Information Technology and People, Vol. 36 No. 1, pp. 1-20, doi: 10.1108/itp-12-2020-0857.
Cheikh-Ammar, M. (2018), “The IT artifact and its spirit: a nexus of human values, affordances, symbolic expressions, and IT features”, European Journal of Information Systems, Vol. 27 No. 3, pp. 278-294, doi: 10.1080/0960085x.2018.1436025.
Ciborra, C.U. (1997), “De profundis? Deconstructing the concept of strategic alignment”, Scandinavian Journal of Information Systems, Vol. 9 No. 1, p. 2.
Colville, I., Pye, A. and Brown, A.D. (2016), “Sensemaking processes and Weickarious learning”, Management Learning, Vol. 47 No. 1, pp. 3-13, doi: 10.1177/1350507615616542.
Crossan, M.M., Lane, H.W. and White, R.E. (1999), “An organizational learning framework: from intuition to institution”, Academy of Management Review, Vol. 24 No. 3, pp. 522-537, doi: 10.2307/259140.
Cyr, J. (2016), “The pitfalls and promise of focus groups as a data collection method”, Sociological Methods and Research, Vol. 45 No. 2, pp. 231-259, doi: 10.1177/0049124115570065.
Davis, F.D. (1989), “Perceived usefulness, perceived ease of use, and user acceptance of information technology”, MIS Quarterly, Vol. 13 No. 3, pp. 319-340, doi: 10.2307/249008.
Denscombe, M. (2017), EBOOK: the Good Research Guide: for Small-Scale Social Research Projects, McGraw-Hill Education, UK.
Dietzmann, C. and Duan, Y. (2022), “Artificial intelligence for managerial information processing and decision-making in the era of information overload”, Proceedings of the 55th Hawaii International Conference on System Sciences, pp. 5923-5932.
Edmondson, A. (2012), “Teaming: how organizations learn”, in Innovate, and Compete in the Knowledge Economy, Jossey-Bass, CA.
Ellström, P.-E. (2006), “Two logics of learning”, in Antaqnacopoulou, H. (Ed.), Learning, Working and Living: Mapping the Terrain of Working Life Learning, Palgrave Macmillan, London, pp. 33-49.
Ellström, P.-E., Elg, M., Wallo, A., Berglund, M. and Kock, H. (2020), “Interactive research: concepts, contributions and challenges”, Journal of Manufacturing Technology Management, Vol. 31 No. 8, pp. 1517-1537, doi: 10.1108/jmtm-09-2018-0304.
Farjoun, M. (2010), “Beyond dualism: stability and change as a duality”, Academy of Management Review, Vol. 35 No. 2, pp. 202-225, doi: 10.5465/amr.2010.48463331.
Ganzin, M., Islam, G. and Suddaby, R. (2020), “Spirituality and entrepreneurship: the role of magical thinking in future-oriented sensemaking”, Organization Studies, Vol. 41 No. 1, pp. 77-102, doi: 10.1177/0170840618819035.
Gioia, D.A., Thomas, J.B., Clark, S.M. and Chittipeddi, K. (1994), “Symbolism and strategic change in academia: the dynamics of sensemaking and influence”, Organization Science, Vol. 5 No. 3, pp. 363-383, doi: 10.1287/orsc.5.3.363.
Gioia, D.A., Corley, K.G. and Hamilton, A.L. (2013), “Seeking qualitative rigor in inductive research: notes on the Gioia methodology”, Organizational Research Methods, Vol. 16 No. 1, pp. 15-31, doi: 10.1177/1094428112452151.
Goto, M. (2022), “Accepting the future as ever-changing: professionals' sensemaking about artificial intelligence”, Journal of Professions and Organization, Vol. 9 No. 1, pp. 77-99, doi: 10.1093/jpo/joab022.
Griffith, T.L. (1999), “Technology features as triggers for sensemaking”, Academy of Management Review, Vol. 24 No. 3, pp. 472-488, doi: 10.2307/259137.
Henfridsson, O. and Söderholm, A. (2000), “Barriers to learning: on organizational defenses and vicious circles in technological adaptation”, Accounting, Management and Information Technologies, Vol. 10 No. 1, pp. 33-51, doi: 10.1016/s0959-8022(99)00012-0.
Jarrahi, M.H. (2018), “Artificial intelligence and the future of work: human-AI symbiosis in organizational decision making”, Business Horizons, Vol. 61 No. 4, pp. 577-586, doi: 10.1016/j.bushor.2018.03.007.
Jarrahi, M.H., Kenyon, S., Brown, A., Donahue, C. and Wicher, C. (2023), “Artificial intelligence: a strategy to harness its power through organizational learning”, Journal of Business Strategy, Vol. 44 No. 3, pp. 126-135, doi: 10.1108/jbs-11-2021-0182.
Kaplan, S. and Orlikowski, W.J. (2013), “Temporal work in strategy making”, Organization Science, Vol. 24 No. 4, pp. 965-995, doi: 10.1287/orsc.1120.0792.
Kim, H.J., Minnino, M. and Nieschwietz, R.J. (2009), “Information technology acceptance in the internal audit profession: impact of technology features and complexity”, International Journal of Accounting Information Systems, Vol. 10 No. 4, pp. 214-228, doi: 10.1016/j.accinf.2009.09.001.
Konlechner, S., Latzke, M., Güttel, W.H. and Höfferer, E. (2019), “Prospective sensemaking, frames and planned change interventions: a comparison of change trajectories in two hospital units”, Human Relations, Vol. 72 No. 4, pp. 706-732, doi: 10.1177/0018726718773157.
Lindgren, S. and Holmström, J. (2020), “A social science perspective on artificial intelligence: building blocks for a research agenda”, Journal of Digital Social Research (JDSR), Vol. 2 No. 3, pp. 1-15, doi: 10.33621/jdsr.v2i3.65.
Lunt, P. and Livingstone, S. (1996), “Rethinking the focus group in media and communications research”, Journal of Communication, Vol. 46 No. 2, pp. 79-98, doi: 10.1111/j.1460-2466.1996.tb01475.x.
Maitlis, S. (2005), “The social processes of organizational sensemaking”, Academy of Management Journal, Vol. 48 No. 1, pp. 21-49, doi: 10.5465/amj.2005.15993111.
Maitlis, S. and Christianson, M. (2014), “Sensemaking in organizations: taking stock and moving forward”, Academy of Management Annals, Vol. 8 No. 1, pp. 57-125, doi: 10.5465/19416520.2014.873177.
Makarius, E.E., Mukherjee, D., Fox, J.D. and Fox, A.K. (2020), “Rising with the machines: a sociotechnical framework for bringing artificial intelligence into the organization”, Journal of Business Research, Vol. 120, pp. 262-273, doi: 10.1016/j.jbusres.2020.07.045.
Malloch, M., Cairns, L., Evans, K. and Bridget, N.O. (2010), The SAGE Handbook of Workplace Learning, (Eds.), Sage Publications. Thousands Oaks.
March, J.G. (1991), “Exploration and exploitation in organizational learning”, Organization Science, Vol. 2 No. 1, pp. 71-87, doi: 10.1287/orsc.2.1.71.
Marková, I., Linell, P., Grossen, M. and Salazar Orvig, A. (2007), Dialogue in Focus Groups: Exploring Socially Shared Knowledge, Equinox publishing, Sheffield.
Meijerink, J. and Bondarouk, T. (2022), “The duality of algorithmic management: toward a research agenda on HRM algorithms, autonomy and value creation”, Human Resource Management Review, Vol. 33 No. 1, 100876, doi: 10.1016/j.hrmr.2021.100876.
Misra, S.K., Sharma, S.K., Gupta, S. and Das, S. (2023), “A framework to overcome challenges to the adoption of artificial intelligence in Indian Government Organizations”, Technological Forecasting and Social Change, Vol. 194, 122721, doi: 10.1016/j.techfore.2023.122721.
Nadkarni, S. and Narayanan, V.K. (2007), “The evolution of collective strategy frames in high-and low-velocity industries”, Organization Science, Vol. 18 No. 4, pp. 688-710, doi: 10.1287/orsc.1070.0268.
Nahavandi, S. (2019), “Industry 5.0—a human-centric solution”, Sustainability, Vol. 11 No. 16, pp. 4371-4385, doi: 10.3390/su11164371.
Nyumba, T.O., Wilson, K., Derrick, C.J. and Mukherjee, N. (2018), “The use of focus group discussion methodology: insights from two decades of application in conservation”, Methods in Ecology and Evolution, Vol. 9 No. 1, pp. 20-32, doi: 10.1111/2041-210x.12860.
Randhawa, K., Nikolova, N., Ahuja, S. and Schweitzer, J. (2021), “Design thinking implementation for innovation: an organization's journey to ambidexterity”, Journal of Product Innovation Management, Vol. 38 No. 6, pp. 668-700, doi: 10.1111/jpim.12599.
Richter, I. (1998), “Individual and organizational learning at the executive level: towards a research agenda”, Management Learning, Vol. 29 No. 3, pp. 299-316, doi: 10.1177/1350507698293003.
Sagodi, A., Dremel, C. and van Giffen, B. (2023), “Sensemaking in AI-based digital innovations: insights from a manufacturing case study”, ECIS 2023 Research Papers, No. 390, available at: https://aisel.aisnet.org/ecis2023_rp/390
Santana, M. and Cobo, M. (2020), “What is the future of work? A science mapping analysis”, European Management Journal, Vol. 38 No. 6, pp. 846-862, doi: 10.1016/j.emj.2020.04.010.
Schwandt, D.R. (2005), “When managers become philosophers: integrating learning with sensemaking”, Academy of Management Learning and Education, Vol. 4 No. 2, pp. 176-192, doi: 10.5465/amle.2005.17268565.
Sony, M. and Naik, S. (2020), “Critical factors for the successful implementation of Industry 4.0: a review and future research direction”, Production Planning and Control, Vol. 31 No. 10, pp. 799-815, doi: 10.1080/09537287.2019.1691278.
Stigliani, I. and Ravasi, D. (2012), “Organizing thoughts and connecting brains: material practices and the transition from individual to group-level prospective sensemaking”, Academy of Management Journal, Vol. 55 No. 5, pp. 1232-1259, doi: 10.5465/amj.2010.0890.
Thomas, J.B., Sussman, S.W. and Henderson, J.C. (2001), “Understanding “strategic learning”: linking organizational learning, knowledge management, and sensemaking”, Organization Science, Vol. 12 No. 3, pp. 331-345, doi: 10.1287/orsc.12.3.331.10105.
Trist, E.L. and Bamforth, K.W. (1951), “Some social and psychological consequences of the longwall method of coal-getting: an examination of the psychological situation and defences of a work group in relation to the social structure and technological content of the work system”, Human Relations, Vol. 4 No. 1, pp. 3-38, doi: 10.1177/001872675100400101.
Van Giffen, B., Herhausen, D. and Fahse, T. (2022), “Overcoming the pitfalls and perils of algorithms: a classification of machine learning biases and mitigation methods”, Journal of Business Research, Vol. 144, pp. 93-106, doi: 10.1016/j.jbusres.2022.01.076.
Venkatesh, V., Morris, M.G., Davis, G.B. and Davis, F.D. (2003), “User acceptance of information technology: toward a unified view”, MIS Quarterly, Vol. 27 No. 3, pp. 425-478, doi: 10.2307/30036540.
Vuori, N., Laamanen, T. and Zollo, M. (2023), “Capability development in infrequent organizational processes: unveiling the interplay of heuristics and causal knowledge”, Journal of Management Studies, Vol. 60 No. 5, pp. 1341-1381, doi: 10.1111/joms.12891.
Weick, K.E. (1995), Sensemaking in Organizations, Sage Publications, Thousand Oaks, Vol. 3.
Wiesböck, F. and Hess, T. (2020), “Digital innovations: embedding in organizations”, Electronic Markets, Vol. 30 No. 1, pp. 75-86, doi: 10.1007/s12525-019-00364-9.
Wijnhoven, F. (2022), “Organizational learning for intelligence amplification adoption: lessons from a clinical decision support system adoption project”, Information Systems Frontiers, Vol. 24 No. 3, pp. 731-744, doi: 10.1007/s10796-021-10206-9.
Wilkinson, S. (1998), “Focus group methodology: a review”, International Journal of Social Research Methodology, Vol. 1 No. 3, pp. 181-203, doi: 10.1080/13645579.1998.10846874.
Zammuto, R.F., Griffith, T.L., Majchrzak, A., Dougherty, D.J. and Faraj, S. (2007), “Information technology and the changing fabric of organization”, Organization Science, Vol. 18 No. 5, pp. 749-762, doi: 10.1287/orsc.1070.0307.
Acknowledgements
The authors acknowledge the Knowledge Foundation, Jönköping University, and the industrial partners for funding the research and education environment on Knowledge Intensive Product Realization SPARK at Jönköping University, Sweden. Project: AFAIR (No: 20200223).