Abstract
Purpose
The study queries the drivers of innovation management in contemporary data-driven organizations/companies. It is argued that data-driven organizations that integrate a strategic orientation grounded in data, human abilities and proactive management are more effective in triggering innovation.
Design/methodology/approach
Research reported in this paper employs constructivist grounded theory, Gioia methodology, and the abductive approach. The data collected through semi-structured interviews administered to 20 Italian start-up founders are then examined.
Findings
The paper identifies the key enablers of innovation development in data-driven companies and reveals that data-driven companies may generate different innovation patterns depending on the kind of capabilities activated.
Originality/value
The study provides evidence of how the combination of data-driven culture, skills' enhancement and the promotion of human resources may boost the emergence of innovation.
Keywords
Citation
Visvizi, A., Troisi, O., Grimaldi, M. and Loia, F. (2022), "Think human, act digital: activating data-driven orientation in innovative start-ups", European Journal of Innovation Management, Vol. 25 No. 6, pp. 452-478. https://doi.org/10.1108/EJIM-04-2021-0206
Publisher
:Emerald Publishing Limited
Copyright © 2021, Anna Visvizi, Orlando Troisi, Mara Grimaldi and Francesca Loia
License
Published by Emerald Publishing Limited. This article is published under the Creative Commons Attribution (CC BY 4.0) licence. Anyone may reproduce, distribute, translate and create derivative works of this article (for both commercial and non-commercial purposes), subject to full attribution to the original publication and authors. The full terms of this licence may be seen at http://creativecommons.org/licences/by/4.0/legalcode
1. Introduction
Smart technologies and the digitalization of business processes may foster the development of innovation (Lee et al., 2014). However, the implementation of a smart technological architecture is not synchronous with the emergence of innovation (Chen et al., 2015; Gupta and George, 2016). The redesign of organizational models through new technologies requires that strategic data management be applied to improve decision making processes and to prompt innovative insights. In this regard, data-driven decision making (DDDM) suggests thee need to reframe and rethink business practices (LaValle et al., 2011; Brynjolfsson et al., 2011). This implies that data is viewed as the driving force behind more effective decision-making to challenge the complexity of the context in which businesses operate (Malik et al., 2021). Research on DDDM suggests that uncritical and devoid of strategy use of technological tools does not foster innovation (Manyika et al., 2011; Wamba et al., 2015). Rather, it is the way people actively use these tools, e.g. through the exchange of knowledge and other practices, that individually or in conjunction may trigger innovative processes and, thus, innovation.
The advent of big data and digital technologies has led to a redefinition of innovation management (Trabucchi and Buganza, 2019). The latter emphasizes the positive role of digitalization in shaping the innovation process, and in redefining the traditional modalities of delivering product and services (Fichman et al., 2014; Wosiek and Visvizi, 2021). This notwithstanding, the literature on innovation tends to focus solely on technology, rather than on the identification of the skills necessary for people to seize innovation opportunities (Perri et al., 2020). In this paper, it is argued that data-driven orientation, strategies and practices (DDDM principles) may foster the adoption of ICT-enhanced structures that combine strategic orientation, skills and process management aimed at extracting value from data. Hence, synergies, in the form of innovative insights and innovation, can only be attained if human capabilities are factored in the process (Wu et al., 2021).
The objective of this paper is twofold: (1) to explore the key enablers of innovation development in data-driven companies (RQ1); and (2) to identify and map the different types of innovation that are activated through the application of data-driven orientation (RQ2). To address these questions, the concepts of data-driven orientation and innovation management are employed to build a framework for the subsequent empirical analysis. Here, semi-structured interviews, administered to twenty (20) Italian start-up founders, are examined through the lens of grounded theory (Mills et al., 2006) and Gioia methodology (Gioia et al., 2013). These are complemented by an abductive procedure. The paper is structured as follows. First, the main principles of data-driven orientation and the key approaches to innovation management in digital era are discussed. Then, the methods and methodology employed in the empirical research, including the limitations, are elaborated. Subsequently, the interviews are examined and discussed to showcase the varieties of instances of innovation developed in data-driven and human oriented companies. Discussion and conclusions follow.
2. The conceptual framework: the role of data-driven orientation in the development of innovation
2.1 Data-driven orientation: key approaches and dimensions
To comply with the challenges of digitalization, digital technologies and data analysis need to be seen as the levers, or means, of innovation development, rather than as a goal, or the end of the process. In this vein, DDDM (LaValle et al., 2011; McAfee and Brynjolfsson, 2012) is conceptualized to explore the key dimensions of business operation that should be harmonized to integrate digital orientation and human resources management, and in this way to support the redefinition of innovation management in the light of a combined human/digital view.
As the concept of big data evolves (Chen et al., 2012), studies on data-driven orientation move from a technological viewpoint (Kusiak, 2009) to a managerial perspective (Chen et al., 2015; Gupta and George, 2016). Here the need to extract relevant meanings and to create value from data, through the transformation of such data assets as key strategic organizational drivers (Trabucchi and Buganza, 2019; Troisi et al., 2019) is stressed.
In a world ruled by data, the novelty and the usefulness of this approach rests in its focus on a mind-set that companies need to adopt to strategically manage technology, to survive, and to thrive by staying innovative in today's business world. The proper adoption of data-driven orientation can reveal how the implementation of a technological architecture is a necessary but not a sufficient condition to facilitate the emergence of innovation (Medina-Borja, 2019). Managers should integrate data collection with the process of interpretation and with the transformation of data into information and knowledge (Spender et al., 2017; Visvizi et al., 2018b). Only in this way a link between data, continuous learning and innovation opportunity can be established.
In other words, if the imperative of a data-driven innovation-focused growth and development of a business is to be attained, the following steps are necessary. First, data-driven companies should re-focus their strategic objectives to conceive of data as the key levers of efficient decision-making, and consequently should adopt a constant start-up attitude. Against this backdrop, the second step is the implementation of a technological infrastructure (Järvinen and Karjaluoto, 2015) based on smart technologies that can ensure the proper management and extraction of information to improve the accessibility and the integration of data (Chaffey and Patron, 2012). Then, to gain relevant information, the ability to integrate, analyse and interpret data in line with the strategic objectives is required. As a result, the opportunities that derive from smart technologies require active human intervention to extract value from data (Chen et al., 2015). Subsequently, the diverse sources of data should be optimized to harmonize input, process and output data that intersect with different functions of the value chain. Lastly, information computing should be supervised by managers to enable the transformation of information into knowledge (Troisi et al., 2019). Therefore, as Figure 1 shows, data-driven orientation needs to integrate three dimensions, i.e. (1) technological; (2) human; (3) managerial.
However, the DDDM debate falls short as regards the question of how to foster the extraction of knowledge from data analysis and, thus, to enhance the transformation of data into innovation opportunities. That is, the relationship between people who activate technology according to a data-driven orientation in contemporary companies (Lepri et al., 2017) on the one hand, and skills and managerial orientation (Carayannis et al., 2018) on the other, remains under-researched. Hence, an insight into how the key dimensions of data-driven orientation can be integrated and harmonized in business practices can contribute to the conceptualization of the complex process of the redefinition of business models, strategies and processes necessary to address the emerging challenges of digitalization.
2.2 The development of innovation in data-driven companies
The advent of digital economy (Saeedi and Visvizi, 2021) and the diffusion of data-driven mindset demand that businesses redefine their orientation, business models, processes and innovation management strategies (Loia et al., 2021). Yet, the impact of ICT and digital technologies on the development of innovation is complex. Technology plays the role of either an enabler, a mediator or the outcome of data-driven management that can promote innovation (Zhou et al., 2014). There has been a tendency in the literature to conceive of innovation mostly as a function of technology (Mothe and Nguyen, 2010; Henao-García and Montoya, 2021). However, as business digitalization increases, the conceptualization of innovation (OECD, 2005) needs to be broadened to include human and social dimensions (Snyder et al., 2016). In other words, innovation is a multi-variate concept that requires multi-scalar input to emerge.
To bypass the limitation of the technology-driven approach, managers should employ an integrated perspective that combines digital, knowledge-based and social orientation in the implementation of innovation strategies. Hence, a multi-dimensional process such as innovation development should be grounded in a complex mix of knowledge-based (human) and institutional (social) dimensions (Lee and Trimi, 2018; Trabucchi and Buganza, 2019). That is, it is necessary to adopt an integrated perspective that bridges the different facets of innovation (Troisi et al., 2021). As synthesized in Figure 2, in the extant research three different approaches to the study of innovation have been identified: (1) technology-driven; (2) knowledge-based; (3) social-oriented.
Traditionally, technological innovation (OECD, 2005) has been conceptualized as the process through which technological advances are produced (Nieto, 2004; Schmidt and Rammer, 2007). It thus included a set of activities that contributed to the increase of the capacity to produce new goods and services (product innovation) or to implement new forms of production, e.g. process innovation (Utterback and Albernathy, 1975). Therefore, commonsensically, even today, technological innovation tends to be considered as the integration of product and process innovation (Mothe and Nguyen, 2010; Zhou et al., 2021). Interestingly, however, also in the past, the technology-driven approach to innovation has been criticized (Hipp and Grupp, 2005) on the grounds of the underrating of, on the one hand, the intangible variables (such as relations, human skills, the mindset etc.) and, on the other hand, of the outcomes (business models, culture, etc.) involved in and arising from the development of innovation. Against these debates that had shaped the field, today the literature (Schmidt and Rammer, 2007; Mothe and Nguyen, 2010) identifies the need to broaden the definition of innovation. In this view, it argued that the concept of innovation necessarily needs to integrate technology, human, knowledge-based, social and relational variables. In this view, the discussion on innovation can be conceptualized as integrating three dimensions, i.e. (1) the traditional one, technology oriented; (2) the focused on the individuals and their capacities, knowledge-based approach (Anand et al., 2007; Shujahat et al., 2019); and (3) the social-oriented approach (Harrisson et al., 2009).
Knowledge-based innovation stems from studies that apply a knowledge-based view. It is thus a firm/organization level theory that conceptualizes organization as the combination of heterogeneous knowledge assets to develop competitive advantage (Denford, 2013). In this approach, innovation is viewed as the result of the creation of new knowledge to solve an unexpected or voluntarily created problem (Dougherty, 2004).
Innovation opportunities in this context arise through the constant mediation and interaction between the human agency (capabilities, knowledge, attitude) and the non-human artifacts and tools (Martín-de Castro et al., 2011), i.e. elements of the structure. What follows is that (digital) skills and competencies are central in the pursuit of innovation. Technology is still one of the key elements but is conceived as a tool that enables knowledge application. As such it should be negotiated through human interactions and resource integration (Martín-de Castro et al., 2011; Mele and Polese, 2011).
From a different perspective, knowledge management can be considered as one of the key levers to develop business model innovation (BMI). The latter can be defined as the discovery of new modes of value proposition, value creation and value capture in an existing organization (Teece, 2010; Bashir and Farooq, 2019). Technological advancement can also serve as a driver for business model innovation. However, the concept of BMI stems from the willingness to overcome the technological focus (Chesbrough, 2007). Hence, even if technology is a lever for innovation, it should be integrated with the introduction of new value proposition, a rethinking of orientation and knowledge management strategies.
The last approach, social-oriented innovation, refers to an emerging area of studies (Van der Have and Rubalcaba, 2016) that stems from the development of new research trends, e.g. social enterprises and social entrepreneurship. These are based on the incorporation of social sphere into management practices and on the criticism of dominant business models (Harrisson et al., 2009). In this perspective, innovation does not imply only the introduction of cutting-edge technology but should also solve social problems, satisfy different human needs, change social relations and increase citizens' empowerment (Ceci et al., 2019).
The advent of social innovation does not involve the abandonment of technological innovation. The literature recognizes the systemic interdependence of social and technological innovation processes (Howaldt et al., 2016). The creation of new social value and the enrichment of actors' participation in decision-making are considered as sources of BMI that stems from a change in businesses activities and value.
The combination of technological, knowledge-based and social factors in the development of innovation may foster the adoption of new business models based on open innovation (OI) Chesbrough (2007). Technology advancement is one of the key goals of open innovation (Baden-Fuller and Haefliger, 2013) and the different combination of internal and external knowledge sharing can give birth to different technological innovation. OI models are based on external collaboration that enhance interaction and knowledge sharing that can create new knowledge, new ideas, new opportunities for innovation. At the same time, it can be noticed that often social innovation is grounded on open innovation paradigms aimed at solving social problems.
Hence, inflows and outflows of knowledge, technology and social shades of innovation are strictly interrelated in the adoption of open innovation strategies, which can be considered as an overarching approach to the systems conceptualization of innovation.
2.3 The research questions
Despite the reinterpretation of innovation as a multi-dimensional construct that involves technological and non-technological processes, the connections among the different types of innovation and the role of non-technological innovation remain under-discussed in the literature (Damanpour, 2014). Clearly, the need to investigate the different shades of innovation to account for the human side of innovation and to explore the loci of innovation in all the different aspects of management has been recognized (Henao-García and Cardona Montoya, 2021; Troisi et al., 2021).
One of the broad questions that remains is how managers embrace the challenge of adapting to the new (digital) business reality. More specifically, the question is how to successfully reorient the existing business models), culture and organizational structure to effectively manage digital technology and to promote business innovation and sustainability (Lewis et al., 2004)?
Past studies sought to identify the enabling dimensions of innovation in digitalized companies (Bouwman et al., 2018), the role of dynamic capabilities (Rachinger et al., 2019) and skills (Sousa and Rocha, 2019) and to explore the complex process of redefinition of entrepreneurship and resources management to comply with the requirements of digitalization (Beliaeva et al., 2019). Moreover, some works offer a conceptualization of the different capabilities that can help exploit the opportunities of big data in data-driven companies (Gupta and George, 2016) and encourage innovation (Troilo et al., 2017).
However, previous contributions do not classify the drivers and the underlying mechanisms for the emergence of innovation in data-driven companies (Trabucchi and Buganza, 2019) by adopting a multilevel perspective that can help further research systematize and measures the drivers and outcomes of innovation process.
In line with the need to adopt an ell-encompassing view on innovation, this study considers both the drivers for innovation as a process and the potential outcome by trying to provide future studies with an extensive conceptualization that can lead to the further measurement of innovation construct through quantitative research.
Extant research confirms the definition of innovation as both an outcome and a process (Mothe and Nguyen, 2010; Kahn, 2018). To shed light on the comprehensive nature of innovation in digital era, the research aims at investigating the two roles of innovation.
Innovation as a process refers to the way in which organization should encourage the development of novelties to generate enjoyable outcomes. Hence, it precedes the development of outcomes.
To explore innovation as an outcome requires the analysis of the output produced in the company, e.g. the most “classical” types of innovation identified above (product innovation, process innovation, business model innovation, social innovation).
By starting from some macro-categories identified in extant research on data-driven orientation, the potential enablers of innovation in data-driven companies are explored through the empirical research to corroborate, enrich and extend the enabling dimensions identified in literature (technological infrastructure, human resources and skills, managerial attitude) that comply with the main drivers of innovation as a process in general (Crossan and Apaydin, 2010).
Even if extant research explains conceptually the difference between innovation as a process and innovation as an outcome, there is the need to shed light on the mechanisms that connect the two roles of innovation (Quintane et al., 2011).
For this reason, this study aims at exploring innovation both as a process (RQ1) and as an outcome (RQ2). Since the drivers of innovation are considered as one of the most relevant dimensions of innovation seen as a process (Crossan and Apaydin, 2010), the first research question that this paper addresses is:
Which are the key enablers of innovation in data-driven companies? (Figure 3)
Therefore, this study queries the conditions under which innovation as a process becomes a function of a skilful exploitation of the strategic opportunities deriving from an effective data management. The value generated from the combination of technological, human, managerial and social dimensions in data-driven companies can enable the emergence of diverse innovation outcomes. Different actors with different orientations and capabilities can interpret and develop new entrepreneurial opportunities differently. The varied nature of human capabilities and skills (technical, managerial, relational, etc.) and of entrepreneurial opportunities can produce distinct types of innovation. The literature offers some insights into this issue (Snyder et al., 2016) by placing new business practices and social benefits (social innovation; sustainable innovation) in the spotlight.
Still, it is imperative that the different types of innovation that can be created from data-driven firms that integrate technological, human and managerial dimension are explored in detail.
Moreover, to examine simultaneously innovation in data-driven companies as a process and an outcome, the second research question of the study aims at investigating the different types of novelties that can be generated through a process of data-driven oriented innovation development. In fact, the link between the process and the outcome of innovation is still unclear, due to a lack of definition for innovation as an outcome (Quintane et al., 2011). Literature emphasizes the need to classify the novelties introduced in a company after innovation development into categories for further analysis (Smart et al., 2007).
This is precisely what the second research question purports to do (see Figure 3):
Which types of innovation can be generated in data-driven companies?
3. Methodology
3.1 Research design
The complexity in the definition and in the interpretation of the different dimensions of data-driven orientation and of the different enablers and outcomes of innovation requires a multi-level research design. Innovation, both as a process and as an outcome, does not “exist” objectively but can be built subjectively through the mediation of human, cultural and social dimensions. Similarly, knowledge and skills recombination for the development of innovation derive strictly from the co-creation and unpredictable joint generation of novelties (abilities, meanings, rules, practices, value, etc.). Therefore, there is the need for a research approach that allows the detection of the “hidden” variables that researchers cannot control easily and of multi-dimensional sub-categories of analysis whose boundaries of meanings are blurred (Yin, 2011).
To this end, an exploratory research based on qualitative approach is conducted and its results are examined through the constructivist grounded theory (Mills et al., 2006; Glaser, 2007) and Gioia methodology (Gioia et al., 2013). Grounded theory (Strauss and Corbin, 1994) is based on the constant comparison of data collected and new classifications and conceptualizations arising from the analysis. Constructivist grounded theory helps the identification of themes that are grounded in and emerge from data and can allow a deeper exploration of concepts and relationships between them (Nag and Gioia, 2012; Castellani et al., 2020). For this reason, this methodology, applied through the administration of in-depth interviews, seems to be suitable for the analysis of both drivers and outcomes of a complex process such as innovation by ensuring a holistic understanding of respondent's attitude, experiences and behavior that cannot be easily obtained through other methodology.
The Gioia methodology implies a continuous reproduction of the interpretative cycle of data-theory (“data collection”, “reconnection of results to theory”), in which the data collected is compared with “old” knowledge and with existing theory (Bonfanti et al., 2019). Through the application of abductive inferential processes, new concepts are re-elaborated from the results, a renewal of meanings follows to encourage the establishment of new valid relationships between concepts and constructs. Several stages of data analysis, interpretation and re-elaboration are required to generate new conceptual models and discover complementary conceptualizations.
The empirical research included four stages (see part 3.2). The administration of semi-structured interviews lasted six months. The interviewees were young entrepreneurs (from 22 to 38 years) of companies that offer digital services, technologies and consulting firms, contacted during a series of events (E-commerce & co., Growth Hacking Universe, etc.) held in Salerno and launched by Start-up Grind, a community of Google for Entrepreneurs between October 2019 and January 2020). The interviews lasted between 20 and 45 min and were conducted by phone (due to the restrictions of Covid-19). In line with the sampling criteria adopted in qualitative approach, once theoretical saturation was reached, the interview process was halted.
As reported in Table 1, twenty start-up founders participated in the research. The size of the final sample is suitable for exploratory research that aims at investigating the different shades of meaning of concepts which have not been operationalized in extant research. For instance, the different enabling factors and types of innovation have not been yet classified in previous studies according to a multilevel perspective (Kahn, 2018). The conceptualization and measurement of the different innovation outcomes according to a comprehensive view that ranges from technological to knowledge-based innovation has not been proposed (Mothe and Nguyen, 2010). Moreover, extant research identifies a gap in the identification of the key sub-dimensions of a socio-technical definition of innovation (Howaldt et al., 2016), of social innovation (Van der Have and Rubalcaba, 2016), of knowledge-based innovation (Quintane et al., 2011).
Moreover, for the advancement of some practically relevant theoretical tools that can be validated through further qualitative and qualitative studies the size of the sample does not represent an issue. Even if the number of interviews is not considered an issue, literature identifies a range from 15 to 30 participants (Morse, 2000; Dworkin, 2012) as the minimum sample size required to reach saturation in grounded theory studies that use in-depth interviews. This number can allow the exploration of research questions, the identification of differences between the conceptual categories of interest and the maximization of the possibility that data can clarify the relationships between the conceptual categories analyzed (Charmaz, 2006).
The description of the interviewees, of their different entrepreneurial roles and sociodemographic characteristics is provided in Table 1. The researchers attributed interviewees a code based on their job position in the companies (F, founders; M, managers; C, consultants).
3.2 Data collection and analysis
In line with the methodological criteria of semi-structured interviews (Corbetta, 2003), a list of themes, rather than structured questions, was used to design the interview sketch (see Appendix). The technique of semi-structured interview aims at “building” intersubjective representations and interpretations of the investigated variables constructed collaboratively through the reciprocal process of perception, interaction, communication and meaning exchange between interviewers and the interviewees. For this reason, it is suitable for the analysis of elaborated in this paper.
The interviews were recorded and transcribed and interpreted by the entire team. Notably, to ensure validity, respondents were asked to review the transcripts and confirm the accuracy of the information collected. The data collected was examined and interpreted through the technique of qualitative content analysis, in line with the precepts of the method proposed by Gioia et al. (2013). Content analysis as an inquiry allows at collecting data through an analysis form, resembling a questionnaire, in which texts (the transcribed interviews), considered as units of classification, are “interviewed” by researchers. The interpretative process required to fulfill this kind of content analysis is neither structured nor codified through specific parameters. Hence, the need to integrate this technique with the criteria laid down in the Gioia methodology. Figure 4 depicts the stages of the research process, described in the following sub-paragraphs.
3.2.1 Theorization
At this stage, after the identification of the research questions, the leading themes were identified to build the interview sketch (see Appendix). The themes derive from a literature review on the key macro-areas of the study (see section 2). Thus, starting from the key dimensions of data-driven orientation and from the key approaches to innovation (as discussed in paragraph 2.1, the macro-area “technological innovation” is sub-divided into product and process innovation) the questions of the interview have been elaborated. As reported in Figure 5, for each dimension of the three macro-areas investigated, different items have been obtained, which have been turned into questions in the interview sketch and leading themes during the data collection process. By means of these items, reframed as assertive statements during the administration of interviews, researchers guided and oriented the discussion with the entrepreneurs in the sample to permit the emergence of the different sub-topics explored. The aim of the process is not to compromise the expression of the real perceptions, opinions and evaluations of the entrepreneurs to ensure that the discussion of the key variables investigated is not “forced” by the researcher and that interviewees do not perceive the dialogue as an “interrogation”.
3.2.2 Coding
The goal of the first step of data analysis (coding) is to systematize the raw data obtained from the simple transcription of interviews. Researchers connected the recurring elements underlying the data corpus to the dimensions and triggers of data-driven orientation and innovation inductively (from the specific to the general dimension) through the comparison between data (specific observation) to theory (generalized concepts). To ensure data triangulation, each researcher coded independently all textual data to derive the first-order concepts. Then, starting from the multiple coding of each textual units, consistency checks are performed to compare coding schemes and detect any discrepancies to obtain a unique coding scheme.
As a result, the theoretical dimensions that guided the elaboration of the interview sketch and data collection were revised, enriched, edited and broadened to include the novelties emerged from the empirical data.
3.2.3 Categorization
The stage of categorization connects the findings obtained in the coding to the different dimensions identified as guidelines of the research by fostering the transition from first-order concepts to a new understanding of data, which can address the further development of new theories (see Figure 4). Then, the results of the coding are classified by the researchers to identify novel conceptual categories (second-order themes), more specific topics obtained from the “semantic” aggregation of first-order concepts. Second-order themes stem from the continuous interrelation between deductive and inductive processes, in which the key dimensions of the three macro-areas of the research are reviewed, specified, and reworked through complex cycles of interpretation.
3.2.4 Conceptualization
Conceptualization is based on the extraction of minimum units of meaning from the sub-categories identified in the categorization. Thus, second-order themes are aggregated and transformed into: (1) different enablers of innovation resulting from the data-driven practices implemented in the sample (RQ1); (2) different types of innovation deriving from the multiple enablers activated by data-driven orientation (e.g. constant sharing of data and enrichment of skills, RQ2). The final conceptual categories are derived through a reaggregation of the second-order themes that are reconnected to the key macro-areas of the study. The “labels” of the categories are identified through deduction processes that detect the underlying conceptual cores of the different (specific) second-order themes and reconnect them to some macro-categories (the general aggregate dimension), through comparisons and similarities between concepts. After the conceptualization, some of the dimensions borrowed from literature are confirmed, whereas others are deleted (e.g. for the category “technological innovation” only “product innovation” is maintained) and new categories are introduced (e.g. cultural change). At the end of the process, the results are conceptualized within a framework that classifies the different sub-types for each research question.
4. Results
4.1 The enabling data-driven dimensions of innovation
The strategic orientation of the entrepreneurs in the sample derives from the adoption of a learning culture based on the relevance of data (data-driven dimension 1) as an essential lever for the creation of innovation opportunities. Even if it is expected that data overturns human decisions, the start-up founder F1 affirms that “normally data can be full of bias and can foster preconceptions, sometimes can help the creation of conversation, but people cannot ‘unmask' data! Data ‘simply' starts the dialogue”. The strategic approach to data management for better decisions should be associated necessarily with a culture of learning, experimentation, a horizontal power distribution that reduces conflicts. Therefore, data is considered as a key strategic driver, but decisions are undertaken “not only based on analytics but also on listening, on the capability to be responsible, to be entrepreneurs, to experience [..] there is the need to listen to customers before than analyzing data from the external environment (M3)”. Managerial experience and entrepreneurial attitude are shared with the organizational members to increase their capabilities by means of e-learning tools such as microlearning. As stressed by interviewee C1: “micro-learning is useful for the creation of a corporate learning system, which permits people to have a 360-degrees learning experience and allows business experts to share their experience, without interrupting their work, by creating over time a vast library of knowledge available to new employees (such as Docebo)”.
This data-orientation enables the activation of the technological infrastructure through the dynamic integration of knowledge among actors which can foster the creation of new skills (data-driven dimension 2) and the empowerment of human resources (data-driven dimension 3) by generating the development of innovative projects based on distributed decision-making. The goal of the implementation of a strategic data architecture is to obtain heterogeneous set of data to reduce bias: “we should learn a lot more on data, especially on data lake, a lake that can contain all the data of the business and interpolate them … we should have a lot of data sources to do this (two-hundred at least). If you have small dataset this is useless (interviewee C2)”.
The adoption of a data-driven culture mediated through human intervention translates into the creation of a series of training and open innovation activities aimed at increasing employees' skills (data-driven dimension 2). In the description of the role of competencies in the generation of value, interviewee F8 affirms: “hard skills are less strategic than soft ones, we recruit people that have at least 10 years of experience, so we take for granted that they own some basic technical competencies. Our biggest concern is that they are able to manage customers in good times and bad times”.
Therefore, technical tasks related to data analysis and collection are based on the activation of hard skills, that are considered as a basic prerequisite, whereas empowerment, commitment and loyalty are based on the activation of soft skills, on which employees should compete. Then, soft skills can make the difference in the attainment of competitive advantage and, potentially, of innovation. Hard skills are based on technical competencies for big data analysis through the use statistical analytics and refer to the ability to extract meaningful findings from the data collected. Therefore, they imply the capability to use analytical thinking.
According to the open innovation manager in the sample (interviewee M6), the ability to analyze data should be associated with methodological sensitiveness: “data analysis can be source of many bias: not only we should collect information from heterogeneous sources, but we should compute and interpret data in line with strategic goals to identify solutions for the development of services, products and processes”.
Taken for granted the hard skills, the entrepreneurs in the sample strongly believe in the relevance of soft skills, individual's subjectivity and creativity as value-added resources for data interpretation. “The machines will always be faster than men in analyzing data, but they will never replace their interpretation, especially when the ethical aspect of decisions comes into play (interviewee M5)”. Therefore, critical thinking, human interpretation and hermeneutics abilities to find innovative solutions starting from data interpretation are strategic soft skills that can provide a company with inimitable know-how. Moreover, communication ability to share the interpreted data with members at different organizational level is needed. As declared by interview F9 (supply chain and operation manager), “the building of multi-skills team is essential to simplify process and enhance real time communication (on data, performance, users' behavior) between management and employees, to enable discussion and to allow management to turn the relevant information into decisions”.
The adoption of a cohesive set of smart technologies for data analysis that allows the constant exchange of skills can foster human resources management strategies aimed at empowering the people engaged in the organization (data-driven dimension 3). Distributed leadership processes enable the creation of digital teams to support Agile working and to enhance not only the engagement of human resources but also diffused decision-making processes and the development of innovation projects. As declared by interviewee M6: “distributed teams permit internal and external actors to work on a common information basis thanks to some single points to manage the information, such as on-board system in which information is stored and shared in real time”.
Moreover, the promotion of human resources is one of the strategic priorities of the interviewees in the sample, who consider people a key resource for the strategic accomplishment of goals. According to most entrepreneurs, people come before planning. As emphasized by interviewee F3 (non-profit company founder): “for us the priority is the growth of people rather than the growth of projects. To make project grow without making people grow is useless and can increase the risk to “use” people and make them leave in a few years”.
The activation of knowledge integration and the empowerment of human resources can foster the improvement of processes through distributed decision-making (data-driven dimension 5) and encourage the co-development of innovation. The contamination between business units is considered necessary in the sample. As affirmed by interviewee C4 (innovation consultant): “from the comparison with different points of view and with different skills, novelties and experiments certainly emerge [..] Experiments may fail, but they are necessary for the survival of companies and for being able to compete in an ever-faster ecosystem”. However, data is not always considered as enabler of value and knowledge creation, since “the dialogue between organizational members and with customers can replace, integrate or contradict data (interviewee F1)”.
Despite the potential negative implications of data, the experience of the entrepreneurs in the sample shows that the synergistic integration between a learning orientation, the active resources and skills' exchange among actors and the empowerment of human resources through distributed decision-making can promote continuous improvement (data-driven dimension 6) and the regeneration of innovation. The interviewees (F1, F9 and M6) declare to use platforms and services (such as Amazon services) “for internal management and projects management to understand when the system fails, to optimize processes based on iterative checks and make test on projects efficacy every two weeks”. Thanks to these instruments, companies can design projects on engineering systems and can be able to understand where the process stops. Moreover, A/B testing is employed “to understand when splitting the same activity into different groups can make things improve, to find the best solution to spend less time and money […] to find solutions that at the beginning we could never have imagined (interviewees F4; F9)”.
Figure 6 synthesizes the findings discussed above by highlighting the transition from first-order concepts to second-order themes and aggregate dimensions. As the last two column show, the key dimensions of data-driven orientation identified in literature (technological, human and managerial) are specified through the complex deduction-induction cycle of data analysis and turned into data-driven enablers of innovation that should be “activated” in data-driven companies through the intervention of human abilities and skills.
4.2 The emergence of different types of innovation
The activation of the data-driven enabling dimensions of innovation identified in the previous paragraph (RQ1) encourages the development of skills that, combined with the promotion of human resources, foster the emergence of different innovation opportunities.
Product innovation is viewed mainly as optimization. Interviewee M7 (innovation manager) affirms: “through our knowledge we support general managers of our corporate customers by offering them the right skills to innovate and to deal with optimization [..] we follow the Mc Kinsey Horizon Model: in the short run, businesses can pursue optimization, in the medium run can increase R&D, in the long run can attain innovation”.
One of the interviewees describes the process innovation offered to a customer through data-driven practices in the following way: “we shared data lake approach with a big food corporation, and they can save two million on animal wastes (interviewee F6)”. Therefore, data-driven innovation can lead not only to the introduction of new products/services but can also simplify and boost business process. Moreover, “internal” process innovation and continuous improvement are pursued in the sample thanks to specialized tools for project management, upgraded based on the insights collected from data: “we changed the interface of one of our internal tools for projects management, since we made some tests, collected data and noticed that people work better if they have information in the forefront to rule better the projects, to detect if something gets worst and to help the others (interviewee F7)”.
Since these capabilities are shared, data-driven companies can generate some types of innovation that go beyond the proposition of improved services to offer new business models. Interviewee F1 describes the business model innovation realized through the provision of strategy to switch business model: “we allowed the general manager of one of our partners at working on his business model: thanks to OKR objectives, we permitted to move a part of human resources on a new business model, with new technologies and new targets”. In this way, new forms of entrepreneurial orientation and new kinds of resource management practices can accelerate the internal transformation process and support top management in strategic decisions to foster innovation and organizational change.
According to interviewee F2 (digital consultant and social media strategist), “any type of company should find a new ‘identity', by redesigning its role and organizational profile and guiding companies towards the adoption of new organization models that make it possible to exploit completely the ‘human capital' [..] the HR department should develop a constant tension to change, by risking continuously and reinforcing its identity”.
The systems perspective on innovation and the shift of attention to human features and data-driven practices is emphasized by interviewee F5: “for us innovation is not just technological: technologies being equal, sometimes companies need to change propositions. To do this, companies should own a suitable governance, good knowledge of customers, marketing tools, capability to listen to market's requirements”. The match between human component and data-driven approach is synthesized in the claim of the company guided by interviewee F1: “Think human, act digital”.
One of the interviewees (M1, knowledge manager) affirms that “the creation of an internal laboratory of innovation can transform the organization into a “place” of analysis in which disruptive solutions can emerge and challenges and crisis can be managed”. Even in this case, according to M1, the capabilities to interpret data can generate innovative outcomes: “we enter companies for a marathon of business analysis, just like for hackathon: for two or more days a real task force of professionals sit around a table together with the internal team of the firms to listen, analyse and design the solution for the development of innovation”.
Not only scientific knowledge is shared to develop new hard skills for future employees but the companies in the sample also “try to create a digital workplace to train sensitive and emotional people able to imagine and to live digital era harmonically and to connect people that would never have met otherwise (interviewee M2, digital strategist and storyteller)”. Therefore, data-driven innovation implies a cultural revolution that overcomes technological innovation and gives birth to innovative educational practices. In the sample, educational innovation is developed through the constant improvement of learning processes and through the transformation of the individual skills and competencies into organizational knowledge, which is integrated with external knowledge deriving from other firms and institutions, such as universities and research centres.
In the description of the education and training programmes implemented in his firm, interviewee M4 (start-up mentor and entrepreneurship educator) affirms: “we work for the people that tomorrow will develop high social impact solutions by working on the emotional structure of youngsters: tomorrow's leaders should cross new boundaries more and more rapidly”. Through open innovation projects, according to M4 the mentorship is bidirectional: “leaders share with youngsters their weaknesses, whereas talents will provide them with energy, vision, imagination, to build relationships that can co-create value for our territories”. Strictly related to educational innovation is the investment in youth entrepreneurship, a common practice in the sample. More than 100 youngsters are “recruited” to provide them with coaching experiences to enrich not only their hard skills, but also soft skills such as “entrepreneurial attitude, which should be enhanced to create a new generation of entrepreneurs, since in contemporary organizations people without entrepreneurial capabilities are not relevant and cannot survive”.
Educational innovation “is not just a radical idea, but an innovative practice, the effective and sustainable application of a new idea of corporate culture and training (interviewee C3)”.
The companies in the sample combine their social/sustainable goals with human resources practices and, through the active participation of organizational members, enable the creation of new forms of social participation and educational models. The relationship between human resources, sustainability and social outcomes is emphasized by interviewee F3: “our ability to be effective is enhanced through the optimal use of resources to achieve social goals, to demonstrate that new ideas coming from the enrichment of skills work better than existing solutions and generate value for society”. As declared by interviewee F5, “social value is created by impacting on people's growth paths in crisis periods, by providing the ecosystem with a series of capabilities and tools to connect them with stimuli and opportunities”.
Lastly, “cultural change” should be realized, since often organizations do not own the right culture to transform their businesses. To enhance transformation, the path is slow and human resources should be trained. For this reason, some entrepreneurs in the sample organize student's traineeship in their firms. However, as declared by interviewee M4 “traineeship is not enough to make the change: that's why we tried with reverse mentorship (students and talents that become mentors of senior employees) [..] but some people are not inclined to change: if seniors have resistance to change, the experiment does not work, but even if seniors have open mind, skills, history they do not have necessarily the ‘culture' of the future”. Therefore, reverse mentorship allows the evolution of companies' culture and the continuous exchange of knowledge, skills, and experiences among different actors. In this way, new meanings, rules, and practices are created that strengthen the sense of belonging to a community and generate and regenerate its culture.
Figure 7 depicts the key findings obtained to address RQ2 and to identify the different types of innovation generated in the data-driven companies in the sample.
5. Discussion
The categorization of the different strategies, goals, activities, resources, technologies implemented by the entrepreneurs in the sample and described in the findings contributes to classify the main enablers of data-driven approach for the development of innovation (RQ1).
Lastly, thanks to data-driven orientation and the synergy between hard and soft skills, which are integrated to create and co-create knowledge, new and innovation can be co-developed, which can give birth to different innovation modalities and outcomes (RQ2).
The results obtained confirm that the complementarity and synthesis between data analysis/management and the promotion of human resources/skills can boost the emergence of innovation. The different categories, dimensions and sub-dimensions identified through the criteria of Gioia methodology are depicted in Figure 8.
Regarding RQ1, the interpretation of the interviews allows the categorization and conceptualization of the enabling dimensions of innovation empowered by data-driven orientation.
Data-driven orientation implies the spreading of a culture (dimension 1) based on learning and data as strategic assets, on the adoption of a proactive orientation to innovation and on the relevance of human resources. This multi-dimensional culture should be translated into a given technological infrastructure based on multiple touchpoints (cloud computing systems, DSS, fuzzy logic, Agile work system) that needs to be “activated” by the constant exchange of resources between people (dimension 2) that encourages, in turn, the empowerment of human resources (dimension 3) through distributed teams and engagement strategies. The proper activation of the technological infrastructure performed through the sharing of resources and the renewal of human abilities can lead to constant renewal of employees' management and research skills (hard skills) and of proactive attitude and creativity (softs skills).
The internalization of data-driven culture and the effective use of digital technologies mediated through human intervention and knowledge exchange can enhance process management based on distributed decision-making (dimension 5), by increasing companies' ability to increase know-how, to manage and improve data exchange, by extracting value from new knowledge.
The synergistic combination of learning culture (Migdadi et al., 2021; Steiber et al., 2020), resources integration and skills enhancement through distributed managerial processes based on a strategic technological architecture can lead to the constant renewal of value and to continuous improvement (dimension 6) that can foster the emergence of innovation over time.
Then, starting from the activation of data-driven strategies and practices, different innovation outcomes can be generated (RQ2). The findings reveal that the entrepreneurs in the sample co-create innovation constantly (and develop continuous improvement and systematic innovation) by generating different types of innovation, which envelop different dimensions (organizational, technological, human, cultural and social). Six different types of innovation have been derived.
Product/process innovation is intended as optimization, a basic form of innovation at the lowest degree of novelty, which leads the introduction of new products/services and can simplify the information flows throughout the supply chain. Business model innovation stems from the application of human skills based on data-driven mind-set, which develops a new proactive orientation that reframes business goals and generates new value propositions, strategies, competencies, knowledge exchange modalities and relational management strategies. Social innovation is connected to the creation of well-being for the entire ecosystem of people (managers, employees, customers but also citizens and community as a whole) and to the co-development of sustainable innovative solutions to generate multiple benefits (Visvizi et al., 2018a; Polese et al., 2018). Educational innovation refers to the harmonization of knowledge integration through open innovation projects and mentorship that improve scientific innovation to create knowledge for people's growth enhancement and the provision of job opportunities (Visvizi et al., 2018b). Cultural change implies the introduction of new practices, meanings, rules and symbols that lead to the actors-driven diffusion of a cohesive culture for organizations, citizens, institutions based on a new proactive-innovation oriented attitude grounded on the relevance of data and human components.
6. Conceptual and managerial implications
The results highlight the enabling factors for innovation and the most proper strategies to exploit the opportunities offered by technology/human enabled business processes, by detecting some knowledge exchange strategies to pursue innovation.
In this way, this paper responds to the call for research introduced in extant literature for the development of studies that would identify and explore the key enabling dimension of data-driven innovation (Trabucchi and Buganza, 2019). Moreover, the study offers an insight into the conceptualization of innovation as a multi-level construct integrating technological, human and managerial processes. These are necessary for the emergence of novelty, or innovation, conceived of as a multi-varied concept, i.e. involving technological and non-technological outcomes. This article contributes to the discussions on data-driven orientation and innovation by showing that data-driven companies, pursuing learning orientation, can not only undertake more effective decisions, but also develop more flexible strategies, processes, and business models (Troisi et al., 2020) (RQ1). The conceptual framework employed in this study clarifies the relationship between data-driven orientation and the emergence of different types of innovation (RQ2). It thus detects how different data management strategies and human skills can produce different innovative outcomes.
The classification of the main enabling dimensions of innovation and of the different types of innovation that data-driven companies can co-develop with their stakeholders enhance researcher's and practitioners' understanding of the complex and multi-variate concept of innovation (Kahn, 2018). This multi-varied conceptualization of innovation, i.e. a conceptualization that integrates the technological, human, institutional/organizational and managerial factors, offers an invaluable insight into: (1) how data, and subsequently information and knowledge, can foster the development of different innovation outcomes; (2) how data analysis strategies and technologies can produce new value; (3) how the agential skills and capacities impact the process of innovation emergence in organizations. In brief, this paper reveals the relationship between a data-driven use of ICT, human resources management and innovation has been clarified. Consequently, managers can collect some insights on the proper combination of data management strategies and human resources management to encourage the transformation of meaningless data into relevant knowledge and stimulate, in turn, the harmonization of complex innovation processes. The empirical research offers some insights on “how” human-mediated data analysis can empower the dynamic integration of skills through constant process of adaptation and reconfiguration of previous knowledge by identifying the main drivers for continuous improvement. In addition, the findings of the study can show management how, through the association of different data-driven strategies, targeted practices to enhance innovation can be identified. Lastly, management can understand how the innovative outcomes introduced can be renewed over time to pursue continuous improvement. In this way, innovation is conceptualized both as a systems process (Troise et al., 2020), which embeds human, cultural and social dimensions, and a systematic process, based on the establishment of a tension to innovation and of a transformative attitude.
7. Conclusions
The work shows how the strategic integration between digital and human component and the adoption of data-driven mind-set can allow the attainment of new knowledge and boost the emergence of co-created innovation. Then, systems and systematic innovation can be pursued based on continuous improvement, on the attainment of growth and on the synergistic integration of multiple innovation outcomes for multiple stakeholders throughout multiple business layers.
The use of big data analytics cannot guarantee the emergence of innovation because human skills, abilities and knowledge are required to activate the capability to use data in view of creating and co-creating value. Exploring the potential connection between the adoption of data-driven orientation, skills enhancement and the development of innovation can help the identification of the different strategic levers for the exploitation of the opportunities offered from technologies and for the promotion of innovation. On the one hand, the study shows that human component can act as one of the main drivers for the proper application of a strategic data management for the creation of entrepreneurial opportunities. On the other hand, the analysis of the key enabling dimensions of data-driven orientation can reveal the most useful strategies for the enrichment of people's skills (Scott and Le Lievre, 2019) to foster the emergence of capabilities which can develop, in turn, opportunities for innovation.
The limitations of the study include all the methodological issues related to adoption of case study methodology, which does not permit the extension of the findings obtained to other contexts. For this reason, to assess the generalizability of the framework, comparative case studies can be performed. Future studies can start from the results proposed in the study to apply the classification of the different types of innovation to specific industries and/or contexts (e.g. healthcare, territory, clusters). Further research on this topic can confirm the validity of the dimensions conceptualized in this research through the application of measurement scale. Mixed methodology, which integrates quantitative and qualitative methods, can be employed to assess the existence of the different types of innovation through quantitative techniques such as multiple regression or structural equation modelling. In this way, the relationships between the dimensions of data-driven decision orientation and the innovative outcomes can be tested and related to other constructs such as actor's engagement, users' behaviour, and/or value co-creation.
Figures
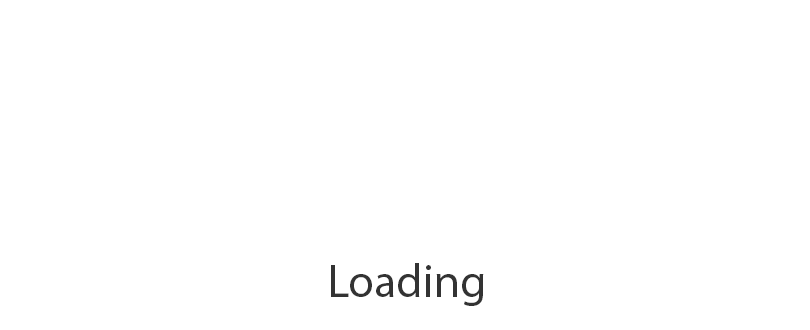
Figure 6
The different steps of Gioia Methodology through content analysis: findings of RQ1
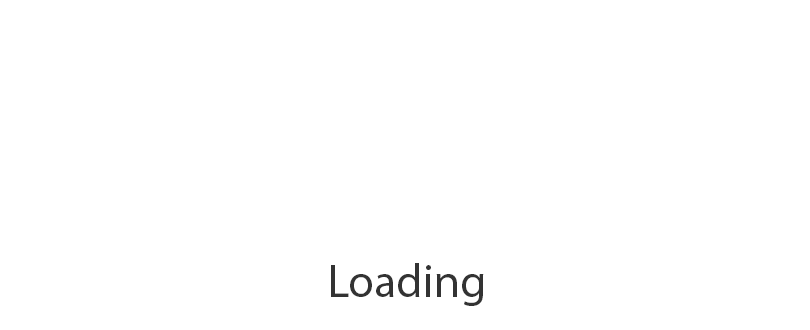
Figure 7
The different steps of Gioia methodology through content analysis: findings of RQ2
Description of the sample
Job position | Sex | Age | City/Region | Code | |
---|---|---|---|---|---|
1 | Start-up co-founder | Male | 26 | Salerno, Campania | F1 |
Digital Life Coach digital Manager | |||||
Digital Consultant; Web site designer | |||||
2 | Start-up owner | Male | 22 | Catania, Sicily | F2 |
Digital consultant Social Media strategist | |||||
3 | Founder non-profit company | Male | 25 | Milan, Lombardia | F3 |
Digital consultant; Social Media coach | |||||
4 | Marketing consultant | Male | 35 | Bari, Apulia | C1 |
Start-up co-founder (digital company) | |||||
5 | Knowledge manager for a digital company | Female | 24 | Bologna, Emilia | M1 |
6 | Senior Advisor for a digital company | Male | 36 | Turin, Piemonte | C2 |
Founder of Growth Hacking day | |||||
Start-up coach and consultant | |||||
7 | Digital strategist; Storyteller | Male | 29 | L'Aquila, Abruzzo | M2 |
8 | Start-up founder (design); Start-up mentor | Male | 35 | Cosenza, Calabria | F4 |
9 | Founder of start-up (social enterprise) | Female | 28 | Genoa, Liguria | F5 |
Researcher in marketing | |||||
10 | Founder start-up (food-tech) | Female | 32 | Olbia, Sardinia | F6 |
11 | Founder start-up (informatics) | Male | Treviso, Veneto | F7 | |
12 | Associate Professor in Marketing | Male | 30 | Isernia, Molise | C3 |
Digital marketing consultant | |||||
13 | Digital entrepreneur | Male | 22 | Potenza, Basilicata | M3 |
14 | Start-up founder; Management consultant | Female | 28 | Udine, Friuli | F8 |
15 | Entrepreneurship education; Start-up mentor | Male | 38 | Aosta, Valle D'Aosta | M4 |
16 | Innovation consultant | Male | 24 | Perugia, Umbria | C4 |
Business and Innovation Designer | |||||
Marketing Analyst | |||||
17 | Digital strategies; Web site developer | Male | 28 | Trento, Trentino Alto Adige | M5 |
Co-founder start-up (informatics) | |||||
18 | Business analyst | Male | 28 | Rome, Lazio | M6 |
Open innovation manager | |||||
19 | Start-up founder; Business coach | Male | 32 | Ascoli, Marche | F9 |
Supply chain and operation manager | |||||
20 | Innovation Manager | Female | 38 | Grosseto, Toscana | M7 |
Co-founder start-up (tourism) |
Source(s): Author's elaboration
Interview sketch
Data-driven decision- making | |
TechnologicaI dimension | What are the technological tools and/or analytics used to collect and analyze data? How are these used to meet the main strategic objectives of your company (e.g. users and/or buyer personas profiling strategies)? |
Human dimension | Is there a strategic planning for data collection, fot the selection of sources and for the extraction and interpretation of results in your company? |
What are the most appropriate skills to manage the collection and analysis of data? How can the human component enhance or hinder effective data analysis and interpretation? | |
Is there an integrated strategv of actions for the continuous training of employees and/or for the enhancement of skills? | |
Managerial dimension | Have you ever had to revise уour decisions in progress based on the results of data interpretation? And after the dialogue with oder employees inside and outside your company? Which of the two phenomena occurred most frequently? |
How are the different members of your company (at each level) involved in business decisions? And in which stage of the decision-making process (problem identification, alternative selection, search for the solution, choice of actions to be taken)? | |
Is the data collected and the results of data interpretation shared within the organization, and “stored”? Which is the role of innovation for the survival of your company? | |
Innovation | |
Technology-driven | Do you think that your activity can contribute to the development of technological advances, of innovative products and services in the industry and of a new way of doing business digitally? If so, how? |
How does your company search for innovation opportunities and try to forecast market's requirement? | |
Кnowledge-based | In your opinion, how much does the constant updating of skills contribute to the development of innovation? How can the participation and the exchange of skills with and among the members of your company support the development of business opportunities and the emergence of innovation? |
Is there an integrated strategy of actions aimed at achieving goals related to the well-being of individuals (social inclusion, equal opportunities, e-democracy)? | |
Social-oriented | How does sustainability core values are diffused in the company? |
Appendix
References
Anand, N., Gardner, H.K. and Morris, T. (2007), “Knowledge-based innovation: emergence and embedding of new practice areas in management consulting firms”, Academy of Management Journal, Vol. 5 No. 2, pp. 406-428.
Baden-Fuller, C. and Haefliger, S. (2013), “Business models and technological innovation”, Long Range Planning, Vol. 46 No. 6, pp. 419-426.
Bashir, M. and Farooq, R. (2019), “The synergetic effect of knowledge management and business model innovation on firm competence: a systematic review”, International Journal of Innovation Science, Vol. 11 No. 1, pp. 1757-1785.
Beliaeva, T., Ferasso, M., Kraus, S. and Damke, E.J. (2019), “Dynamics of digital entrepreneurship and the innovation ecosystem: a multilevel perspective”, International Journal of Entrepreneurial Behavior and Research, Vol. 26 No. 2, pp. 266-284.
Bonfanti, A., Castellani, P., Giaretta, E. and Federico, B. (2019), “Developing entrepreneurial learning triggered by factory tours”, The Learning Organization, Vol. 26 No. 6, pp. 574-587.
Bouwman, W.A.G.A., Nikou, S., Molina-Castillo, F.J. and de Reuver, G.A. (2018), “The impact of digitalization on business models”, Digital Policy, Regulation and Governance, Vol. 20 No. 2, pp. 105-124.
Brynjolfsson, E., Hitt, L.M. and Kim, H.H. (2011), “Strength in numbers: how does data-driven decision- making affect firm performance?”, Working Paper, Sloan School of Management, MIT, Cambridge, MA, April 22.
Carayannis, E., Del Giudice, M., Saviano, M. and Caputo, F. (2018), “Beyond Big Data: from smart to wise knowledge management”, in Barile, S., Espejo, R., Perko, I. and Saviano, M. (Eds), Cybernetics and Systems: Social and Business Decisions, Giappichelli-Routledge, London, pp. 261-267.
Castellani, P., Bonfanti, A., Canestrino, R. and Magliocca, P. (2020), “Dimensions and triggers of memorable tourism experiences: evidence from Italian social enterprises”, The TQM Journal, Vol. 32 No. 6, pp. 20201115-1138.
Ceci, F., Masciarelli, F. and Poledrini, S. (2019), “How social capital affects innovation in a cultural network: exploring the role of bonding and bridging social capital”, European Journal of Innovation Management, Vol. 23 No. 5, pp. 895-918.
Chaffey, D. and Patron, M. (2012), “From web analytics to digital marketing optimization: increasing the commercial value of digital analytics”, Journal of Direct, Data and Digital Marketing Practice, Vol. 14 No. 1, pp. 30-45.
Charmaz, K. (2006), Constructing Grounded Theory: A Practical Guide Through Qualitative Analysis, Sage, Thousand Oaks, CA.
Chen, H., Chiang, R.H. and Storey, V.C. (2012), “Business intelligence and analytics: from big data to big impact”, MIS Quarterly, Vol. 36 No. 4, pp. 1165-1188.
Chen, D.Q., Preston, D.S. and Swink, M. (2015), “How the use of big data analytics affects value creation in supply chain management”, Journal of Management Information Systems, Vol. 32 No. 4, pp. 4-39.
Chesbrough, H. (2007), “Business model innovation: it's not just about technology anymore”, Strategy and Leadership, Vol. 35 No. 6, pp. 12-17.
Corbetta, P. (2003), Social Research: Theory, Methods and Techniques, Sage, New York, NY.
Crossan, M.M. and Apaydin, M. (2010), “A multi‐dimensional framework of organizational innovation: a systematic review of the literature”, Journal of Management Studies, Vol. 47 No. 6, pp. 1154-1191.
Damanpour, F. (2014), “Footnotes to research on management innovation”, Organization Studies, Vol. 35 No. 9, pp. 1265-1285.
Denford, J.S. (2013), “Building knowledge: developing a knowledge‐based dynamic capabilities typology”, Journal of Knowledge Management, Vol. 17 No. 2, pp. 175-194.
Dougherty, D. (2004), “Organizing practices in services: capturing practice-based knowledge for innovation”, Strategic Organization, Vol. 2 No. 1, pp. 35-64.
Dworkin, S.L. (2012), “Sample size policy for qualitative studies using in-depth interviews”, Archives of Sexual Behavior, Vol. 41, pp. 1319-1320.
Fichman, R.G., Dos Santos, B.L. and Zheng, Z.E. (2014), “Digital innovation as a fundamental and powerful concept in the information systems curriculum”, MIS Quarterly, Vol. 38 No. 2, pp. 329-345.
Gioia, D., Corley, K. and Aimee, H. (2013), “Seeking qualitative rigor in inductive research: notes on the Gioia methodology”, Organizational Research Methods, Vol. 16 No. 1, pp. 15-31.
Glaser, B.G. (2007), “Constructivist grounded theory?”, Historical Social Research/Historische Sozialforschung, Vol. 19 Supplement, pp. 93-105.
Gupta, M. and George, J.F. (2016), “Toward the development of a big data analytics capability”, Information and Management, Vol. 53 No. 8, pp. 1049-1064.
Harrisson, D., Bourque, R. and Széll, G. (2009), Social Innovation, Economic Development, Employment and Democracy, Peter Lang, Frankfurt am Main.
Henao-García, E.A. and Montoya, R.A.C. (2021), “Fostering technological innovation through management and marketing innovation. The human and non-technological linkage”, European Journal of Innovation Management, Vol. ahead of print No. ahead of Print, doi: 10.1108/EJIM-03-2021-0148 (in press) (accessed 14 July 2021).
Hipp, C. and Grupp, H. (2005), “Innovation in the service sector: the demand for service-specific innovation measurement concepts and typologies”, Research Policy, Vol. 34 No. 4, pp. 517-535.
Howaldt, J., Kaletka, C. and Schröder, A. (2016), “Social entrepreneurs: important actors within an ecosystem of social innovation”, European Public and Social Innovation Review, Vol. 1 No. 2, pp. 95-110.
Järvinen, J. and Karjaluoto, H. (2015), “The use of Web analytics for digital marketing performance measurement”, Industrial Marketing Management, Vol. 50, pp. 117-127.
Kahn, K.B. (2018), “Understanding innovation”, Business Horizons, Vol. 61 No. 3, pp. 453-460.
Kusiak, A. (2009), “Innovation: a data-driven approach”, International Journal of Production Economics, Vol. 122 No. 1, pp. 440-448.
LaValle, S., Lesser, E., ShockleyRebecca, H.M. and Krushcwitz, N. (2011), “Big data, analytics and the path from insights to value”, MIT Sloan Management Review, Vol. 52 No. 2, p. 21.
Lee, S.M. and Trimi, S. (2018), “Innovation for creating a smart future”, Journal of Innovation and Knowledge, Vol. 3 No. 1, pp. 1-8.
Lee, J., Kao, H.A. and Shanhu, Y.S. (2014), “Service innovation and smart analytics for industry 4.0 and big data environment”, Procedia Cirp, Vol. 16 No. 1, pp. 3-8.
Lepri, B., Staiano, J., Sangokoya, D., Letouzé, E. and Oliver, N. (2017), “The tyranny of data? The bright and dark sides of data-driven decision making for social good”, in Transparent Data Mining for Big and Small Data, Springer, Cham, CH, pp. 3-24.
Lewis, J., Wright, P.C. and Geroy, G.D. (2004), “Managing human capital”, Management Decision, Vol. 4 No. 2, pp. 205-228.
Loia, F., Capobianco, N. and Vona, R. (2021), “Towards a resilient perspective for the future of offshore platforms. Insights from a data driven approach”, Transforming Government: People, Process and Policy, Vol. ahead-of-print No. ahead-of-print, doi: 10.1108/TG-04-2021-0067 (in press) (accessed 8 September 2021).
Malik, R., Visvizi, A. and Skrzek-Lubasińska, M. (2021), “The gig economy: current issues, the debate, and the new avenues of research”, Sustainability, Vol. 13 No. 2021, p. 5023, doi: 10.3390/su13095023.
Manyika, J., Chui, M., Brown, B., Bughin, J., Dobbs, R., Roxburgh, C. and Byers, A.H. (2011), Big Data: The Next Frontier for Innovation, Competition and Productivity, McKinsey Global Institute, New York, NY.
Martín-de-Castro, G., Delgado-Verde, M., López-Sáez, P. and Navas-López, J.E. (2011), “Towards 'an intellectual capital-based view of the firm': origins and nature”, Journal of Business Ethics, Vol. 98 No. 4, pp. 649-662.
McAfee, A. and Brynjolfsson, E. (2012), “Big data: the management revolution”, Harvard Business Review, Vol. 90, pp. 60-66.
Medina-Borja, A. (2019), “Embedding humans into service systems analysis: the evolution of mathematical thinking about services”, in Handbook of Service Science, Vol. 2, pp. 743-771.
Mele, C. and Polese, F. (2011), “Key dimensions of service systems: Interaction in social & technological networks to foster value co-creation”, The Science of Service Systems, Springer, New York, NY, pp. 37-59.
Migdadi, M.M. (2021), “Organizational learning capability, innovation and organizational performance”, European Journal of Innovation Management, Vol. 24 No. 1, pp. 151-172.
Mills, J., Bonner, A. and Karen, F. (2006), “The development of constructivist grounded theory”, International Journal of Qualitative Methods, Vol. 5 No. 1, pp. 25-35.
Morse, J.M. (2000), “Determining sample size”, Qualitative Health Research, Vol. 10, pp. 3-5.
Mothe, C. and Nguyen, T.T.U. (2010), “The impact of non-technological innovation on technical innovation: do services differ from manufacturing? An empirical analysis of Luxembourg firms”, Ceps Instead, Working paper, 10.
Nag, R. and Gioia, D.A. (2012), “From common to uncommon knowledge: foundations of firm-specific use of knowledge as a resource”, Academy of Management Journal, Vol. 55 No. 2, pp. 421-457.
Nieto, M. (2004), “Basic propositions for the study of the technological innovation process in the firm”, European Journal of Innovation Management, Vol. 7 No. 4, pp. 314-324.
OECDEurostat (2005), Oslo Manual - Proposed Guidelines for Collecting and Interpreting Technological Innovation Data, OECD, Paris.
Perri, C., Giglio, C. and Corvello, V. (2020), “Smart users for smart technologies: investigating the intention to adopt smart energy consumption behaviors”, Technological Forecasting and Social Change, Vol. 155, p. 119991.
Polese, F., Botti, A., Grimaldi, M., Monda, A. and Vesci, M. (2018), “Social innovation in smart tourism ecosystems: how technology and institutions shape sustainable value co-creation”, Sustainability, Vol. 10 No. 1, p. 140.
Quintane, E., Casselman, R.M., Reiche, B.S. and Nylund, P.A. (2011), “Innovation as a knowledge‐based outcome”, Journal of Knowledge Management, Vol. 15 No. 6, pp. 928-947.
Rachinger, M., Rauter, R., Müller, C., Vorraber, W. and Schirgi, E. (2019), “Digitalization and its influence on business model innovation”, Journal of Manufacturing Technology Management, Vol. 30 No. 8, pp. 1143-1160, doi: 10.1108/JMTM-01-2018-0020.
Saeedi, K. and Visvizi, A. (2021), “Software development methodologies, HEIs, and the digital economy”, Education Science, Vol. 11 No. 2, p. 73, doi: 10.3390/educsci11020073.
Schmidt, T. and Rammer, C. (2007), “Non-technological and technological innovation: strange bedfellows?”, ZEW-Centre for European Economic Research Discussion Paper, (07-052).
Scott, R. and Le Lievre, M. (2019), “Welcome to the marriage of human capability and artificial intelligence”, Strategic HR Review, Vol. 19 No. 1, pp. 10-14.
Shujahat, M., Sousa, M.J., Hussain, S., Nawaz, F., Wang, M. and Umer, M. (2019), “Translating the impact of knowledge management processes into knowledge-based innovation: the neglected and mediating role of knowledge-worker productivity”, Journal of Business Research, Vol. 94, pp. 442-450.
Smart, P., Bessant, J. and Gupta, A. (2007), “Towards technological rules for designing innovation networks: a dynamic capabilities view”, International Journal of Operations and Production Management, Vol. 27 No. 10, pp. 1069-1092.
Snyder, H., Witell, L., Gustafsson, A., Fombelle, P. and Kristensson, P. (2016), “Identifying categories of service innovation: a review and synthesis of the literature”, Journal of Business Research, Vol. 69 No. 7, pp. 2401-2408.
Sousa, M.J. and Rocha, Á. (2019), “Skills for disruptive digital business”, Journal of Business Research, Vol. 94, pp. 257-263.
Spender, J.C., Corvello, V., Grimaldi, M. and Rippa, P. (2017), “Startups and open innovation: a review of the literature”, European Journal of Innovation Management, Vol. 20 No. 1, pp. 4-30.
Steiber, A., Alänge, S. and Corvello, V. (2020), “Learning with startups: an empirically grounded typology”, The Learning Organization, Vol. 28 No. 2, pp. 153-166, doi: 10.1108/TLO-04-2020-0061.
Strauss, A. and Corbin, J. (1994), “Grounded theory methodology”, in Denzin, N.K. and Lincoln, Y.S. (Eds), Handbook of Qualitative Research, SAGE, Thousand Oaks, CA, pp. 273-285.
Teece, D.J. (2010), “Business models, business strategy and innovation”, Long Range Planning, Vol. 43 Nos 2-3, pp. 172-194.
Trabucchi, D. and Buganza, T. (2019), “Data-driven innovation: switching the perspective on Big Data”, European Journal of Innovation Management, Vol. 22 No. 1, pp. 23-40.
Troilo, G., De Luca, L.M. and Guenzi, P. (2017), “Linking data‐rich environments with service innovation in incumbent firms: a conceptual framework and research propositions”, Journal of Product Innovation Management, Vol. 34 No. 5, pp. 617-639.
Troise, C., Matricano, D. and Sorrentino, M. (2020), “Open Innovation Platforms: exploring the importance of knowledge in supporting online initiatives”, Knowledge Management Research and Practice, Vol. 13 No. 3, pp. 1-9.
Troisi, O., Grimaldi, M. and Loia, F. (2020), “Redesigning business models for data-driven innovation: a three-layered framework”, The International Research and Innovation Forum, Springer, Cham, pp. 421-435.
Troisi, O., Maione, G., Grimaldi, M. and Loia, F. (2019), “Growth hacking: insights on data-driven decision making from three firms”, Industrial Marketing Management, Vol. 90, pp. 538-557.
Troisi, O., Visvizi, A. and Grimaldi, M. (2021), “The different shades of innovation emergence in smart service systems: the case of Italian cluster for aerospace technology”, Journal of Business and Industrial Marketing, Vol. ahead of print No. ahead of print, doi: 10.1108/JBIM-02-2020-0091 (in press) (accessed 29 January 2021).
Utterback, J.M. and Abernathy, W.J. (1975), “A dynamic model of process and product innovation”, Omega, Vol. 3 No. 6, pp. 639-656.
Van der Have, R.P. and Rubalcaba, L. (2016), “Social innovation research: an emerging area of innovation studies?”, Research Policy, Vol. 45 No. 9, pp. 1923-1935.
Visvizi, A., Lytras, M., Damiani, E. and Hassan, M. (2018a), “Policy making for smart cities: innovation and social inclusive economic growth for sustainability”, Journal of Science and Technology Policy Management, Vol. 9 No. 2, pp. 126-133.
Visvizi, A., Lytras, M. and Linda, D. (2018b), “(Re)defining smart education: towards dynamic education and information systems for innovation networks”, in Lytras, M., Linda, D. and Visvizi, A. (Eds), Enhancing Knowledge Discovery and Innovation in the Digital Era, IGI Global, Hershey, PA, pp. 1-12.
Wamba, S.F., Akter, S., Edwards, A., Chopin, G. and Gnanzou, D. (2015), “How ‘big data' can make big impact: findings from a systematic review and a longitudinal case study”, International Journal of Production Economics, Vol. 165, pp. 234-246.
Wosiek, R. and Visvizi, A. (2021), “The VWRCA index: measuring a country's comparative advantage and specialization in services. The case of Poland”, Economies, Vol. 9, p. 48, doi: 10.3390/economies9020048.
Wu, S., Ding, X., Liu, R. and Gao, H. (2021), “How does IT capability affect open innovation performance? The mediating effect of absorptive capacity”, European Journal of Innovation Management, Vol. 24 No. 1, pp. 43-65.
Yin, R.K. (2011), Applications of Case Study Research, Sage, New York, NY.
Zhou, Z.H., Chawla, N.V., Jin, Y. and Williams, G.J. (2014), “Big data opportunities and challenges: discussions from data analytics perspectives”, IEEE Computational Intelligence Magazine, Vol. 9 No. 4, pp. 62-74.
Zhou, X., Cai, Z., Tan, K.H., Zhang, L., Du, J. and Song, M. (2021), “Technological innovation and structural change for economic development in China as an emerging market”, Technological Forecasting and Social Change, Vol. 167, p. 120671.