Abstract
Purpose
The purpose of this research is to systematically review the properties of supply chains demonstrating that they are complex systems, and that the management of supply chains is best achieved by steering rather than controlling these systems toward desired outcomes.
Design/methodology/approach
The research study was designed as both exploratory and explanatory. Data were collected from secondary sources using a comprehensive literature review process. In parallel with data collection, data were analyzed and synthesized.
Findings
The main finding is the introduction of an inductive framework for steering supply chains from a complex systems perspective by explaining why supply chains have properties of complex systems and how to deal with their complexity while steering them toward desired outcomes. Complexity properties are summarized in four inter-dependent categories: Structural, Dynamic, Behavioral and Decision making, which together enable the assessment of supply chains as complex systems. Furthermore, five mechanisms emerged for dealing with the complexity of supply chains: classification, modeling, measurement, relational analysis and handling.
Originality/value
Recognizing that supply chains are complex systems allows for a better grasp of the effect of positive feedback on change and transformation, and also interactions leading to dynamic equilibria, nonlinearity and the role of inter-organizational learning, as well as emerging capabilities, and existing trade-offs and paradoxical tensions in decision-making. It recognizes changing dynamics and the co-evolution of supply chain phenomena in different scales and contexts.
Keywords
Citation
Abbasi, M. and Varga, L. (2022), "Steering supply chains from a complex systems perspective", European Journal of Management Studies , Vol. 27 No. 1, pp. 5-38. https://doi.org/10.1108/EJMS-04-2021-0030
Publisher
:Emerald Publishing Limited
Copyright © 2021, Maisam Abbasi and Liz Varga
License
Published in European Journal of Management Studies. Published by Emerald Publishing Limited. This article is published under the Creative Commons Attribution (CC BY 4.0) licence. Anyone may reproduce, distribute, translate and create derivative works of this article (for both commercial and non-commercial purposes), subject to full attribution to the original publication and authors. The full terms of this licence maybe seen at http://creativecommons.org/licences/by/4.0/legalcode
Introduction
The desired outcomes of managing supply chains or networks have been cited in several papers in the literature. Managing interactions among the value-adding business/work processes, organizational resources, and ties and relationships can lead to the following: optimize the configuration and utilization of resources; facilitate access to scarce inter-organizational resources, capabilities, knowledge, skills, experience, infrastructures, markets, capital and innovation; reduce transactional and total costs; increase effectiveness, efficiency and profitability; improve overall performance; align operations and strategies; match supply with demand better; improve visibility; safeguard property rights; increase customers'/consumers' satisfaction as well as service levels; share risks; and minimize uncertainties (Mentzer et al., 2001; Christopher, 2005; Greer and Lei, 2012; Dubey et al., 2020; Bowman and Collier, 2006; Huggins, 2010; van Fenema and Keers, 2018).
Although supply chain management (SCM) is underpinned by a desire for interacting and integrating (Mentzer et al., 2001; Stock and Boyer, 2009; Lambert, 2006; Drake and Schlachter, 2008; Simchi-Levi et al., 2004), it is simplistically treated in both theory and practice, given the following: the emergent properties that arise from interactions over time; improper handling of proximities, feedbacks, nonlinearities, transformation (Ojha et al., 2018), ambidexterity (Turner et al., 2013), dynamic capabilities (Ambrosini and Bowman, 2009; Vogel and Güttel, 2013), learning processes (Chiva et al., 2010), adaptive-, absorptive- and desorptive capacities (Dobrzykowski et al., 2015; Roldán Bravo et al., 2020), viability properties (Ivanov and Dolgui, 2020), resistance, conflicts, paradoxical tensions (Niesten and Stefan, 2019), path dependencies, contingencies (Bowman and Collier, 2006) and changing organizational-ownership, structure, culture, behavior (Larentis et al., 2019), identities, citizenship, power and politics; or when the extent and context dependencies of interactions are neglected.
It is also simplistic to assume that all stakeholders ranging from way upstream suppliers to far downstream customers/consumers can be correctly identified, objectively prioritized and truly integrated with error-free flows of products (goods and services) and information, due to the fact that the boundaries of control are limited to one organization and not to the end-to-end chains/networks. Furthermore, there is lack of research-based understanding regarding when or how dis-integration, re-integration or re-configuration occurs or should occur in supply chains (SCs). This could also imply challenges in data analytics (Ivanov and Dolgui, 2021a), as it is vague what is or should be included in big data, the best way to detect their anomalies, how they should be semantically interoperated and interpreted (Datta, 2015, 2017), who owns or is in charge of them, where and how long they should be stored and remembered, or how they are transformed over time.
Understanding SCs from the perspective of complex systems and their general properties can be beneficial for explaining even complex SCs, whereby the perspective explains why SCs behave as they do in reality. Complex systems are open systems which operate under quasi-equilibrium conditions (Gell-Mann, 1995), whereas their states are determined by the values of their inputs and outputs (Cilliers, 2005), resulting from the interactions of interconnected subsystems, with interactions being highly sensitive to the history of the subsystems and to their current context (McMillan, 2006; Hogue and Lord, 2007; Hoogeboom and Wilderom, 2020; Asthana et al., 2020). In addition, complex systems possess the capacity to respond to their environments in more than one way (Allen and Strathern, 2003).
A complex systems perspective can also facilitate the understanding of how SCs are more effectively managed, i.e. directed, assessed, improved and transformed toward desired outcomes. This perspective achieves this understanding by challenging simplistic, superficial and positivistic approaches, techniques, assumptions or mindsets in SCM – some examples include: objective reality, deliberate design, reductionism, uniformity, determinism, stability, rationality, equilibrium, linearity, symmetry, order, hierarchy, centralization and controllability.
The purpose of this research is to systematically review the complexity properties of SCs by demonstrating that SCs are complex systems and that the management of such systems is best achieved by steering or coaxing them. Accordingly, in order to fulfill the research purpose, the research question to be answered is: Why do SCs have properties of complex systems and how to deal with them while steering them toward desired outcomes?
By answering the research question, this study aims to theoretically introduce an inductive framework for steering SCs from a complex systems perspective by transforming their multidisciplinary conceptual understanding and research paradigm. It also aims to guide practitioners as well as decision-makers by critically challenging some of the simplistic, taken-for-granted assumptions in SCM and by recognizing supply chain systems open structures and their dynamics and behavioral patterns and also multi-scale and multi-contextual interactions while transforming them toward the desired outcomes over time.
The next sections provide an overview of the methodologies for collecting, analyzing and synthesizing data, as well as the measures for increasing the quality of research. These are followed by a findings section, followed by complementary reflections, the presentation of the inductive framework for steering SCs away from a complex systems perspective as well as the identification of research gaps in the discussion section. The paper ends with concluding remarks, possibilities for further research and critical reflections regarding research limitations.
Methodology
The research study was designed as both exploratory and explanatory (Saunders et al., 2009), in order to clarify the understanding of properties and dealing mechanisms of complex SC systems, and also to establish relationships among them, respectively. As summarized in Figure 1, the research methodology was inspired by the Sven-Step Model for a Comprehensive Literature Review (CLR), presented by Onwuegbuzie and Frels (2016).
Step 1: Exploring Beliefs and Topics
Our ontological and epistemological stance in this research was mainly that of constructionism and interpretivism, respectively (Bryman and Bell, 2007), as these better reflect the subjectivity, interactions, nonlinearities, dynamic capabilities and transformative changes that exist in management of complex SC systems over time.
Step 2: Initiating the Search
To systematically review the literature, Scopus was searched by [complex* AND {supply chain}*] in title or abstract or keywords of the documents. By limiting the search into the peer-reviewed journal articles, written in English and published until end of 2019, 4,279 hints of articles were identified. Afterward, abstracts of all the identified articles were read, from which 304 articles were determined as pertinent within the scope of this research study.
Step 3: Storing and Organizing Information
In the next step, the bibliometric information as well as access links of the pertinent articles were saved in a cloud-based version of RefWorks and considered for further investigation.
Step 4: Selecting/Deselecting Information
By skimming the whole body of the pertinent articles, 99 articles (see the “systematic literature review (SLR) references” list) were selected as relevant. To judge the relevance of the articles, their main bodies had to incorporate foundational, explicit and worthy information (such as meta-reflection, in-depth analysis, critical thinking and reflexive practices) about the complexity properties of SC systems and mechanism(s) for dealing with the complexity of SCs. Furthermore, we found that the number of relevant articles was sufficient for data saturation and for identifying the emergence of the patterns of complexity properties and dealing mechanisms. Other pertinent articles were either considered as supplementary for possible use or deselected as not useable for answering the research question in this research study.
Step 5: Expanding the Search
In parallel with the SLR, a narrative literature review (see the “narrative literature review (NLR) references” list) was performed in order to increase research authenticity by collecting further supportive references from well-established manuscripts – articulated in complexity science discipline – which address properties of complex systems in general terms than complex SCs in specific terms. The bibliometric information as well as access links of the narratively reviewed literature were also saved in the RefWorks.
Step 6: Analyzing and Synthesizing Information
Inspired by the “grounded theory” approach discussed in Strauss and Corbin (1990, 1998), Saunders et al. (2009) as well as Bryman and Bell (2007), data were analyzed in three stages: open-, axial- and selective coding. In the first stage, i.e. the open coding stage, while reading the selected articles and manuscripts, data about complexity properties of SC systems as well as mechanisms for dealing with them were chunked and grouped into smaller segments or meaningful conceptual units and given a descriptive label or code. In the axial coding stage, relationships or similarities between the open codes were identified in order to arrange and explain them into clusters of categories. This was developed to a selective coding stage, where categories were linked and integrated to form the emerged themes or what we call properties and dealing mechanisms in this research study.
Relationships among the emerged complexity properties and dealing mechanisms were further re-evaluated (Alvesson and Sandberg, 2020) and analytically synthesized (Breslin and Gatrell, 2020), based on accumulated experience, insights and wisdom (Saunders et al., 2009) during the research study. This led to generation of an inductive, conceptual framework (Post et al., 2020) for steering SCs from a complex systems perspective.
Step 7: Presenting the CLR Report
Once the first full draft of the CLR report was written, it was audited, quality checked and proofread several times before journal submission. To judge research quality, two criteria were taken into account: authenticity and trustworthiness. To increase the authenticity of the CLR, Scopus was used to select a sufficient number of relevant peer-reviewed literature which had relevance for the notion of the complexity of SCs qualitatively and/or quantitatively.
To increase trustworthiness, the systematically collected data, memos from the grounded theory and also research diaries were registered in a common database which was shared by the authors. The work-in-process text was also circulated among a number of scholars in the field, in order to align interpretations and understand data during the entire research process. Accordingly, although there is subjectivity about how the collected data was interpreted and codified, which is common in constructive anti-positivistic qualitative research, the data collection process is transparent and replicable.
Findings
The results of the analysis reveal the complexity properties of SC systems, as well as mechanisms for dealing with the complexity of SCs.
Complexity properties of supply chain systems
SCs have properties of both complicated and complex systems. As a result, SCM should go beyond silo or atomic analysis of its constituent subsystems, such as goods, services, organizational resources and stakeholders. This section presents a synthesis of such properties which have been categorized into four categories abbreviated to “SDBD properties”: Structural properties, Dynamic properties, Behavioral properties and Decision-making properties.
The SDBD properties arise from a subjectively defined “complexity profile”. A complexity profile is related to the scale of the system (i.e. the unit of analysis) and describes in detail the system and its subsystems, as well as the context in which the system operates. Scale of the system is subjective (Casti, 1994), ranging from micro (SC of a focal organization, business unit or individual) to meso (SC among clusters of organizations or industrial/economic segments) and ultimately macro (SC in the aggregate economy). Details in describing the system and its subsystems also depend on the observer, since the details reflect the interests of the observer. Context of operation is inclusive, ranging from local, urban and regional to national, multinational, continental or global. A description of those SDBD properties that could arise in every scale and context of observation from our subjective point of view is detailed below.
Structural properties
Structural properties delineate relatively static heterogeneous subsystems of SCs, shaping their nodes or vertices. As summarized in Table A1 in the Appendix, complexity arises due to the number, size, variety, extent and echelons/tiers of relatively static physical/tangible resources or components of SCs at each scale or context and the information or time required for the description of subsystems.
Dynamic properties
Dynamic properties delineate dynamic heterogeneous inter-flows and inter-processes of SCs, shaping their edges or connections or links. As summarized in Table A2 in the Appendix, complexity also arises due to the number, volume/degree, frequency, variety and extent of multiple flows of products (goods, services), movable physical/tangible-, human-, informational-, and financial resources; as well as value adding business/work processes that circulate among subsystems or are transformed by them.
Behavioral properties
As summarized in Table A3 in the Appendix, behavioral properties delineate the macro characteristics in SCs which originated from complex subsystems of the SCs and their interactions. Some of the properties which were adapted from the properties of complex adaptive systems (CAS) and are highlighted in this paper including: emergence due to interactions among subsystems and their nonlinearities over time; self-organization due to their openness and autonomy; and adaptive and evolutionary capacities of their agent-based subsystems.
Emergence. Complexity also arises on account of the degree and extent of interactions among the subsystems, i.e. the degree and extent of the effects on other subsystems while changing structural or dynamic properties of a subsystem. These interactions – which are sensitive to the history of the subsystems and to their current context (Hogue and Lord, 2007) – can lead to both linear and nonlinear behaviors in complex systems. Examples of nonlinearity can be found in system dynamics, nonlinear programing or modeling-related literature or those which reflect Forrester's flywheel effect and bullwhip effect caused by the amplification of demand distortion, rationing and shortage gaming, order batching and price fluctuations (Wilding, 1998; Wycisk et al., 2008; Surana et al., 2005; Ouyang and Li, 2010; Ma et al., 2019; Serdarasan, 2013; Tu et al., 2019), among others.
Due to the changes in the complexity profile of the system and the degree, extent, frequency and nature of its interactions, the holistic system has a dynamic macroscopic property or collective behavior (Gell-Mann, 1995) that differs from the microscopic properties or behavior of its subsystems. In other words, the whole is more than (and different in kind from) the sum of its parts (Holland, 1995; Letiche, 2000; Reitsma, 2001; Cilliers, 2005; Merali, 2006; Choi et al., 2001). Due to the presence of linearity in complicated systems, these show a simple emerging (emerging simplicity) property that makes them deterministic. Due to both linearity and nonlinearity in complex systems, they have a complex emerging (emerging complexity) property that makes them probabilistic or stochastic (Bar-Yam, 1997), with existing uncertainties and indeterminism (Nilsson and Gammelgaard, 2012), such as in the case of demand, delivery time windows, lead times, quality or quantity by suppliers, processing and manufacturing schedules and employees' behavior (de Leeuw et al., 2013; Serdarasan, 2013; Bozarth et al., 2009; Milgates, 2001; Wilding, 1998). This means that complex systems are not completely or deterministically predictable (Gershenson and Heylighen, 2004; Casti, 1994; McMillan, 2006).
As Holland (1992) and Kauffman (1995) put forward, complex systems' behaviors are semi-predictable, as they reveal underlying patterns of behavior over time, such as the business cycle exemplified by Choi et al. (2001). In managing SCs, the patterns of emergent properties of the system – what we call its capabilities – can be identified and learned. According to Olavarrieta and Ellinger (1997, p. 563), capabilities are “complex bundles of individual skills, assets and accumulated knowledge exercised through organizational processes that enable firms to co-ordinate activities and make use of their resources.” Capabilities should be directed toward the fulfillment of shared values and behaviors that lead to the emergence of desired outcomes in SC systems. With regards this, as highlighted in Abbasi (2014), in order to study SC performance and capabilities, the holistic assessments of the system – with different complexity profiles, i.e. different scales as well as contexts – must go beyond the assessment of the performance and capability of each subsystem in isolation.
Adaptation and self-organization. CAS learn from the patterns of emergent properties and react to changes based on their schemata (values, rules, norms, beliefs, assumptions, mental models and images) over time.
The schemata influence the behavior of agents [the subsystems that populate a complex system, partake in the process of spontaneous change in such a system and are able to interact meaningfully in the course of events (Choi et al., 2001)] of CAS while they are reacting to changes in their environments or creating their local surroundings. While the patterns of emergent properties and schemata are interpreted and learned over time, agents behave by self-organizing (Gershenson, 2007) based on the feedback that they receive by spontaneously rearranging their interactions with each other in the quest to optimize their overall fitness, without the need for an internal or external controller (Kauffman, 1995).
SCs are CAS because they have some subsystems with agency characteristics (e.g. intelligent goods and resources [humans, machineries, organizations]) that are able to intervene meaningfully in the course of events, or, as Bruzzone et al. (2005) highlight, because they sense and re-act to external stimuli. Complex adaptive SCs, or networks, are comprised of heterogeneous interacting agents that work under quasi-equilibrium (Li et al., 2010) and a combination of regularity and randomness (Surana et al., 2005). They show properties such as the ability to learn the patterns, self-organization, autonomy and emergent behaviors. Schemata in complex adaptive SCs should be in favor of fulfilling desired outcomes such as financially and institutionally-driven values, rules and norms which are expected to be shared among the agents throughout the system.
To adapt to the schemata, the “agency” characteristics of SCs need to increase, in order to: intelligently save, process and analyze the changes over time; identify the patterns; learn; and have the capacity to decide de-centrally. However, giving more freedom and a higher degree of autonomy to the agents can increase resilience (Casti, 1994) as well as the probability of emergence of innovative [desirable or acceptable] properties.
Self-organization and autonomy also enable the SCs to add or delete relations between agents, shift strategies in different markets (Fisher, 1997; Chand et al., 2018), adapt to different legislations (Statsenko et al., 2018; Li et al., 2010) or adapt their forms of inter-relationships – in the scale and context where they operate –, such as: vertical; horizontal; arm's length/transactional; cooperative; collaborative; competitive; coopetitive; operational; tactical; strategic partnership; joint venture; foreign direct investment (FDI); franchising; outsourced; alliance building and clustering; joint research and development; collaborative planning, forecasting and replenishment (CPFR); vendor-managed inventory (VMI); integrated product/process development; mergers and acquisitions; and joint action arrangements.
To self-organize, agents need to be open-minded and have sufficient autonomy to interact with other agents of their networks outside their functional boundaries. Ideal SCs adapt to the schemata by organizing themselves based on feedback (Choi et al., 2001; Varga et al., 2009), without an internal or external controller or a centralized decision-maker (Touboulic et al., 2018). However, opportunistic behavior, bounded rationality, lack of know-how or skills, market imperfection, asset specificity, subjectivity and asymmetry in information and also interpretation can hinder the perfect adaptation to the schemata and exclusion of control or orchestration in SCs. That is why discussion about self-organization and adaptation, which originated from natural sciences, requires more research in social sciences and applications such as SCM.
When analyzing adaptability, some papers reviewed in the literature highlight “resilience”. Hearnshaw and Wilson (2013) differentiate resilience from adaptability by relating the former to the capacity to carry out functions despite disruptions or damage by disturbance, that is, the capacity to resist change and preserve connectivity after nodal removal. The latter is then related to the capacity to adapt to novel and unexpected changes, the capacity to self-organize and reconfigure the structure and behavior to satisfy new conditions.
Other authors find overlaps between resilience and adaptability. Datta et al. (2007) define SC resilience as being not only the ability to maintain control over performance variability in the face of disturbance, but that it is also a sign of being adaptive and is capable of sustained response to sudden and significant shifts in the environment in the form of uncertain demands. Accordingly, a resilient system is expected to return to its original state or a better state after turbulence and disruption (Olivares Agulia and ElMarapgy, 2018). Asokan et al. (2017) identify three different articulations of resilience, namely: “engineering resilience”, which is the same as the term of elasticity and emphasizes the time a system takes to return to equilibrium or a steady state; “ecological resilience” accepts the presence of multiple stability regions and measures the amount of perturbation which the system can absorb; whereas “adaptive cycle of resilience” captures the concept of continuous change, which has four stages, namely: exploitation (r), conservation (K), release (Ω) and reorganization (α).
Asokan et al. (2017) argue that robustness (i.e. maintaining some level of functional parts and pathways or the ability to run the system at different output levels, or the ability to cope with errors during execution, according to Olivares Agulia and ElMarapgy (2018)) and transformation (facilitating interactions, expanding capacity and capability when needed) are two parameters that are crucial for a resilient system. Flexibility can lead to both robustness and transformation in times of stress, shock or strain. Further mechanisms for increasing SC resilience have been highlighted by Datta et al. (2007), Hearnshaw and Wilson (2013) and Ma et al. (2014), which include, among others: a hybrid balanced flexibility and redundancy; timely information sharing; agility and responsiveness by regular sensing; visibility; decentralized structure providing autonomy and good coordination; a high level of collaboration with key suppliers, including monitoring their financial and operational health and even working with them to reduce their vulnerabilities; flexibility to produce on demand, based on global and local information, rather than fixed monthly plans; and informed coordinated decision making that constitutes institutional memory and intelligence. According to Birkie et al. (2017), at a higher level of complexity, resilience capabilities lead to more performance benefits when compared with situations with a lower level of complexity.
Evolution. Evolution is related to gradual change or development in complex systems over time. As Gell-Mann (1995, p. 244) highlights, “evolution proceeds by steps, and at each step, complexity can either increase or decrease, but the effect on the whole set of existing species is that the greatest complexity represented has a tendency to grow larger with time.” Accordingly, the capacity of a complex system to interact, learn, adapt and self-organize can change over time. As stated by Bar-Yam (1997, p. 538–539), “the theory of evolution is based upon two processes, mutation and selection, that are assumed to give rise to incremental changes in organisms.” Mutation is related to heritable variations, mainly through changes in the genome from generation to generation, while selection (Lewontin, 1970) is related to differential reproduction.
Building upon these two processes, Abbasi (2014) highlights how SCs can increase probability of their sustainability and gradually developing their behavioral capacities over time by: replicating heredity by, for example, transferring and transforming memory of the generations of the system in time; selecting coopetitively by, for example, letting the subsystems democratically decide, select and constructively compete; having enough variety and diversity by, for example, keeping back-ups from the subsystems, double sourcing, and diversifying the agents, products, processes, and markets.
Pathak et al. (2009) elaborate upon evolutionary role of intelligent agents that dynamically adjust both their overall and local fitness in their surrounding contexts. As Li et al. (2010) put forward, the evolution of complex adaptive supply networks is a function of its structure and fitness. Structure is related to the collection of nodes and edges, and the weight of the edges, whereas fitness is defined as a process of matching the environmental and internal factors. The results of their study show, on the one hand, that government regulations, demand and market structure are the external environmental factors that have the most impact on the evolution of complex adaptive supply networks. On the other hand, firm strategies, product structure complexity, technological complexity and organizational considerations are the predominant internal factors that influence the evolution of complex adaptive supply networks. When analyzing the evolutionary properties and models of SCs, Rose-Anderssen et al. (2009) refer to the method of cladistics in the cases of commercial aerospace SCs, for visualizing evolutionary timelines based on variation in the traits of a SC and the coopetitive selection in the environment. Nair et al. (2009) highlight the reciprocation theory based on the PD (prisoner's dilemma) game and the tit-for-tat strategy (TFT) when discussing the evolution of cooperation between agents. According to Hearnshaw and Wilson (2013), on the one hand, scale-free networks with power-law distributions are likely to improve their fitness and acquisition rate for connections, as are firms that form tightly coupled partnerships with other firms for their exchange strategies. On the other hand, firms that continue to carry out an arms-length transaction will not.
Decision-making properties
As summarized in Table A4 in the Appendix, decision-making properties denote the capacity of multiple-criteria decision making and also of dealing with paradoxical tensions and exogenous effects.
As complex systems are interwoven systems which include interactions in several scales and contexts, decision making by optimizing one parameter can lead to restrictions or conflicts with the other parameters (Serdarasan, 2013; de Leeuw et al., 2013). As a result, decision making in complex systems requires the capacity to semi-optimize the system as a whole when making perfectly isolated decisions. Some parts of sub-systems might attempt to be optimized, however this can lead to the sub-optimal performance of holistic systems. This results from limited and local knowledge as Cilliers (1998, p. 4) states: “each element in the system is ignorant of the behavior of the system as a whole, it responds only to information that is available to it locally. This point is vitally important. If each element ‘knew’ what was happening to the system as a whole, all of the complexity would have to be present in that element.”
The rationale for the extensiveness in complexity theory is based on the notion of paradoxes which are apparent in complex phenomena. Paradoxes can be a state in which two apparently contracting or conflicting, yet interrelated, elements appear to exist simultaneously, neither of which can be eliminated or resolved (Stacey et al., 2000). According to Smith and Lewis (2011), such elements seem logical when considered in isolation, yet irrational, inconsistent and absurd when juxtaposed.
Nilsson and Gammelgaard (2012) reflect upon the rationale for the existence of paradoxes and transformative theology when explaining the properties of complex logistical and SC systems from CAS and complexity thinking (CT) approaches. Some examples of paradoxical properties that may coexist in SCM are: stabilizing price movement with suppliers in order to minimize complexity while increasing manufacturing flexibility to embrace growth in the product portfolio (Turner et al., 2018); coopetition or horizontal collaboration (Nilsson, 2005; Surana et al., 2005); organizational ambidexterity by reconciling ‘exploitation’ (refining and using existing knowledge) and ‘exploration’ (innovation, problem solving and creating new knowledge) (Turner et al., 2018); increase in the self-regulatory survival capacity through an increment in variety, which is also a hindrance to rapid adaptation (Ramirez, 2012) and organizational unity and integrity. Abbasi (2014) furthers the list by highlighting: developing core competency/division of labor/division of perception and knowledge, while being multi- and interdisciplinary/holistic; the centralization of decision-making to increase efficiency as well as encouraging its decentralization to make SCs democratic, resilient and robust; increasing freedom and autonomy for the sake of self-organization and creativity, while establishing restrictions and regulations for the sake of controlling work routines, giving preferences, management and governance, or taking advantage of capabilities that emerge from the bundling of resources.
Exogenous effects. CAS have reflexive relationships with their surrounding natural, business/economic, institutional and socio-political environments or that which is defined as being outside of their boundaries. Changes in the system both shape and are shaped by changes in the surrounding environments. Dynamic interactions between the system and its environment – in addition to dynamic interaction among the subsystems (Bar-Yam, 1997) – take us from issues of simple adaptation and evolution to issues of co-adaptation and co-evolution (Merali, 2006). Schemata of CAS co-adapt and co-evolve with the schemata of their surrounding environments.
Supply and demand for goods and services in the surrounding environments shape the SC systems. However, changes in SC systems, such as launching new products, re-engineering the processes or the emergence of inter-organizational resources reshape existing environments. SC systems dynamically co-adapt with emerging infrastructures, technologies, values, norms, cultural as well as with juridical rules and regulations in their surrounding natural, business/economic, institutional and socio-political environments in different contexts. Experimenting with changes to the degree and diversity of mutual interactions between the CAS and their rugged and dynamic (Capaldo and Giannoccaro, 2015; Choi et al., 2001) or harsh and mild (Pathak et al., 2009) environments can open doors to co-evolution.
Mechanisms for dealing with the complexity of supply chains
With regards the mechanisms for dealing with the complexity of SCs, five mechanisms were inductively conceived from the systematic literature review, namely: classification, measurement, modeling, relational analysis and handling.
Classification mechanisms
As summarized in the former section, almost all of the systematically reviewed literature elaborates on complexity classification by revealing one or two of the properties of the complexity of SCs. However, as illustrated in Tables A1–A4 in the Appendix, structural as well as dynamic properties were discussed much more than behavioral and decision-making properties.
Modeling mechanisms
Table A5 in the Appendix provides an overview of the modeling mechanisms, which can be summarized as: simulation-based, swarm intelligence, network dynamics, evolutionary game theory, mathematical statistics and miscellaneous models.
Simulation-based models were dominant among the systematically reviewed literature and are mainly represented by “agent-based modeling”, which offer promising approaches for understanding the dynamic, behavioral and decision-making properties of complex adaptive SCs by simulating how each agent autonomously makes decisions based on its interactions with the environment and other agents. According to Surana et al. (2005), agent-based modeling is a bottom-up approach which simulates the underlying processes that are believed to be responsible for the global pattern, by enabling the evaluation of which mechanisms are most influential in producing the emergent pattern in question.
Another simulation-based model was “system dynamics”, which is deterministic and does not require multiple iterations (Barbosa and Azevedo, 2017, 2019; Pathak et al., 2007). The drawback of system dynamics models is that the structure has to be determined before starting the simulation (Surana et al., 2005). “Scenario analysis”, “discrete event simulation” and “cellular automata” were also carried out among the systematically reviewed papers in the literature. According to Nair et al. (2009), cellular automata are discrete dynamical systems where space, time and the states of the system are all discrete. Other simulation-based models are based on “evolutionary algorithms”, in particular “genetic algorithms” and “evolving hypergraphs”. Swarm intelligence was represented by the artificial bee colony (ABC), which offer heuristic multi-objective optimization algorithms.
Network dynamics models shed light on the properties of the dynamical patterns of network topologies, such as: the “clustering coefficient” [that expresses network transitivity, which is the average probability of two neighboring nodes that are connected to a given local node being connected to each other], “path length” [the distance between any two nodes chosen at random], “degree distribution” [the average number of connections possessed by each node in the network], “degree of centrality” [which represents the range size of a node control, whereby the higher the degree centrality of a node, the more neighbor nodes are associated with it in the network], “betweenness centrality” [the number of shortest paths from all vertices to all the others that pass through that node] and “network density” [the number of edges of a node, divided by the total possible edges that a node could have].
According to Hearnshaw and Wilson (2013), the key properties of efficient SCs are a high clustering coefficient, a short characteristic path length and a power law connectivity distribution. Two topological models that have particularly been proposed in the systematically reviewed literature were the Watts-Strogatz (WS) model and the Barabási-Albert (BA) model, which have topologies that fall between the regular and the random network models (Surana et al., 2005; Hearnshaw and Wilson, 2013; Ma et al., 2014; Zhang and Liu, 2013; Wang et al., 2018). WS exhibits a high clustering coefficient and the small-world property, i.e. a short characteristic path length, whereas each node has roughly the same number of connections. These findings render WS topology appropriate for establishing the trade-off between reducing transaction costs and improving the decentralized synchronization of SC and efficiently transferring the flows across the system. BA exhibits a low clustering coefficient, a short characteristic path length and power law connectivity distribution (which is known as “scale-free”), all of which indicates the presence of a small number of highly connected nodes or hub nodes, and a large number of nodes with a low number of connections. BA provides improved economic performance, especially regarding a lower transaction cost, over the WS model. BA also improves the synchronization of SCs, as well as the robustness and resilience of the system against the removal of nodes, although it is fragile in respect of the specific removal of the most highly connected nodes. As Statsenko et al. (2018) posit, if a sufficient network density exists, then this is a sign of its responsiveness and adaptability. Inspired from network dynamics, neural networks were highlighted in Pathak et al. (2007) and Surana et al. (2005), as well as in McKelvey et al. (2009).
Evolutionary game theory models investigate the evolutionary dynamics of strategy choices. Mathematical statistics models apply probability theory and statistical models for decision-making support. Originating from strategic and performance management jargon, situation-actors-process (SAP)-learning-action-performance (LAP) or SAP-LAP is a qualitative model that captures the managerial insights and learnings of complex situations and it tends to be dynamic in nature. According to Kavilal et al. (2018) and also Piya et al. (2019), interpretive structural modeling (ISM) is a methodology designed for identifying interdependence among specific items in a complex system by creating a distinguishable hierarchical model, which in turn defines a problem or an issue. Design structure matrix (DSM) is a square matrix which investigates the relationships between the elements of a system. Finally, coupled map lattice (CML) is a branch of dynamical systems, where space and time are discrete and its state is continuous. CML models the behavior of a nonlinear system.
Measurement mechanisms
Measurement mechanisms provide several numerical means for quantifying the complexity of SCs. Entropy-based measures, mathematical optimization or mathematical programming measures, multi-criteria decision-making approaches and indices all emerged from the systematically reviewed literature (see Table A6 in the Appendix).
The first group is constructed based on entropy or Shannon's information entropy, i.e. the amount of information needed to describe or monitor the static/structural or dynamic/operational state of a system, which is a measure of the dimension of variety, disorder and uncertainty. With its origin in operations research, mathematical optimization or mathematical programming provides numerical analytical methods for optimal decision making, mainly by taking the static aspects of the SCs into consideration. Multi-criteria decision-making approaches are represented by the analytic hierarchy process (AHP), and also by the rough set theory (RST) in the systematically reviewed literature. The last group are indices, which mainly provide indicators of SC structural and/or dynamic complexity, inspired by network topology and graph theory.
Relational analysis mechanisms
The fourth group includes those mechanisms that elaborate on the relational analysis between the complexity properties of SCs and different variables, such as flexibility, resilience, responsiveness, adaptability, integration, disruptions, risk, costs, innovation and performance. As summarized in Table A7 in the Appendix, the resultant relational mechanisms can be classified as positive direct relations, negative direct relations, positive moderating relations and negative moderating relations. One of the studies highlights quadratic relations.
Handling mechanisms
The last group highlights mechanisms for handling the complexity of SCs, which can be summarized as mitigating and accommodating strategies (Table A8 in the Appendix). Mitigating strategies highlight the handling of structural and dynamic properties of the complexity of SCs, while accommodating strategies mainly concern the handling of the behavioral and decision-making properties of the complexity of SCs.
Mitigating strategies. The first type of mitigating strategies elaborate on the managing of variety reduction in the following ways by: removing low-volume or low-contribution products from offerings; reducing duplication and redundancy; focusing on a narrower range of elements (e.g. products, suppliers, customers, shipping points, distribution centers, outsourcing partners, geographies); the rationalization of SKUs and modular product architecture or, as Fernández Campos et al. (2019) suggested, by establishing commonalities among the elements, which thus reduces internal diversity while minimizing the effect of this diversity on the extent of the firms' businesses, for instance, by deploying platform teams which seek to define a common internal architecture of processes and tools for SC activities across businesses and geographies.
The second type of mitigating strategies highlights optimal SC configuration by, for example: allocating effective suppliers, partnering firms, or bespoke distribution channels to contain the complexity within a reduced domain, where specialized resources can be leveraged; reducing non-value-added steps and processes; and decoupling practices such as assembly sequence planning and postponement by narrowing the range of activities that must bear structural and dynamic complexity in SCs while preserving firm responsiveness.
The last type of mitigating strategies emphasize exercising tighter control and intervention, which, in turn can include the following: detailed interface management, sub-tier intervention, tight planning and control of many first-tier suppliers, as well as detailed multi-level contracting and risk taking; process standardization and partitioning among the multiple players; tighter integration and relationship management by rigidly systematizing information collection and tangible knowledge management (documentation, organization and storage of business-process information, managerial and group experiences) and by building long-term relationships with key channel partners.
Accommodating strategies. Accommodating strategies are dominant by those that work on managing interrelationships and reciprocal interdependencies, including the following: pay-offs from cooperation; coordination and collaboration among stakeholders, such as suppliers, customers and service providers; decision support through the sharing of information and [both tacit and explicit] knowledge; communication, synchronization and alignment between learning teams, processes and functions, both inside and outside the internal SC; intelligent monitoring, connectivity, clustering, pattern recognition, end-to-end visibility and transparency, by using big data as well as neural network monitoring and smart parts; joint consortiums/alliances and partnerships; and delegated responsivity and risk sharing, such as VMI agreements.
Other accommodating strategies include flexibility and resilience, which highlight the capability of a firm to gain a competitive advantage by quickly realigning its resources and responding faster to unpredictable changes in demand and business model, as well as adjusting to influences of the external environment, regulations and institutions. In this regard, other authors suggest the following: providing a hedge against operational, demand and other environmental uncertainties by building redundancy and buffers, such as extra or adaptive capacity (such as labor, space, machinery, equipment, systems, time, multi-sourcing, outsourcing) and inventory (raw material, semi-finished and finished goods) all of which have the effect of shortening the planning and forecasting horizons; process redesign; periodic batch control; and relying upon flexible workforce, organizational structure and resources.
The last type of accommodating strategies concerns the following: managing by positive feedback; increasing dimensionality, autonomy and improvisation; adopting an ambidextrous approach; co-evolutionary decision making in alignment with contextual conditions at the time; and handling trade-offs. Wilding (1998) and de Leeuw et al. (2013) exemplify trade-offs that exist between having additional buffer stocks in order to reduce uncertainty and increasing costs or demand amplification. As Nilsson and Gammelgaard (2012) state, CAS and CT assume the simultaneous existence of order and unorder, subjectivity, conflicts, power and the emergent indeterminable future of living with, rather than attempting to remove them.
Discussion
Figure 2 illustrates the interrelationship between the emerging dealing mechanisms, which is a continuous process for the classification of complexity in SCs and modeling, measurement, relational analysis and handling over time. Juxtaposing and putting together the potential complexity properties of SCs reveal and explain the clusters of the patterns of their emergent properties without being reductionist. Patterns are dynamic, whilst being subject to evolutionary, revolutionary and exogenous changes over time.
On the one hand, modeling tools can be beneficial for revealing the emergent patterns or collective states of behavior which emerge from the interactions of subsystems over time, while on the other hand, measurement tools can be beneficial as a decision-making support while analyzing emergent patterns. Accordingly, patterns have to be saved in the memory of the system and learned. Pattern learning is a topic that is missing in the mainstream field of SC (complexity) management. Handling complexity goes hand in hand with complexity relational analysis, in order to judge whether mitigating and/or accommodating complexity leads to desired outcomes and creates a foundation for re-classifying complexity over time.
It is very common in different scientific and nonscientific jargons to talk about the necessity of “simplifying” complexity. As explained in the findings section, from a complex systems perspective, complexity is not an unpleasant property that can always be discarded, ignored or oversimplified, but rather it can enable co-evolution with the market or institutional needs as well as the emergence of new properties, such as systemic resilience, robustness and acceptable innovation. Some of the literature refers to this as the “complexity zone” or “edge of chaos”, i.e. a place where components of the complex system never quite lock into place, and yet never quite dissolve into turbulence either, according to Waldrop (1992). The edge of chaos is a constantly shifting battle zone between stagnation and anarchy and is the one place where a complex system can be spontaneous, adaptive and alive.
Among other studies, Dittfeld et al. (2018) for instance refer to this phenomenon when highlighting how one of their case studies within the food processing industry uses differences in demand patterns – associated with high detailed complexity (as a function of numerous and variety) – to achieve better production planning, increased utilization rates and less uncertainty at the plant level. In another study, Birkie et al. (2017) explain that the structural complexity of SCs (as a function of variety and dependencies within system components) is found to have a significant positive relation with performance improvement after disruption, together with resilience capability. When analyzing the trade-offs between structural complexity vs cost and robustness vs cost in a case study setting, Olivares Agulia and ElMarapgy (2018) suggest that complexity (in terms of the number of components and interactions between them) is necessary to achieve robustness and an increase in cost is required to attain a balanced level of complexity and robustness.
Ivanov (2021) elaborates on how some leading firms rely on adaptation strategies (namely, scalability (by expanding network size and capacity), repurposing (by process and product flexibility), substitution (by structural reconfiguration such as usage of backup suppliers, redundancy or product substitution) or intertwining (by fostering collaboration among different economic segments)) to achieve SC viability in responding to the COVID-19 pandemic. The authors provide some insights about how to estimate different thresholds for adaptation investment, preparedness, intensity and impacts, while deploying the different adaptation strategies.
Sharma et al. (2019) conclude that horizontal and vertical complexity (represented by the number of direct suppliers and the number of Tier-2 suppliers per Tier-1 supplier, respectively) in the supply network have a nonlinear relationship with the innovation performance of a firm, which is moderated by a firm's strategic emphasis on value creation (i.e. to find the emphasis on whom) and its influence over the network. Choi and Krause (2006) also propose that although a reduction in complexity may lead to lower transaction costs and increased supplier responsiveness, in certain circumstances this can also increase supply risk and reduce supplier innovation. Therefore, reducing supply base complexity in general could be a cost-efficient approach, although blindly reducing this base could potentially decrease the purchasing company's overall competitiveness.
In contrast to Giannoccaro et al. (2018), who show that the dimensions of complexity (number of firms and level of interrelationships among firms) are negatively related to supply network performance – although moderated by the scope of control –, in their field study and survey of 274 apparel manufacturers and their suppliers in Bangladesh, Chowdhury et al. (2019) propose that SC network complexity (NC) can improve supply chain resilience (SCRE) and, as a result, SC performance (SCP) also, if the NC acts as a buffer in improving flexibility (e.g. by using multiple suppliers, buyers, markets, and alternative transportations which all lead to an increase in flexibility and a reduction in supply risk), as well as a reduction of a SC's vulnerability during supply disruptive events in the SC. Such flexibility in the network leads to higher SCP and, as NC is increased with the increase in SC relational performance (SCRP), the effect of SCRE on SCP is increased. However, as Yang and Yang (2010) investigate, there is a trade-off between redundancy (such as multiple sourcing) and flexibility, where it can be expected that adding redundancy and building flexibility can increase complexity to such a point that an increased exposure of the SC to risk occurs.
As Choi et al. (2001) and Li et al. (2010) posit, when managing supply networks, managers need to appropriately balance how much to control [by deterministically reducing dimensionality and through negative feedback] and how much to allow to emerge [by increasing dimensionality and through positive feedback]. For imposing too much control detracts from innovation and flexibility, and, conversely, too much emergence can undermine managerial predictability and work routines. Giannoccaro et al. (2018) further suggest that scope of control is nonlinearly related to supply network performance, whereby as the scope of control increases, supply network performance initially increases, but then decreases. In other words, the relationship between scope of control and supply network performance follows an inverted-U shape. It is important to bear in mind that complex systems possess behavioral and decision-making properties and that oversimplifying may destroy them, or even produce models that severely misrepresent the original system. In other words, there is a limit to which systems and their control models can be simplified, as these have to satisfy Ashby's law of requisite variety. As Einstein supposedly said when asked how complex a system should be: “everything should be made as simple as possible, but not simpler”.
“Steering”, rather than “controlling” SCs better grasps the evidence of emerging phenomena from the dynamics of interconnected complex systems, as well as gradual/evolutionary, radical/nonlinear/revolutionary and co-evolutionary changes, subjectivity, contextuality and self-organization in managing complex systems. Steering SCs involves adaptability in a dynamically changing environment, where the future is unknown, and is thus semi-predictable, based on trajectories of behavioral patterns. Figure 3 summarizes the learning from this paper regarding the steps for steering SCs as complex systems, which includes defining a complexity profile, classification, measurement, modeling as well as a relational analysis of the complexity properties of SCs and explains how to deal with them over time.
This study also reveals the identification of some research gaps. The authors argue that the science of complexity is relevant and useful for the science of SCM. SCs are not the only social systems which are complex, and that organizational and institutional complexity, that is, the perspective that organizations and institutions are complex systems, is already understood. Therefore, exploring emerging and innovative properties of diverse inter-flows, value-adding processes, tangible as well as intangible organizational resources, ties and relationships all deserve further research from both theoretical and empirical perspectives. Another avenue for further research is the investigation of the co-adaptation and co-evolution of exogenous properties due to governmental, institutional, social and market intervention in different scales and contexts. Once one accepts that SCs are complex systems, it is possible to use achievements in dealing with complex systems from other fields to achieve a better understanding of complex SCs.
Another opportunity for further research is to investigate the effects of structural and dynamical changes (for example, in the aftermath of a pandemic such as COVID-19, or the ripple effect) (Ivanov and Dolgui, 2020, 2021b) on behavioral and decision-making properties and how to model, measure or handle these changes over time.
The above-mentioned classification of the complexity of SCs represents powerful tools for the identification and classification of the agents of agent-based models. Intelligent SCs based on agent-based systems and intelligent flows are powerful tools for accommodating complexity, as are value-adding processes in the system. The analysis and traverse of different parts of intelligent agent-based SCs (such as smart products, IoT (Datta, 2015), cyber-physical systems, digital twins (Datta, 2017; Ivanov and Dolgui, 2021a), robotic and autonomous processes, cognitively intelligent production and distribution systems, clusters of organizations) as well as their application in developing sustainable supply chains would be of great interest.
Conclusion
The main finding in this study is that complexity properties can be summarized in four inter-dependent categories, which we have abbreviated to “SDBD properties”, namely: Structural, Dynamic, Behavioral and Decision making, all of which enable the assessment of SCs as complex systems. The dealing mechanisms framework consists of five steps: classifying complexity, modeling complexity, measuring complexity, relational analysis of complexity and handling desirable/undesirable complexity.
The main contribution of this research is the emergence of the inductive framework for steering SCs from a complex systems perspective, through explaining why SCs have properties of complex systems and suggesting how to deal with their complexity while steering them toward the desired outcomes.
The results suggest that the classification of complexity properties of SCs is a substantial precedent for the modeling, measurement, relational analysis and handling of the complexity of SCs. Complexity classification entails the exposure and assessment of the various components and sub-systems of SCs, which could be complex systems in their own right. Classification also provides knowledge regarding emergent patterns from behaviors and interactions which, in turn, create emergent phenomena which describe not only desirable qualities of the SCs but also the risk of the system.
The inductive framework can be valuable from a pedagogical, research and scientific point-of-view. It has the potential to systematically develop the building blocks of a formal theory (Bryman and Bell, 2007) and to generate a novel perspective or conceptual understanding (Post et al., 2020; Alvesson and Sandberg, 2020; Breslin and Gatrell, 2020) in steering supply chains as complex systems. It could also possibly shift the research paradigm, as well as the classical boundaries of knowledge (Post et al., 2020) in SCM by critically analyzing (Breslin and Gatrell, 2020) and creatively synthesizing (Alvesson and Sandberg, 2020) inter-organizational and institutional insights.
Furthermore, this research – by critically challenging certain taken-for-granted assumptions – can guide both practitioners and decision-makers in understanding why SCs are difficult to manage and control, and subsequently suggest opportunities to steer, or coax them toward the desired outcomes. Recognizing that SCs are complex systems enables a better grasp of the effect of positive feedback on change and transformation, as well as the interactions leading to dynamic equilibria, nonlinearity and the role of learning and innovative capacities, trade-offs and of paradoxical tensions, while also recognizing changing dynamics and the co-evolution of SC phenomena in different scales and contexts.
The limitations of the contribution of this study concerning the methods used include: the choice of database for literature review, the criteria for selection and inclusion and the subjective classification of the literature. While much transparency is in evidence, it is likely that alternative classifications will arise over time with the inclusion of researchers who possess different historical experiences. That is not to say that the above-presented classification is wrong, but rather that there are other alternative valid classifications, as described by Richardson (2004, p. 76) “for complex systems (by which I really mean any part of reality I care to examine) there exists an infinitude of equally valid, non-overlapping, potentially contradictory descriptions.”
In terms of next steps, we propose the testing of the inductive framework, as well as the SDBD properties, which will include case studies in different industries, scales and contexts of SCs. A catalogue of schemata could be developed with contextual information, management interventions and the emergent properties of the SCs over time, which would help build a robust library of SCs patterns and related phenomena, mediated by context and management behaviors.
A further literature review is planned, to include the use of mixed methods to make it possible to also compare and contrast the findings on the complexity of SCs with other types of networks, organization, institutional regime, and market, etc. Both the application of quantitative and qualitative work in network theory on network topology is also of interest, as is research regarding high reliability organizations and regimes for which public policy makes way for diverse interpretation, and, finally, the study of markets in which competition and collaboration are equally desirable.
Figures
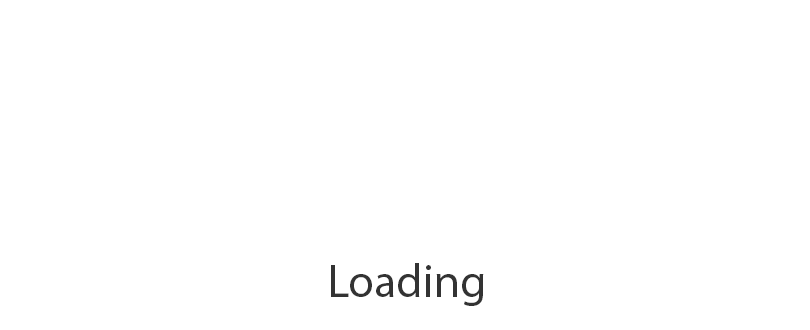
Figure 1
The seven-step model for a comprehensive literature review (CLR) inspired by Onwuegbuzie and Frels (2016)
Structural properties of supply chains complexity
Dynamic properties of supply chains complexity
Behavioral properties of supply chains complexity
Decision-making properties of supply chains complexity
Summary of the reviewed complexity modeling mechanisms
Selective coding: step 3 | Axial coding: step 2 | Open coding: step 1 | Supportive references |
---|---|---|---|
Complexity modeling | Simulation-based | Agent-based modeling | Barbosa and Azevedo (2017, 2019), Bruzzone et al. (2005), Ge et al. (2015), Kim (2009), Mertens et al. (2018), Li et al. (2009, 2010), Zhao et al. (2019), Datta et al. (2007), Nilsson and Darley (2006), McKelvey et al. (2009), Pathak et al. (2007), Surana et al. (2005), Giannoccaro et al. (2018), Giannoccaro (2015), Capaldo and Giannoccaro (2015) |
System dynamics | Barbosa and Azevedo (2017, 2019), Pathak et al. (2007), Surana et al. (2005) | ||
Scenario analysis | Ekinci and Baykasoğlu (2019), Drzymalski (2015) | ||
| Barbosa and Azevedo (2017, 2019), Turner and Williams (2005), Nair et al. (2009), Chen and Lin (2012), Wu et al. (2007), Pathak et al. (2007), Surana et al. (2005) | ||
Evolutionary algorithms
| Suo et al. (2018), Zhang and Liu (2013), Pathak et al. (2007, 2009), Surana et al. (2005), McKelvey et al. (2009) | ||
Swarm intelligence | Propose a multi-objective algorithm for optimization of a complex SC, in regard to simultaneous optimization of cost and lead-time, based on artificial bee colony (ABC) | Jiang et al. (2019a) | |
Present a complex network-oriented artificial bee colony (ABC) algorithm, meta-heuristic approach, by adopting simulated annealing and gradient decent for modeling global bi-objective optimization of cost and lead-time in a three-echelon SC | Jiang et al. (2019b) | ||
Network dynamics |
| Surana et al. (2005), Hearnshaw and Wilson (2013), Ma et al. (2014), Zhang and Liu (2013), Wang et al. (2018), Statsenko et al. (2018), Pathak et al. (2007), McKelvey et al. (2009) | |
Evolutionary game theory | Refers to evolutionary game theory while providing an overview of different modeling mechanisms | Pathak et al. (2007) | |
Propose a price game model in a multi-channel SC with the methods of the stability domain, the bifurcation as well as the maximum Lyapunov exponent diagrams | Ma et al. (2019) | ||
Establish a dynamic nonlinear hybrid SC game model with a service factor | Tu et al. (2019) | ||
Mathematical statistics | Use techniques from multivariate statistics and time series methods, as well as simulation, to capture dynamic interactions between firms in the supply network and the changing configuration, or topology, of the resulting networks that were generated in the total supply network environment in a case study setting of the US automobile industry | Pathak et al. (2009) | |
Refers to statistical physics while providing an overview of different modeling mechanisms | Pathak et al. (2007) | ||
Miscellaneous | Apply a situation-actors-process (SAP)-learning-action-performance (LAP) qualitative model – originated form the strategic and performance management jargons – to understand and analyze the concept of SC complexity drivers and its dynamic behavior in the Indian mining equipment industry | Chand et al. (2018) | |
Use interpretive structural modeling (ISM) to study the interdependence among drivers of SC complexity | Kavilal et al. (2018), Piya et al. (2019) | ||
Use design structure matrix (DSM) to analyze internal relationship between the complexity value, network size and connection probability in ER random networks, small- world networks as well as BA networks | Wang et al. (2018) | ||
Refers to coupled map lattice (CML) as a modeling branch of dynamical systems | Surana et al. (2005) |
Summary of the reviewed complexity measurement mechanisms
Selective coding: step 3 | Axial coding: step 2 | Open coding: step 1 | Supportive references |
---|---|---|---|
Complexity measurement | Entropy-based | Entropy-based measures of static/structural or dynamic/operational states of complexity | Huatuco et al. (2010), Isik (2010), Kriheli and Levner (2018),Nair and Reed-Tsochas (2019),Cheng et al. (2014), Olivares Agulia and ElMarapgy (2018),Rodewald et al. (2016), Hamta et al. (2018),Modrak and Marton (2014), Sivadasan et al. (2006) |
Mathematical optimization or mathematical programming | Propose assembly sequence planning and optimal assembly SC configuration as two measures of assembly systems and SCs complexity | Hu et al. (2008) | |
Demonstrate how the optimal assembly supply network is obtained by comparing the total complexity values of the feasible configurations | Hamta et al. (2018) | ||
In a case study setting, rely upon mathematical programming to find the optimal product mix for the entire value chain such that the contribution margin (CM) is maximized. In a second step, another nonlinear mathematical programming model (referred to as cost allocation model (CAM)) is used to allocate the costs of the optimized product mix to the individual products | Klosterhalfen et al. (2019) | ||
Refer to Hamiltonian-based optimization as well as reliability-based design optimization methods | Pathak et al. (2007), Surana et al. (2005) | ||
Multi-criteria decision-making | Apply AHP for measuring macro-institutional environmental complexity surrounding global SC operations | Kinra and Kotzab (2008) | |
Rely upon AHP to quantify and prioritize drivers of SC complexity for managerial decision making | Chand et al. (2018) | ||
Put forward rough set theory (RST) approach as a tool for evaluating and analyzing sustainable SC complexity | Bai and Sarkis (2018) | ||
Indices | Present five indices of SC complexity driven from graph theory, namely size index (referring to the number/numerousness of nodes or entities in the SC and the interrelations between them); density index (defined as the ratio of the number of edges to the theoretical number of potential edges); cycles index (loops of nodes that start and end at the same node. Cycles in SCs are interpreted as outsourcing entities that need to perform an activity over the flow of materials and return it to the sender […] cycle index is calculated as the ratio between the number of cycles and the theoretical maximum number of cycles); paths index (considers the number of paths in the SC and compares it with the minimum theoretical number of paths […] SC complexity increases as the number of possible routes in the SC increases); and decision points index (characterizes the cumulative complexity of decision-making as a function of number of the nodes in a path […] as the decisions to be made for each supplier increase, the potential for more errors exists) | Olivares Agulia and ElMarapgy (2018) | |
Propose a graph-theoretic approach (GTA) to quantify SC complexity by a single numerical index considering the interdependence and the inheritance of the SC complexity drivers, namely, numerousness, variability, diversity, lack of visibility and uncertainty. GTA synthesizes the interrelationship among different variables or sub-system variables and provides a synthetic score for the entire system | Kavilal et al. (2018) | ||
Reflect upon indices related to average path length representing the number of edges in the shortest path connecting two nodes in the network; average clustering coefficient referring to the degree of mutual exchange between nodes in the network; and degree of a node referring to the number of edges connecting the node in the network | Chen and Lin (2012) | ||
Elaborate upon density of ties as a measure of complexity in a network | Nair and Reed-Tsochas (2019) | ||
In an identical study, provide an index of SC complexity as a function of the weight of connections among the nodes | Caridi et al. (2010) | ||
Point out three indicators for SC complexity: flow complexity metrics (FC) which assume a positive, linear relationship between tiers, nodes, links and complexity; restiveness estimator (RT) based on the number of feasible sequences in a graph; as well as aggregate indicator of process complexity (AC) based on integration of three sub-indicators, namely, binding of structure, diameter of network and structure diversity | Modrak and Semanco (2011) | ||
Presents three measures of value chain complexity: the number of parts in a final application, the number of parts affected by a material change in a final application and the difficulty of changing the value chain of a final application | Musso (2009) | ||
Illustrate three indicators for complexity (topological properties) of assembly SCs as typical representatives of convergent SCs: an indicator composed of number of links and tiers [for a case when dominant demand exists]; an indicator of total structure complexity composed of sum of local module degrees [for a case when dominant demand does not exist]; and an entropy-based index of configuration complexity of assembly SCs [for a case when assembly SCs are considered as general networks] | Modrak and Marton (2014) | ||
Propose a unified measure of complexity by integrating both product variety and assembly process information | Hu et al. (2008) | ||
Present a position index to determine the overall complexity of the supply network, within a multiple case study in the Austrian agricultural industry, based on contribution of five generic core parameters, namely number of elements, number of interrelationships, degree of uncertainty, customer-driven product variety and geographical components | Gerschberger et al. (2012) | ||
In a multiple case study, offer an operational complexity index in make-to-stock as well as make-to-order settings by measuring variety and uncertainty coming from the customer and the supplier, goodness of the planning and scheduling adaptable to the uncertainty, and level of flexibility of the process which restricts the planning or scheduling in dealing with the variety and uncertainty | Wu et al. (2007) | ||
In a wholesale setting, illustrate complexity scores for measuring eight identified drivers of SC complexity, namely, uncertainty, diversity, size, variability, structure, speed, lack of information synchronization and lack of cooperation | de Leeuw et al. (2013) | ||
Present a measure of SC complexity value by combing SC strength (as a function of the quantity of arcs and their weights, and the number of virtual arcs) and SC clustering (denseness or connectivity of the network […] define SC clustering coefficient as the ratio of the number of total upstream and downstream suppliers a node is connected with to the total number of possible up and downstream suppliers, regardless of whether they are physical or virtual arcs) | Drzymalski (2015) | ||
Propose a measure of SC network complexity as a function of the sum of the complexity values of the SC network node enterprises, the sum of the complexity values of the associated edges of the node enterprises in the SC network and the complexity values generated by the topological structures between SC network nodes, i.e. the complexity of the network topology. The measure is then applied to the complexity evolution analysis of ER stochastic network, WS small-world network, NW small-world network and BA scale-free network | Wang et al. (2018) |
Summary of the reviewed complexity relational analysis mechanisms
Selective coding: step 3 | Axial coding: step 2 | Open coding: step 1 | Supportive references |
---|---|---|---|
Complexity analysis | Positive direct relations | Positive direct relationships among horizontal, vertical and spatial SC complexity and their synergies with frequency of SC disruptions | Bode and Wagner (2015) |
SC structural complexity is found to have a significant positive relation with performance improvement after disruption, along with resilience capability | Birkie et al. (2017) | ||
Direct relation between supply chain complexity and urban controlled energy footprint (CEF) in Beijing | Chen et al. (2019) | ||
Higher supplier-induced complexity is positively related to the number of adverse outcomes (particularly measured as the number of complaints related to a particular supplier) in a supply chain | Gerschberger et al. (2017) | ||
Significant and positive relationship between product complexity and vertical integration in a case study setting of luxury-performance segment of the auto industry | Novak and Eppinger (2001) | ||
The following supply chain characteristics increase supply chain decision-making complexity: the number of items, bottleneck items, the extent of global operations, the number of production facilities and the extension of lead times and planning horizons | Asmussen et al. (2018) | ||
More complex the product (in terms of the number of components), the higher the risk of potential supply chain disruption | Inman and Blumenfeld (2014) | ||
Positive relationship between operational complexity and SC costs in a make-to-stock case of supplier-customer systems in UK | Wu et al. (2007) | ||
Positive relation between SC structural complexity and complexity of information being shared within the SC | Posey and Bari (2009) | ||
Positive relationship between supply base complexity and transaction cost | Choi and Krause (2006) | ||
Nonlinear relationship between both horizontal complexity and vertical complexity with respect to innovation performance | Sharma et al. (2019) | ||
Under high product complexity, firms tend to implement internal and supplier integration, while product complexity does not have a direct impact on customer integration | Shou et al. (2017) | ||
Negative direct relations | Negative relation between plant-level performance (i.e. plant schedule attainment and manufacturing costs) and upstream complexity, internal manufacturing complexity as well as downstream complexity | Bozarth et al. (2009) | |
Cost estimation accuracy is negatively affected by supply chain decision-making complexity | Asmussen et al. (2018) | ||
Increased value chain complexity leads to longer adoption delay, i.e. adoption time of materials and likely other upstream products in the plastics industry | Musso (2009) | ||
Dimensions of complexity (number of firms and level of interrelationships among firms) are negatively related to supply network performance, but the extent of performance decrease depends on the scope of control exerted by the focal company in the supply network | Giannoccaro et al. (2018) | ||
Direct negative relationship between uncertainty dimension of SC complexity and delivery performance | Milgate (2001) | ||
Negative relationship between delivery performance and the level of process-product complicatedness as well as management system uncertainty | Vachon and Klassen (2002) | ||
Negative relationship between supply base complexity and supplier responsiveness | Choi and Krause (2006) | ||
Degree of tight coupling in a supply network is negatively related to network complexity. Moreover, supply network complexity is negatively related to traceability and transparency | Skilton and Robinson (2009) | ||
Negative relation between spatial complexity and innovation performance | Sharma et al. (2019) | ||
Positive moderating relations | Positive moderating effect of product complexity on the link between SC adaptability and cost performance as well as operational performance | Eckstein et al. (2015) | |
Moderating effect of product and market complexity on the relationship of SC information integration with financial and operational performance outcomes | Wong et al. (2015) | ||
SC structural complexity positively moderates the resilience–performance link | Birkie et al. (2017) | ||
Supply chain relational performance (SCRP) [in terms of an increase in trust, cooperation, and commitment] and network complexity (NC) [as a function of the number of nodes in the network, interconnections between the nodes and the geographical spread of the network] individually, as well as their interaction, positively moderate the link between supply chain resilience (SCRE) [which consists of flexibility, redundancy, visibility and collaboration dimensions] and supply chain performance (SCP) … As NC increased with the increase in SCRP, the effect of SCRE on SCP increased | Chowdhury et al. (2019) | ||
Relationship between SC integration and performance is positively moderated by supply complexity | Gimenez et al. (2012) | ||
SC type influences the relationship between learning and adaptation and that the product complexity positively moderates this effect | Giannoccaro (2015) | ||
Relationship between SC structural complexity and information complexity is positively moderated by the amount of shared information | Posey and Bari (2009) | ||
A firm's strategic emphasis on value creation and its influence moderate the link between the complexity dimensions (horizontal, vertical, spatial) and innovation performance | Sharma et al. (2019) | ||
Negative moderating relations | Negative moderating effect of supply complexity on the relationship between internal knowledge transfer and SC flexibility as well as negative moderating effect of product complexity on the relationship between external knowledge transfer and SC flexibility | Blome et al. (2014) | |
SC type influences the relationship between learning and adaptation and that the turbulence of the environment negatively moderate this effect | Giannoccaro (2015) | ||
Product complexity has negative moderating impact on the relationship between SC integration and SC performance (in terms of innovation and flexibility) | Caniato and Größler (2015) | ||
Quadratic relations | Propose: (1) positive quadratic relationship between supply base complexity and supply risk, (2) negative quadratic relationship between supply base complexity and supplier innovation | Choi and Krause (2006) |
Summary of the reviewed complexity handling mechanisms
Selective coding: step 3 | Axial coding: step 2 | Open coding: step 1 | Supportive references |
---|---|---|---|
Complexity handling | Mitigating strategies | Adopting “variety reducing management practices” to physically reduce the level of structural complexity in two ways: The first is by aiding the firm to focus on a narrower range of elements (e.g. products, customers, geographies, etc.) via rationalization exercises such as rationalization of SKUs; The second is by establishing commonalities among these elements, thus reducing internal diversity while minimizing the effect of this on the breadth of the firms' businesses (for instance, by deploying platform teams that seek to define a common internal architecture of processes and tools for supply chain activities across businesses and geographies) | Fernández Campos et al. (2019) |
Elaborate upon strategic and human cognitive ability moderators – that may moderate the impact of SC decision-making complexity – including: scope and boundary management (by limiting the number of SKUs or shipping points, tightening the processes partitioning among the multiple players); tighter integration and relationship management (by reducing duplication and redundancy, building long-term relationships with key channel partners); reducing non-value-added steps and processes; rigidly systematizing information integration (by collection of relevant available technologies and skilled staff); tangible knowledge management (documentation, organization and storage business-process information, managerial and group experiences); fostering human cognitive processes gained through natural intellect, experience, training and consultancy | Manuj and Sahin (2011) | ||
Suggest strategies for reducing the unnecessary complexity by, for example, reducing the number of products, the options in the product and the SKUs (product complexity), the number of outsourcing partners, the number of distribution centers; standardization of operations; process automation | Serdarasan (2013) | ||
Identify mechanisms used by wholesalers for mitigating SC complexity: keeping inventory, rationalization both in terms of product and in terms of customers and suppliers | de Leeuw et al. (2013) | ||
Highlight actions taken by the study firm to reduce supply chain complexity: removing low-volume, low-contribution products from offerings; three-day frozen production | Aitken et al. (2016) | ||
Modular product architecture | Shamsuzzoha (2018), Olivares Agulia and ElMarapgy (2018), Choi et al. (2001) | ||
Supply chain design and development by optimal supply chain configuration, identifying the right suppliers or partnering firms, based on appropriate criteria | Gunasekaran et al. (2015) | ||
Reducing buffer within the system as well as interactive complexity, i.e. ways that parts within a system are connected and interact; Postponement | Yang and Yang (2010) | ||
Confinement and decoupling practices by focusing on the dependencies and relations between elements in the system: Confinement practices (e.g. outsourcing, customized distribution channels) aim to contain complexity within a reduced domain, where specialized resources can be leveraged; Decoupling seeks to lessen the relations and constraints between elements and to render certain parts of the system more independent from others (e.g. postponement, stocks) | Fernández Campos et al. (2019) | ||
Propose below measures for mitigating complexity of assembly systems and supply chains: (1) assembly sequence planning; (2) optimal assembly supply chain configuration | Hu et al. (2008) | ||
Planning and control | Turner et al. (2018), Giannoccaro et al. (2018) | ||
Highlight “control and intervention strategy” as a generic strategy for managing offshore wind power supply network complexity with following characteristics: tight control and detailed interface management; sub-tier intervention, engage with many 1st tier suppliers; detailed multi and multi-level contracting; interface risks on complex projects, risk taking; seek optimization of a portfolio of projects, project factory | Johnsen et al. (2019) | ||
Accommodating strategies | Relationship development | Turner et al. (2018) | |
Working collaboratively with suppliers, joint consortiums/alliances | Sayed et al. (2017) | ||
Coordination and collaboration practices, i.e. managerial approaches or systems that foster sharing knowledge and solutions in the supply chain as well as the synchronization and alignment between teams and functions inside and outside the internal supply chain … These practices do not reduce extant complexity, but rather make the supply chain more capable of coping with it by allowing managers to understand the effects of their actions outside their domain and facilitating for complexity to be managed more holistically rather than from within silos | Fernández Campos et al. (2019) | ||
Highlight “coordination and delegation strategy” as a generic strategy for managing offshore wind power supply network complexity with following characteristics: delegated responsibility and systems sourcing; engage with few first-tier suppliers; few contracts, mainly with first tier suppliers; risk sharing opportunistic approach to project selection – no pipeline | Johnsen et al. (2019) | ||
Managing interdependencies; specifically, the reciprocal interdependency, involving simultaneous, ongoing relationships with multiple linkages among firms in supply network. The results suggest that depending on the nature of the interdependence, incentive schemes and governance structures should be set up in supply chain relationships that aid in the formation of cooperative relationships throughout the network | Nair et al. (2009) | ||
Suggest integrating neural network monitoring with smart parts autonomous supply networks to manage their vulnerability to the nonlinear and extreme dynamics found in CAS within the business world | Wycisk et al. (2008) | ||
Decision support and knowledge generation practices are equally employed to accommodate complexity. These practices increase the supply chain's ability to cope with extant complexity by enhancing decision making at (generally) the individual manager level. In order to do so, they aid managers partially surpass their cognitive limitations in dealing with complexity in various ways. Some practices allow managers to filter out and focus on a reduced number of decisions and elements (e.g. automation, vendor rating tools, anti-mixing systems) | Fernández Campos et al. (2019) | ||
Elaborate upon tactical moderators – that may moderate undesirable outcomes of SC decision-making complexity – by increasing system flexibility including: building buffers (such as extra capacity (labor, space, machinery, equipment, systems, time, multi sourcing, etc.) or inventory (raw material, semi-finished and finished goods) which provide a hedge against operational, demand and other environmental uncertainties); flexible workforce | Manuj and Sahin (2011) | ||
Suggest strategies for managing/adjusting to the necessary complexity by, for example supply chain integration; collaboration with suppliers, customers and service providers; supply chain visibility; synchronization of data; information sharing; logistics outsourcing; planning on a daily basis; process improvement and redesign | Serdarasan (2013) | ||
Identify mechanisms used by wholesalers for accommodating SC complexity: flexibility of resources; information exchange and communication; outsourced activities; developing a separate unit for dealing with complicated activities. Furthermore, highlight that managing complexity requires trade-offs … for example, while investment in additional inventory may reduce complexity related to uncertainty, it will increase costs and assets and it requires appropriate capabilities to manage inventory … companies may choose to reduce complexity by reducing their supplier base, yet this may lead to an increase in supply risks | de Leeuw et al. (2013) | ||
Highlight actions taken by the study firm to absorb supply chain complexity: separate demand planning for MTS and MTO; focused packaging lines; direct interaction between production and marketing/MTO customers; VMI agreement that provided direct visibility into plant inventory levels (IP enhancement) | Aitken et al. (2016) | ||
Developing CASN resilience by entity resilience, utilization of local relief capacity, entity diversity, adaptive entity capacity, clustering and connectivity, path length and redundancy, topology dynamics and trust making | Day (2014) | ||
Flexibility and resilience | Turner et al. (2018), Asokan et al. (2017), Yang and Yang (2010), Birkie et al. (2017) | ||
Offers some recommendations for dealing with SC complexity such as: dealing with uncertainty by buffering inventory while recognizing the trade-off between increased inventory and demand amplification; reducing demand amplification by JIT, vendor integration and time-based management; short-term forecasts and prediction of patterns; short-term strategic management; diminishing chaotic uncertainty by running the planning system with a period of less than the prediction horizon; periodic batch control (PBC) | Wilding (1998) | ||
Propose two types of adaptive strategies, namely reactive and proactive strategies, a firm can leverage to restructure a supply chain network and accordingly reduce the negative effects of supply chain network disruptions: Reactive strategies restructure the network in response to a disruption event among first-tier suppliers. In other words, reactive strategies are used when an immediate supplier of a focal firm fails; Proactive strategies are used when a distant (beyond first tier) disruption is observed but has not yet hit the focal firm | Zhao et al. (2019) | ||
| Gunasekaran et al. (2015) | ||
The emergent patterns in a supply network can much better be managed through positive feedback, which allows for autonomous action | Choi et al. (2001) | ||
Handling trade-offs | Sayed et al. (2017), Yang and Yang (2010), Olivares Agulia and ElMarapgy (2018) | ||
Ambidextrous approach | Turner et al. (2018) | ||
The results suggest that decisions and choices in supply chain management should be aligned to contextual conditions instead of being exclusively based on “best practices” that would be principles of general applicability | Wanke and Corrêa (2014) | ||
Complex adaptive systems (CAS) and complexity thinking (CT) assume simultaneous existence of order and unorder, subjectivity, conflicts, power, an emergent indeterminable future to live with instead of trying to remove it | Nilsson and Gammelgaard (2012) | ||
Identify 14 management implications addressing environmental factors for the commercial aerospace manufacturing sector including: globalization (access to skills for innovation, low-cost manufacturing, access to global markets), product complexity miniaturization (specialization, consolidation, systematization), standard interfaces, technology, quality standards, innovation, market responsiveness, information sharing, supplier performance, risk mitigation | Varga et al. (2009) | ||
Delve into the minds of operations managers, who must carry out an interpretation process to set goals, process information, perceive their environment and actively solve problems when confronted with the organization's decision to implement supply chain initiatives | Omar et al. (2012) | ||
| Li et al. (2010) |
References (Narrative literature review (NLR))
Abbasi, M. (2014), Exploring Themes and Challenges in Developing Sustainable Supply Chains: A Complexity Theory Perspective, Media-Tryck AB, Lund.
Allen, P.M. and Strathern, M. (2003), “Evolution, emergence, and learning in complex systems”, Emergence, Vol. 5, pp. 8-33.
Alvesson, M. and Sandberg, J. (2020), “The problematizing review: a counterpoint to Elsbach and Van Knippenberg's argument for integrative reviews”, Journal of Management Studies, Vol. 57, pp. 1290-1304.
Ambrosini, V. and Bowman, C. (2009), “What are dynamic capabilities and are they a useful construct in strategic management?”, International Journal of Management Reviews, Vol. 11, pp. 29-49.
Asthana, S., Gradinger, F., Elston, J., Martin, S. and Byng, R. (2020), “Capturing the role of context in complex system change: an application of the Canadian context and capabilities for integrating care (CCIC) framework to an integrated care organisation in the UK”, International Journal of Integrated Care, Vol. 20, pp. 1-10.
Bar-Yam, Y. (1997), Dynamics of Complex Systems, Perseus Books, Reading, Massachusetts.
Bowman, C. and Collier, N. (2006), “A contingency approach to resource-creation processes”, International Journal of Management Reviews, Vol. 8, pp. 191-211.
Breslin, D. and Gatrell, C. (2020), “Theorizing through literature reviews: the miner-prospector continuum”, Organizational Research Methods. doi: 10.1177/1094428120943288.
Bryman, A. and Bell, E. (2007), Business Research Methods, Oxford University Press, New York.
Casti, J. (1994), Complexification: Explaining a Paradoxical World through the Science of Surprise, Harper Collins Publishers, New York.
Chiva, R., Grandío, A. and Alegre, J. (2010), “Adaptive and generative learning: implications from complexity theories: adaptive and generative learning”, International Journal of Management Reviews, Vol. 12, pp. 114-129.
Christopher, M. (2005), Logistics and Supply Chain Management: Creating Value-Adding Networks, Prentice-Hall, London.
Cilliers, P. (1998), Complexity and Postmodernism: Understanding Complex Systems, Routledge, London.
Cilliers, P. (2005), “Complexity, deconstruction and relativism”, Theory, Culture and Society, Vol. 22, pp. 255-267.
Datta, S.P.A. (2015), “Dynamic socio-economic disequilibrium catalyzed by the internet of things”, Journal of Innovation Management, Vol. 3, pp. 4-9.
Datta, S.P.A. (2017), “Emergence of digital twins – is this the march of reason?”, Journal of Innovation Management, Vol. 5, pp. 14-33.
Dobrzykowski, D., Leuschner, R., Hong, P. and Roh, J. (2015), “Examining absorptive capacity in supply chains: linking responsive strategy and firm performance”, The Journal of Supply Chain Management, Vol. 51, pp. 3-28.
Drake, M.J. and Schlachter, J.T. (2008), “A virtue-ethics analysis of supply chain collaboration”, Journal of Business Ethics, Vol. 82, pp. 851-864.
Dubey, R., Gunasekaran, A., Childe, S.J., Papadopoulos, T., Luo, Z. and Roubaud, D. (2020), “Upstream supply chain visibility and complexity effect on focal company's sustainable performance: Indian manufacturers' perspective”, Annals of Operations Research, Vol. 290, pp. 343-367.
Fisher, M.L. (1997), “What is the right supply chain for your product?”, Harvard Business Review, Vol. 75, pp. 105-116.
Gell-Mann, M. (1995), The Quark and the Jaguar: Adventures in the Simple and the Complex, W. H. Freeman, New York.
Gershenson, C. (2007), Design and Control of Self-Organizing Systems, Vrije Universiteit, Brussel.
Gershenson, C. and Heylighen, F. (2004), How Can We Think the Complex?, Centrum Leo Apostel Vrije Universiteit, Brussel.
Greer, C. and Lei, D. (2012), “Collaborative innovation with customers: a review of the literature and suggestions for future research”, International Journal of Management Reviews, Vol. 14, pp. 63-84.
Hogue, M. and Lord, R.G. (2007), “A multilevel, complexity theory approach to understanding gender bias in leadership”, The Leadership Quarterly, Vol. 18, pp. 370-390.
Holland, J.H. (1992), Adaptation in Natural and Artificial Systems: An Introductory Analysis with Applications to Biology, Control, and Artificial Intelligence, MIT Press, Cambridge.
Holland, J.H. (1995), Hidden Order: How Adaptation Builds Complexity, Addison-Wesley, Reading, Massachusetts.
Hoogeboom, M.A.M. and Wilderom, C.P. (2020), “A complex adaptive systems approach to real-life team interaction patterns, task context, information sharing, and effectiveness”, Group and Organization Management, Vol. 45, pp. 3-42.
Huggins, R. (2010), “Forms of network resource: knowledge access and the role of inter-firm networks”, International Journal of Management Reviews, Vol. 12, pp. 335-352.
Ivanov, D. (2021), “Supply chain viability and the COVID-19 pandemic: a conceptual and formal generalisation of four major adaptation strategies”, International Journal of Production Research, Vol. 59, pp. 3535-3552.
Ivanov, D. and Dolgui, A. (2020), “Viability of intertwined supply networks: extending the supply chain resilience angles towards survivability. A position paper motivated by COVID-19 outbreak”, International Journal of Production Research, Vol. 58, pp. 2904-2915.
Ivanov, D. and Dolgui, A. (2021a), “A digital supply chain twin for managing the disruption risks and resilience in the era of Industry 4.0”, Production Planning and Control, Vol. 32, pp. 775-788.
Ivanov, D. and Dolgui, A. (2021b), “OR-methods for coping with the ripple effect in supply chains during COVID-19 pandemic: managerial insights and research implications”, International Journal of Production Economics, Vol. 232, 107921.
Kauffman, S. (1995), At Home in the Universe: The Search for Laws of Self-Organization and Complexity, Oxford University Press, Oxford.
Lambert, D. (2006), Supply Chain Management: Processes, Partnerships, Performance, The Hartley Press, Florida.
Larentis, F., Antonello, C. and Slongo, L. (2019), Inter-Organizational Culture Linking Relationship Marketing with Organizational Behavior, Springer International Publishing, Cham.
Letiche, H. (2000), “Phenomenal complexity theory as informed by Bergson”, Journal of Organizational Change Management, Vol. 13, pp. 545-557.
Lewontin, R.C. (1970), “The units of selection”, Annual Review of Ecology and Systematics, Vol. 1, pp. 1-18.
Mason, M. (2009), “Making educational development and change sustainable: insights from complexity theory”, International Journal of Educational Development, Vol. 29, pp. 117-124.
McMillan, E. (2006), Complexity, Organizations and Change – An Essential Introduction, Routledge, London, New York.
Mentzer, J.T., DeWitt, W., Keebler, J.S., Min, S., Nix, N.W., Smith, C.D. and Zacharia, Z.G. (2001), “Defining supply chain management”, Journal of Business Logistics, Vol. 22, pp. 1-25.
Merali, Y. (2006), “Complexity and information systems: the emergent domain”, Journal of Information Technology, Vol. 21, pp. 216-228.
Niesten, E. and Stefan, I. (2019), “Embracing the paradox of interorganizational value co-creation–value capture: a literature review towards paradox resolution”, International Journal of Management Reviews, Vol. 21, pp. 231-255.
Nilsson, F. (2005), Adaptive Logistics – Using Complexity Theory to Facilitate Increased Effectiveness in Logistics, MediaTryck AB, Lund.
Ojha, D., Acharya, C. and Cooper, D. (2018), “Transformational leadership and supply chain ambidexterity: mediating role of supply chain organizational learning and moderating role of uncertainty”, International Journal of Production Economics, Vol. 197, pp. 215-231.
Olavarrieta, S. and Ellinger, A.E. (1997), “Resource-based theory and strategic logistics research”, International Journal of Physical Distribution and Logistics Management, Vol. 27, pp. 559-587.
Onwuegbuzie, A.J. and Frels, R. (2016), 7 Steps to a Comprehensive Literature Review : a Multimodal & Cultural Approach, SAGE, Los Angeles.
Ouyang, Y. and Li, X. (2010), “The bullwhip effect in supply chain networks”, European Journal of Operational Research, Vol. 201, pp. 799-810.
Post, C., Sarala, R., Gatrell, C. and Prescott, J.E. (2020), “Advancing theory with review articles”, Journal of Management Studies, Vol. 57, pp. 351-376.
Ramirez, G.A. (2012), “Sustainable development: paradoxes, misunderstandings and learning organizations”, The Learning Organization, Vol. 19, pp. 58-76.
Reitsma, F. (2001), “A response to simplifying complexity”, Geoforum, Vol. 34, pp. 13-15.
Richardson, K.A. (2004), “Systems theory and complexity: part 1”, Emergence: Complexity and Organization, Vol. 6, pp. 75-79.
Roldán Bravo, M., Stevenson, M., Moreno, A. and Lloréns Montes, F. (2020), “Absorptive and desorptive capacity configurations in supply chains: an inverted U-shaped relationship”, International Journal of Production Research, Vol. 58, pp. 2036-2053.
Rose-Anderssen, C., Baldwin, J.S., Ridgway, K., Allen, P.M., Varga, L. and Strathern, M. (2009), “A cladistic classification of commercial aerospace supply chain evolution”, Journal of Manufacturing Technology Management, Vol. 20, pp. 235-257.
Saunders, M., Lewis, P. and Thornhill, A. (2009), Research Methods for Business Students, Prentice-Hall, Harlow.
Simchi-Levi, D., Kaminsky, P. and Simchi-Levi, E. (2004), Managing the Supply Chain the Definitive Guide for the Business Professional, McGraw-Hill, New York.
Smith, W.K. and Lewis, M.K. (2011), “Toward a theory of paradox: a dynamic equilibrium model of organizing”, Academy of Management Review, Vol. 36, pp. 381-403.
Stacey, R.D., Griffin, D. and Shaw, P. (2000), Complexity and Management – Fad or Radical Challenge to Systems Thinking?, Routledge, London.
Stock, J.R. and Boyer, S.L. (2009), “Developing a consensus definition of supply chain management: a qualitative study”, International Journal of Physical Distribution and Logistics Management, Vol. 39, pp. 690-711.
Strauss, A. and Corbin, J.M. (1990), Basics of Qualitative Research: Grounded Theory Procedures and Techniques, Sage, Newbury Park, California.
Strauss, A. and Corbin, J.M. (1998), Basics of Qualitative Research: Techniques and Procedures for Developing Grounded Theory, Sage, Thousand Oaks, California.
Turner, N., Swart, J. and Maylor, H. (2013), “Mechanisms for managing ambidexterity: a review and research agenda”, International Journal of Management Reviews, Vol. 15, pp. 317-332.
van Fenema, P.C. and Keers, B. (2018), “Interorganizational performance management: a co-evolutionary model: interorganizational performance management”, International Journal of Management Reviews, Vol. 20, pp. 772-799.
Vogel, R. and Güttel, W. (2013), “The dynamic capability view in strategic management: a bibliometric review”, International Journal of Management Reviews, Vol. 15, pp. 426-446.
Waldrop, M.M. (1992), Complexity: The Emerging Science at the Edge of Order and Chaos, Simon and Schuster, New York.
Young, O.R. (2012), Navigating the Sustainability Transition: Governing Complex and Dynamic Socio-Ecological Systems, Oxford University Press, Oxford.
References (Systematic literature review (SLR))
Aitken, J., Bozarth, C. and Garn, W. (2016), “To eliminate or absorb supply chain complexity: a conceptual model and case study”, Supply Chain Management, Vol. 21, pp. 759-774.
Asmussen, J.N., Kristensen, J. and Wæhrens, B. (2018), “Cost estimation accuracy in supply chain design: the role of decision-making complexity and management attention”, International Journal of Physical Distribution and Logistics Management, Vol. 48, pp. 995-1019.
Asokan, V.A., Yarime, M. and Esteban, M. (2017), “Introducing flexibility to complex, resilient socio-ecological systems: a comparative analysis of economics, flexible manufacturing systems, evolutionary biology, and supply chain management”, Sustainability, Vol. 9, pp. 1-17.
Bai, C. and Sarkis, J. (2018), “Honoring complexity in sustainable supply chain research: a rough set theoretic approach (SI:ResMeth)”, Production Planning and Control: Rethinking Research Methods in Operations and Supply Chain Management, Vol. 29, pp. 1367-1384.
Barbosa, C. and Azevedo, A. (2017), “Hybrid simulation for complex manufacturing value-chain environments”, Procedia Manufacturing, Vol. 11, pp. 1404-1412.
Barbosa, C. and Azevedo, A. (2019), “Assessing the impact of performance determinants in complex MTO/ETO supply chains through an extended hybrid modelling approach”, International Journal of Production Research, Vol. 57, pp. 3577-3597.
Birkie, S.E., Trucco, P. and Fernandez Campos, P. (2017), “Effectiveness of resilience capabilities in mitigating disruptions: leveraging on supply chain structural complexity”, Supply Chain Management: An International Journal, Vol. 22, pp. 506-521.
Blome, C., Schoenherr, T. and Eckstein, D. (2014), “The impact of knowledge transfer and complexity on supply chain flexibility: a knowledge-based view”, International Journal of Production Economics, Vol. 147, pp. 307-316.
Bode, C. and Wagner, S.M. (2015), “Structural drivers of upstream supply chain complexity and the frequency of supply chain disruptions”, Journal of Operations Management, Vol. 36, pp. 215-228.
Bozarth, C.C., Warsing, D.P., Flynn, B.B. and Flynn, E.J. (2009), “The impact of supply chain complexity on manufacturing plant performance”, Journal of Operations Management, Vol. 27, pp. 78-93.
Bruzzone, A., Mosca, R., Revetria, R., Bocca, E. and Briano, E. (2005), “Agent directed HLA simulation for complex supply chain modeling”, Simulation, Vol. 81, pp. 647-655.
Caniato, F. and Größler, A. (2015), “The moderating effect of product complexity on new product development and supply chain management integration”, Production Planning and Control, Vol. 26, pp. 1306-1317.
Capaldo, A. and Giannoccaro, I. (2015), “Interdependence and network-level trust in supply chain networks: a computational study”, Industrial Marketing Management, Vol. 44, pp. 180-195.
Caridi, M., Crippa, L., Perego, A., Sianesi, A. and Tumino, A. (2010), “Do virtuality and complexity affect supply chain visibility?”, International Journal of Production Economics, Vol. 127, pp. 372-383.
Chand, P., Thakkar, J. and Ghosh, K. (2018), “Analysis of supply chain complexity drivers for Indian mining equipment manufacturing companies combining SAP-LAP and AHP”, Resources Policy, Vol. 59, pp. 389-410.
Chen, H.H. and Lin, A.M. (2012), “Complex network characteristics and invulnerability simulating analysis of supply chain”, Journal of Networks, Vol. 7, pp. 591-597.
Chen, S., Zhu, F., Long, H. and Yang, J. (2019), “Energy footprint controlled by urban demands: how much does supply chain complexity contribute?”, Energy, Vol. 183, pp. 561-572.
Cheng, C.-Y., Chen, T.-L. and Chen, Y.-Y. (2014), “An analysis of the structural complexity of supply chain networks”, Applied Mathematical Modelling, Vol. 38, pp. 2328-2344.
Choi, T., Dooley, K. and Rungtusanatham, M. (2001), “Supply networks and complex adaptive systems: control versus emergence”, Journal of Operations Management, Vol. 19, pp. 351-366.
Choi, T. and Krause, D.R. (2006), “The supply base and its complexity: implications for transaction costs, risks, responsiveness, and innovation”, Journal of Operations Management, Vol. 24, pp. 637-652.
Chowdhury, M., Quaddus, M. and Agarwal, R. (2019), “Supply chain resilience for performance: role of relational practices and network complexities”, Supply Chain Management, Vol. 24, pp. 659-676.
Datta, P.P., Christopher, M. and Allen, P. (2007), “Agent-based modelling of complex production/distribution systems to improve resilience”, International Journal of Logistics Research and Applications, Vol. 10, pp. 187-203.
Day, J.M. (2014), “Fostering emergent resilience: the complex adaptive supply network of disaster relief”, International Journal of Production Research, Vol. 52, pp. 1970-1988.
de Leeuw, S., Grotenhuis, R. and van Goor, A.R. (2013), “Assessing complexity of supply chains: evidence from wholesalers”, International Journal of Operations and Production Management, Vol. 33, pp. 960-980.
Dittfeld, H., Scholten, K. and Van Donk, D. (2018), “Burden or blessing in disguise: interactions in supply chain complexity”, International Journal of Operations and Production Management, Vol. 38, pp. 314-332.
Drzymalski, J. (2015), “A measure of supply chain complexity incorporating virtual arcs”, Journal of Systems Science and Systems Engineering, Vol. 24, pp. 486-499.
Eckstein, D., Goellner, M., Blome, C. and Henke, M. (2015), “The performance impact of supply chain agility and supply chain adaptability: the moderating effect of product complexity”, International Journal of Production Research, Vol. 53, pp. 3028-3046.
Ekinci, E. and Baykasoğlu, A. (2019), “Complexity and performance measurement for retail supply chains”, Industrial Management and Data Systems, Vol. 119, pp. 719-742.
Fernández Campos, P., Trucco, P. and Huaccho Huatuco, L. (2019), “Managing structural and dynamic complexity in supply chains: insights from four case studies”, Production Planning and Control, Vol. 30, pp. 611-623.
Ge, H., Gray, R. and Nolan, J. (2015), “Agricultural supply chain optimization and complexity: a comparison of analytic vs simulated solutions and policies”, International Journal of Production Economics, Vol. 159, pp. 208-220.
Gerschberger, M., Engelhardt-Nowitzki, C., Kummer, S. and Staberhofer, F. (2012), “A model to determine complexity in supply networks”, Journal of Manufacturing Technology Management, Vol. 23, pp. 1015-1037.
Gerschberger, M., Manuj, I. and Freinberger, P. (2017), “Investigating supplier-induced complexity in supply chains”, International Journal of Physical Distribution and Logistics Management, Vol. 47, pp. 688-711.
Giannoccaro, I. (2015), “Adaptive supply chains in industrial districts: a complexity science approach focused on learning”, International Journal of Production Economics, Vol. 170, pp. 576-589.
Giannoccaro, I., Nair, A. and Choi, T. (2018), “The impact of control and complexity on supply network performance: an empirically informed investigation using NK simulation analysis: the impact of control and complexity”, Decision Sciences, Vol. 49, pp. 625-659.
Gimenez, C., van der Vaart, T. and van Donk, D.P. (2012), “Supply chain integration and performance: the moderating effect of supply complexity”, International Journal of Operations and Production Management, Vol. 32, pp. 583-610.
Gunasekaran, A., Subramanian, N. and Rahman, S. (2015), “Supply chain resilience: role of complexities and strategies”, International Journal of Production Research, Vol. 53, pp. 6809-6819.
Hamta, N., Akbarpour Shirazi, M., Behdad, S. and Fatemi Ghomi, S. (2018), “Modeling and measuring the structural complexity in assembly supply chain networks”, Journal of Intelligent Manufacturing, Vol. 29, pp. 259-275.
Hearnshaw, E.J.S. and Wilson, M.M.J. (2013), “A complex network approach to supply chain network theory”, International Journal of Operations and Production Management, Vol. 33, pp. 442-469.
Hu, S., Zhu, X., Wang, H. and Koren, Y. (2008), “Product variety and manufacturing complexity in assembly systems and supply chains”, CIRP Annals, Vol. 57, pp. 45-48.
Huatuco, L.H., Burgess, T.F. and Shaw, N.E. (2010), “Entropic-related complexity for re-engineering a robust supply chain: a case study”, Production Planning and Control, Vol. 21, pp. 724-735.
Inman, R. and Blumenfeld, D. (2014), “Product complexity and supply chain design”, International Journal of Production Research, Vol. 52, pp. 1956-1969.
Isik, F. (2010), “An entropy-based approach for measuring complexity in supply chains”, International Journal of Production Research, Vol. 48, pp. 3681-3696.
Jiang, J., Wu, D., Chen, Y. and Li, K. (2019a), “Complex network oriented artificial bee colony algorithm for global bi-objective optimization in three-echelon supply chain”, Applied Soft Computing Journal, Vol. 76, pp. 193-204.
Jiang, J., Wu, D., Chen, Y., Yu, D., Wang, L. and Li, K. (2019b), “Fast artificial bee colony algorithm with complex network and naive Bayes classifier for supply chain network management”, Soft Computing, Vol. 23, pp. 13321-13337.
Johnsen, T., Mikkelsen, O. and Wong, C. (2019), “Strategies for complex supply networks: findings from the offshore wind power industry”, Supply Chain Management: An International Journal, Vol. 24, pp. 872-886.
Kavilal, E.G., Prasanna Venkatesan, S. and Sanket, J. (2018), “An integrated interpretive structural modeling and a graph-theoretic approach for measuring the supply chain complexity in the Indian automotive industry”, Journal of Manufacturing Technology Management, Vol. 29, pp. 478-514.
Kim, W.-S. (2009), “Effects of a trust mechanism on complex adaptive supply networks: an agent-based social simulation study”, Journal of Artificial Societies and Social Simulation, Vol. 12.
Kinra, A. and Kotzab, H. (2008), “A macro-institutional perspective on supply chain environmental complexity”, International Journal of Production Economics, Vol. 115, pp. 283-295.
Klosterhalfen, S., Kallrath, J., Frey, M., Schreieck, A., Blackburn, R., Buchmann, J. and Weidner, F. (2019), “Creating cost transparency to support strategic planning in complex chemical value chains”, European Journal of Operational Research, Vol. 279, pp. 605-619.
Kriheli, B. and Levner, E. (2018), “Entropy-based algorithm for supply-chain complexity assessment”, Algorithms, Vol. 11.
Li, G., Ji, P., Sun, L. and Lee, W. (2009), “Modeling and simulation of supply network evolution based on complex adaptive system and fitness landscape”, Computers and Industrial Engineering, Vol. 56, pp. 839-853.
Li, G., Yang, H., Sun, L., Ji, P. and Feng, L. (2010), “The evolutionary complexity of complex adaptive supply networks: a simulation and case study”, International Journal of Production Economics, Vol. 124, pp. 310-330.
Ma, J., Lou, W. and Tian, Y. (2019), “Bullwhip effect and complexity analysis in a multi-channel supply chain considering price game with discount sensitivity”, International Journal of Production Research, Vol. 57, pp. 5432-5452.
Ma, Y.-F., Chen, L.-W., Meng, S.-D. and Yi, C.-Q. (2014), “A study on the risk control of supply chain under the background of globalization”, Journal of Industrial and Production Engineering, Vol. 31, pp. 221-228.
MacIntosh, R. and MacLean, D. (1999), “Conditioned emergence: a dissipative structures approach to transformation”, Strategic Management Journal, Vol. 20, pp. 297-316.
Manuj, I. and Sahin, F. (2011), “A model of supply chain and supply chain decision-making complexity”, International Journal of Physical Distribution and Logistics Management, Vol. 41, pp. 511-549.
McKelvey, B., Wycisk, C. and Hülsmann, M. (2009), “Designing an electronic auction market for complex ‘smart parts’ logistics: options based on LeBaron's computational stock market”, International Journal of Production Economics, Vol. 120, pp. 476-494.
Mertens, A., Van Meensel, J., Willem, L., Lauwers, L. and Buysse, J. (2018), “Ensuring continuous feedstock supply in agricultural residue value chains: a complex interplay of five influencing factors”, Biomass and Bioenergy, Vol. 109, pp. 209-220.
Milgate, M. (2001), “Supply chain complexity and delivery performance: an international exploratory study”, Supply Chain Management: An International Journal, Vol. 6, pp. 106-118.
Modrak, V. and Marton, D. (2014), “Configuration complexity assessment of convergent supply chain systems”, International Journal of General Systems, Vol. 43, pp. 508-520.
Modrak, V. and Semanco, P. (2011), “Complexity assessment of supply chains structure: a comparative study”, Research Journal of Applied Sciences, Vol. 6, pp. 410-415.
Musso, C. (2009), “New learning from old plastics: the effects of value-chain-complexity on adoption time”, Technovation, Vol. 29, pp. 299-312.
Nair, A., Narasimhan, R. and Choi, T. (2009), “Supply networks as a complex adaptive system: toward simulation-based theory building on evolutionary decision making”, Decision Sciences, Vol. 40, pp. 783-815.
Nair, A. and Reed-Tsochas, F. (2019), “Revisiting the complex adaptive systems paradigm: leading perspectives for researching operations and supply chain management issues”, Journal of Operations Management, Vol. 65, pp. 80-92.
Nilsson, F. and Darley, V. (2006), “On complex adaptive systems and agent-based modelling for improving decision-making in manufacturing and logistics settings – experiences from a packaging company”, International Journal of Operations and Production Management, Vol. 26, pp. 1351-1373.
Nilsson, F. and Gammelgaard, B. (2012), “Moving beyond the systems approach in SCM and logistics research”, International Journal of Physical Distribution and Logistics Management, Vol. 42, pp. 764-783.
Novak, S. and Eppinger, S. (2001), “Sourcing by design: product complexity and the supply chain”, Management Science, Vol. 47, pp. 189-204.
Olivares Aguila, J. and ElMaraghy, W. (2018), “Structural complexity and robustness of supply chain networks based on product architecture”, International Journal of Production Research: Modularity and Supply Chain Management, Vol. 56, pp. 6701-6718.
Omar, A., Davis-Sramek, B., Fugate, B. and Mentzer, J. (2012), “Exploring the complex social processes of organizational change: supply chain orientation from a manager's perspective”, Journal of Business Logistics, Vol. 33, pp. 4-19.
Pathak, S.D., Day, J.M., Nair, A., Sawaya, W.J. and Kristal, M.M. (2007), “Complexity and adaptivity in supply networks: building supply network theory using a complex adaptive systems perspective”, Decision Sciences, Vol. 38, pp. 547-580.
Pathak, S.D., Dilts, D.M. and Mahadevan, S. (2009), “Investigating population and topological evolution in a complex adaptive supply network”, Journal of Supply Chain Management, Vol. 45, pp. 54-67.
Piya, S., Shamsuzzoha, A. and Khadem, M. (2019), “An approach for analysing supply chain complexity drivers through interpretive structural modelling”, International Journal of Logistics Research and Applications, Vol. 23, pp. 1-26.
Posey, C. and Bari, A. (2009), “Information sharing and supply chain performance: understanding complexity, compatibility, and processing”, International Journal of Information Systems and Supply Chain Management, Vol. 2, pp. 67-76.
Rodewald, J., Colombi, J., Oyama, K. and Johnson, A. (2016), “Methodology for simulation and analysis of complex adaptive supply network structure and dynamics using information theory”, Entropy, Vol. 18, doi: 10.3390/e18100367.
Sayed, M., Hendry, L. and Zorzini Bell, M. (2017), “Institutional complexity and sustainable supply chain management practices”, Supply Chain Management: An International Journal, Vol. 22, pp. 542-563.
Serdarasan, S. (2013), “A review of supply chain complexity drivers”, Computers and Industrial Engineering, Vol. 66, pp. 533-540.
Shamsuzzoha, A. (2018), “Modular product architecture to manage supply chain complexity”, International Journal of Supply Chain Management, Vol. 7, pp. 31-37.
Sharma, A., Pathak, S., Borah, S. and Adhikary, A. (2019), “Is it too complex? The curious case of supply network complexity and focal firm innovation”, Journal of Operations Management, Vol. 66, pp. 839-865.
Shou, Y., Li, Y., Park, Y. and Kang, M. (2017), “The impact of product complexity and variety on supply chain integration”, International Journal of Physical Distribution and Logistics Management, Vol. 47, pp. 297-317.
Sivadasan, S., Efstathiou, J., Calinescu, A. and Huatuco, L.H. (2006), “Advances on measuring the operational complexity of supplier–customer systems”, European Journal of Operational Research, Vol. 171, pp. 208-226.
Skilton, P.F. and Robinson, J.L. (2009), “Traceability and normal accident theory: how does supply network complexity influence the traceability of adverse events?”, The Journal of Supply Chain Management, Vol. 45, pp. 40-53.
Statsenko, L., Gorod, A. and Ireland, V. (2018), “A complex adaptive systems governance framework for regional supply networks”, Supply Chain Management: An International Journal, Vol. 23, pp. 293-312.
Suo, Q., Guo, J., Sun, S. and Liu, H. (2018), “Exploring the evolutionary mechanism of complex supply chain systems using evolving hypergraphs”, Physica A: Statistical Mechanics and Its Applications, Vol. 489, pp. 141-148.
Surana, A., Kumara, S., Greaves, M. and Raghavan, U.N. (2005), “Supply-chain networks: a complex adaptive systems perspective”, International Journal of Production Research, Vol. 43, pp. 4235-4265.
Touboulic, A., Matthews, L. and Marques, L. (2018), “On the road to carbon reduction in a food supply network: a complex adaptive systems perspective”, Supply Chain Management: An International Journal, Vol. 23, pp. 313-335.
Tu, H., Mao, X. and Wang, X. (2019), “Complexity of a dynamic hybrid supply chain game model with a service factor”, Nonlinear Dynamics, Vol. 97, pp. 2055-2066.
Turner, K. and Williams, G. (2005), “Modelling complexity in the automotive industry supply chain”, Journal of Manufacturing Technology Management, Vol. 16, pp. 447-458.
Turner, N., Aitken, J. and Bozarth, C. (2018), “A framework for understanding managerial responses to supply chain complexity”, International Journal of Operations and Production Management, Vol. 38, pp. 1433-1466.
Vachon, S. and Klassen, R.D. (2002), “An exploratory investigation of the effects of supply chain complexity on delivery performance”, IEEE Transactions on Engineering Management, Vol. 49, pp. 218-230.
Varga, L., Allen, P., Strathern, M., Rose-Anderssen, C., Baldwin, J. and Ridgway, K. (2009), “Sustainable supply networks: a complex systems perspective”, Emergence, Vol. 11, pp. 16-36.
Wang, H., Gu, T., Jin, M., Zhao, R. and Wang, G. (2018), “The complexity measurement and evolution analysis of supply chain network under disruption risks”, Chaos, Solitons and Fractals, Vol. 116, pp. 72-78.
Wanke, P. and Corrêa, H. (2014), The Relationship between the Logistics Complexity of Manufacturing Companies and Their Supply Chain Management, Uma Publicação Da Associação Brasileira de Engenharia de Produção, Produção, Vol. 24, pp. 233-254.
Wilding, R. (1998), “The supply chain complexity triangle: uncertainty generation in the supply chain”, International Journal of Physical Distribution and Logistics Management, Vol. 28, pp. 599-616.
Wong, C.W.Y., Lai, K.-H. and Bernroider, E.W.N. (2015), “The performance of contingencies of supply chain information integration: the roles of product and market complexity”, International Journal of Production Economics, Vol. 165, pp. 1-11.
Wu, Y., Frizelle, G. and Efstathiou, J. (2007), “A study on the cost of operational complexity in customer–supplier systems”, International Journal of Production Economics, Vol. 106, pp. 217-229.
Wycisk, C., McKelvey, B. and Hülsmann, M. (2008), “Smart part supply networks as complex adaptive systems: analysis and implications”, International Journal of Physical Distribution and Logistics Management, Vol. 38, pp. 108-125.
Yang, B. and Yang, Y. (2010), “Postponement in supply chain risk management: a complexity perspective”, International Journal of Production Research, Vol. 48, pp. 1901-1912.
Zhang, F. and Liu, J. (2013), “Evolution modeling of degree preference supply chain network”, Journal of Applied Sciences, Vol. 13, pp. 4428-4434.
Zhao, K., Zhiya, Z. and Blackhurst, J.V. (2019), “Modelling supply chain adaptation for disruptions: an empirically grounded complex adaptive systems approach”, Journal of Operations Management, Vol. 65, pp. 190-212.