Abstract
Purpose
Robotic process automation (RPA) has recently emerged as a technology focusing on the automation of repetitive, frequent, voluminous and rule-based tasks. Despite a few practical examples that document successful RPA deployments in organizations, evidence of its economic benefits has been mostly anecdotal. The purpose of this paper is to present a step-by-step method to RPA investment appraisal and a business case demonstrating how the steps can be applied to practice.
Design/methodology/approach
The methodology relies on design science research (DSR). The step-by-step method is a design artefact that builds on the mapping of processes and modelling of the associated costs. Due to the longitudinal nature of capital investments, modelling uses discounted cashflow and present value methods. Empirical grounding characteristic to DSR is achieved by field testing the artefact.
Findings
The step-by-step method is comprised of a preparatory step, three modelling steps and a concluding step. The modelling consists of compounding the interest rate, discounting the investment costs and establishing measures for comparison. These steps were applied to seven business processes to be automated by the case company, Estate Blend. The decision to deploy RPA was found to be trivial, not only based on the initial case data, but also based on multiple sensitivity analyses that showed how resistant RPA investments are to changing circumstances.
Practical implications
By following the provided step-by-step method, executives and managers can quantify the costs and benefits of RPA. The developed method enables any organization to directly compare investment alternatives against each other and against the probable status quo where many tasks in organizations are still carried out manually with little to no automation.
Originality/value
The paper addresses a growing new domain in the field of business process management by capitalizing on DSR and modelling-based approaches to RPA investment appraisal.
Keywords
Citation
Ylä-Kujala, A., Kedziora, D., Metso, L., Kärri, T., Happonen, A. and Piotrowicz, W. (2023), "Robotic process automation deployments: a step-by-step method to investment appraisal", Business Process Management Journal, Vol. 29 No. 8, pp. 163-187. https://doi.org/10.1108/BPMJ-08-2022-0418
Publisher
:Emerald Publishing Limited
Copyright © 2023, Antti Ylä-Kujala, Damian Kedziora, Lasse Metso, Timo Kärri, Ari Happonen and Wojciech Piotrowicz
License
Published by Emerald Publishing Limited. This article is published under the Creative Commons Attribution (CC BY 4.0) licence. Anyone may reproduce, distribute, translate and create derivative works of this article (for both commercial and non-commercial purposes), subject to full attribution to the original publication and authors. The full terms of this licence may be seen at http://creativecommons.org/licences/by/4.0/legalcode
1. Introduction
We live in a world saturated with digital technologies. There always seems to be a new technological buzzword around the corner; big data, Internet of things, cloud computing, artificial intelligence, machine learning, data mining, blockchain or robotic process automation. In recent years, robotic process automation (RPA) has been generating interest in businesses and academia alike. RPA belongs to a family of intelligent automation (IA) technologies designed to automate business processes from the simplest rule-based tasks to increasingly adaptive judgment-based decisions that augment human intellectual capabilities (Viehhauser, 2020; Ng et al., 2021). Alongside other IA technologies, RPA has the potential to increase agility in business process management by initiating rapid business process changes in organizations following the virtues of both leanness and flexibility (Badakhshan et al., 2020; Schmitt and Hörner, 2021).
IA solutions are typically differentiated in terms of each technology's cognitive processing capabilities and ability to handle process complexity (Lacity and Willcocks, 2021). As software robots are often configured to follow a predetermined choreography (Hofmann et al., 2020), traditional RPA is relatively easy to adopt compared to other IA technologies, such as machine learning which demands much higher process complexity. As Willcocks et al. (2015) have aptly phrased, a software robot is “an infinitely scalable virtual human being that can be instructed to carry out operational procedures at the speed of a machine”. Tasks suitable for RPA are ideally repetitive, frequent, voluminous and highly standardized (Santos et al., 2020). As they are based on simple rules and logic with a limited need for exception handling, the automation of such processes releases humans to more cognitively challenging tasks (Mendling et al., 2018).
As a relatively new technology, RPA has been adopted mostly by businesses and other organizations, while academic research on the topic is still underdeveloped, although the number of publications has been steadily increasing in recent years (Syed et al., 2020). As it stands, the empirical research is founded on case studies that focus on specific topics, such as how RPA can be implemented into the existing organizational IT infrastructure (Carden et al., 2019), RPA governance models (Kedziora and Penttinen, 2020) and organizational change management when automation affects employees' job descriptions (Dunlap and Lacity, 2017). As software robots can process large amounts of transaction data with relative ease, the studies show an emphasis on accounting (Cooper et al., 2019) and auditing (Huang and Vasarhelyi, 2019), thus continuing the on-going trend of digitalization of accounting as a field and profession (Knudsen, 2020).
Regardless of the recent interest and developments in RPA, the literature is lacking proper discussion on RPA investments from implementation considerations to associated costs and economic benefits by means of systematic methods and models for RPA investment appraisal. Despite many examples from accounting, auditing and other practical application contexts and domains, the questions remain: “How feasible is RPA as an investment?” and “What needs to be considered when RPA is deployed in an organization?” The answers to these questions should be of interest not only to academics, but to any executive or manager seeking to adopt the technology.
A good approach to evaluating the adoption of technology is to conduct an investment appraisal. Aside from a few anecdotal notions of RPA projects having excellent ROI, i.e. return on investment (van der Aalst et al., 2018), reported to be as high as 600–850% (Lacity and Willcocks, 2016), quantifiable data of such benefits is largely missing. As the assessment of the economic impact of technology has been challenging to organizations in the past (Berghout et al., 2011) and identifying and quantifying the benefits of information technology investments is extremely difficult without systematic approaches (Ward et al., 2008), it is important to have purpose-built tools for RPA investment appraisal, such as frameworks and models. Consulting businesses have their own calculators and guidelines for RPA implementation (e.g. Team IM, 2021), but typically these are produced as marketing material for promoting service sales.
Inspired by the existent research gaps, the objective of the study is to obtain a toolbox for assessing the economic feasibility of RPA investments. In this paper, this type of toolbox is introduced in the form of the following step-by-step method to RPA investment appraisal.
- (1)
Preparatory Step: Establish a consistent system of classification (i.e. apply a process framework to map the phases, stages, tasks and other project elements). Use and adapt a deployment/implementation framework to your phasing, staging and tasking needs.
- (2)
Modelling Steps: Track and trace all project costs and when they occur. Attach these costs to the phases, stages, tasks and other cost-incurring project elements. Model, assess and iterate when necessary. Use the following steps:
Step 1: Compound the Interest Rate
Step 2: Discount the Investment Costs
Step 3: Establish Measures for Comparison
- (3)
Concluding Step: Compare the investment against the status quo. Remember to also conduct sensitivity analyses, especially on variables that are the least conservative estimates.
- (4)
Optional Step: Recruit a service partner, such as a consultant or service provider, to assist in RPA solution development including, e.g. the coding, maintaining and hosting of software robots.
The process through which such a method can be extracted is known as design science research (DSR), where practical problems are addressed by creating general knowledge in the form of design artefacts. The step-by-step method is a design artefact that is empirically grounded with a business case of a company known as “Estate Blend” (see Section 2 for details).
The step-by-step method is a DSR artefact that consists of two essential components. First, a deployment framework is established (see 1. Preparatory Step). The implementation and deployment considerations associated with RPA projects (i.e. phases, stages and tasks) are thoroughly examined with the intent of creating a consistent system of classification to support the modelling. Second, a model for appraising RPA investments is constructed, which should be understood as the backbone of our step-by-step method (see 2. Modelling Steps). Aligned with the deployment framework, the model observes RPA investments from initial planning through deployment to eventual substitution. The costs incurred within each phase, stage and task are determined and calculated with discounted cashflow and present value methods employing compounded interest rates for asymmetrical periodic lengths. By doing so, we can establish measures to compare the cost of automation against the cost of manual processing.
It should be noted that the deployment framework and the RPA investment model address a call by Syed et al. (2020) for research on RPA methodologies and benefits, more precisely methodological support for implementation, support for benefit realization and comprehensive metrics for benefits. Comparisons and sensitivity analyses complement the previous steps by assessing how assumptions influence key metrics in the model (see 3. Concluding Step). Sensitivity analyses enable the evaluation of obtained results. As the Estate Blend's case will demonstrate, having a service partner may enhance the overall process (see 4. Optional Step).
The paper is structured as follows:
Section 2 outlines the DSR-driven research design underlying the paper;
Section 3 discusses appraisal and deployment considerations related to RPA;
Section 4 exemplifies RPA deployment phasing, staging, and tasking through frameworks;
Section 5 introduces case company Estate Blend's journey towards automation;
Section 6 demonstrates how RPA investment appraisal was conducted at Estate Blend;
Section 7 discusses our step-by-step method, provides recommendations to practice, reflects on both the lessons learned and our contributions and evaluates the DSR artefact; and
Section 8 concludes the paper by highlighting further research avenues.
2. Research design
Methodologically, the development process of the step-by-step method to RPA investment appraisal is based on the principles of DSR. DSR is concerned with developing “general knowledge” to support the design of solutions to “field problems”, while specific knowledge and specific problems remain as the domain of practitioners (van Aken, 2004; Hevner et al., 2004). General knowledge in DSR can be formulated as “design artefacts”, i.e. human-made objects that are applicable as solutions to practical problems (Johannesson and Perjons, 2014). Design artefacts can be expressed, e.g. in the form of design exemplars that demonstrate how an outcome can be reached through certain actions by following the basic logic of “if you want to achieve Y in situation Z, then perform (something like) action X” (van Aken, 2005).
The step-by-step method is a design artefact that relies on analytic modelling using relatively simple mathematical equations to account for RPA investment costs and process mapping to establish a deployment framework that complements the model by providing a system of classification to be followed. Models are important in research as they imply systematic organization of, and relationships between, concepts (Ghauri and Grønhaug 2002). In this paper, such organization and relationships take place in relation to investment phasing, staging and tasking that are established as a framework prior to modelling. The artefact should be understood as a design exemplar that each practitioner can adapt to fit their specific situation, as RPA investments are discussed through a set of universal guidelines, “a step-by-step method”.
In DSR, the research process is commonly presented as cyclical. Hevner (2007), for example, argues that there are three cycles: design, relevance and rigour. The design is the primary cycle where an initial design concept is refined into a final product (i.e. a design artefact) through multiple rounds of production and testing. The relevance cycle refers to the underlying problem domain that establishes a context for an artefact to be empirically grounded and field tested. The rigour cycle, on the other hand, integrates the artefact into the existing knowledge base as it is important to know how your contribution relates to prior (academic) knowledge and applications.
A simplified process of building the model as a design artefact is illustrated in Figure 1. In terms of Hevner's (2007) DSR cycles, the figure represents the actions of the design cycle. The first step was to familiarize ourselves with RPA. The creation of the model was initiated in straight succession. When structuring the first draft, it was quickly discovered that timing is extremely important in RPA projects. Due to this longitudinal nature of the model's temporal dimension, the present value method was applied to cost appraisal (see, e.g. Götze et al., 2008) by means of discounting cash flows both ex ante deployment and ex post deployment. The model was restructured several times in an iterative manner based on the received feedback. Giving an exact number of cycles is difficult because some feedback was quick to resolve, whereas other rounds took some lengthier back-and-forth discussions. Hence, we use the expression “n” in the figure.
Once the structure was finalized, the empirical grounding of the model was established by means of a business case. Multi-methodological approaches, such as using cases to ground analytic models, are appropriate ways of validating constructs and demonstrating relevance in research (Choi et al., 2016). Field testing of the created artefacts is continuously highlighted by DSR researchers (see, e.g. van Aken, 2004; Peffers et al., 2008), and the notion of contextual, empirical grounding is also included in Hevner's (2007) relevance cycle. The case company, Estate Blend, is a pseudonym for a construction, commercial property and infrastructure developer and a provider of real estate services in Northern Europe to conceal the actual identity of the organization. The business case describes RPA implementation by a leading Nordic service provider of financial and accounting services at a customer site that is Estate Blend.
The research data were provided by the IA unit of the service provider with approval from Estate Blend. This is primary project data that comprises, e.g. the number and type of tasks to be automated, the costs associated with each task prior to RPA deployment, the costs of the RPA investment project, licensing information and certain service pricing guidelines. The researchers were familiarized with the business context during a few practical sessions. To challenge the baseline values of the business case, multiple sensitivity analyses were conducted. In general, sensitivity testing is a sound practice in all modelling to verify that the constructs and their interplay are operating as intended (Murray-Smith, 2015).
3. RPA investments: appraisal and deployment considerations
The appraisal and evaluation of capital investments in information assets and therefore the feasibility analysis of such projects have been long-standing domains of discussion within information systems (IS) and information technology (IT) research. For instance, Joshi and Pant (2008) refer to IT evaluation and project feasibility analysis as a “major theme” beginning in the late 1980s. Similarly, Frisk et al. (2015) state that the evaluation of IS investments has been “a top IT management issue” for a very long time, which has resulted in a continually expanding range of ideas, proposals and assessment methods produced by academia.
However, Frisk et al. (2015) continue by adding that the rate of contributions to IS/IT investments has been declining, which becomes evident with a literature search and retrieval. The focus has shifted from investment appraisal and evaluation towards business value and how novel technologies, such as big data analytics (see, e.g. Mikalef et al., 2017; Grover et al., 2018), can provide that value to organizations. Despite this decline in contributions to the IS/IT academic knowledge base, the need for appraising and evaluating investment projects is not disappearing. For instance, Gartner (2022) forecasts worldwide IT spending to continue as strong in 2023, totaling 4.6tn USD, as enterprises push forward with efficiency-driven digital business initiatives, such as automation projects, in response to economic turmoil.
IS/IT investment research has traditionally been comprised of two streams (Joshi and Pant, 2008); ex post analysis focused on the study of investments and their effects on organizational performance, and ex ante analysis which has centred on techniques to assess investment projects' economic feasibility. Lefley (2013) has pointed out that many scholars apply the terms “appraisal” and “evaluation” interchangeably even though the former should be about the initial process of project justification (i.e. ex ante analysis), while the latter relates to the post-implementation review of project realization (i.e. ex post analysis). In this respect, the term “appraisal” is adopted in the following sections as we approach RPA investments from a project justification perspective.
Apart from the above-mentioned ex ante analysis versus ex post analysis divide, two schools of thought have emerged in the IS/IT investment literature: an economic approach using “hard methods” and a socio-technical approach using “soft methods” (Berghout and Tan, 2013). Hard methods are techniques that perceive investments as something that consist of quantifiable costs and benefits, both of which can be assigned monetary values and measured with various financial metrics. By following the taxonomy of Irani and Love (2002), these methods are economic discounting appraisal and economic ratio appraisal techniques, such as discounted cashflow, net present value, internal rate of return, payback period and cost-benefit analysis.
As eloquently phrased by Frisk et al. (2015), soft methods do not necessarily eschew numbers completely but are often based on much fuzzier types of data, including subjectivity in the assessment process. Irani and Love (2002) categorize these within analytic portfolio appraisal, strategic appraisal, integrated appraisal and other appraisal techniques. Soft methods have emerged from the critique of hard methods, which are argued to have difficulties in not only understanding the human and organizational mechanics of investment decision-making, but also coping with, e.g. intangible, non-financial benefits and indirect costs (Anandarajan and Wen, 1999; Irani, 2002; Joshi and Pant, 2008; Berghout and Tan, 2013; Frisk et al., 2015).
In their study of IS/IT investments, Irani (2002) found that indirect costs are often more significant than more easily quantifiable direct costs, indirect costs also being retrospective in identification and thus in danger of spiralling out of control. Compared to RPA, conventional IS/IT investments are significantly more capital-intensive and lengthier by nature, which accentuates the risk of cost overruns due to indirect costs not being fully recognized (Love et al., 2006). According to Bygstad (2017), RPA is indeed often regarded as the perfect example of lightweight IT, being relatively easy to implement as it does not require heavy investments in IT infrastructure, and robot development can even bypass the organizational IT function.
The notion of IS/IT projects (i.e. the traditional, heavyweight system acquisitions) being fundamentally different from other capital investments in terms of the suitable methods has been voiced in the literature (Peacock and Tanniru, 2005; Lefley, 2013; Hynek et al., 2014). Based on empirical data, Hynek et al. (2014) conclude that IS/IT investments are often perceived to be different, but evidence indicates that, in practice, there is no significant difference in the financial and risk assessment models adopted in appraisal between IS/IT investments and other types of capital investments. They add that these models should be adequate for various IS/IT investment appraisals given that they are supported by proper strategic assessment.
Overall, the critique towards using conventional appraisal methods, such as present value and discounted cashflow, is warranted in terms of traditional IS/IT investments due to the inherent complexity of these projects. Then again, RPA is lightweight IT that requires minimal capital investments in infrastructure, which denotes that, e.g. indirect costs are much less of an issue in comparison to conventional IS/IT investments. Therefore, the economic approach and “hard methods” should be more than adequate for appraising RPA investments, considering that such techniques are still implemented even for conventional IS/IT investments regardless of their shortcomings. According to, e.g. Lefley (2013), practitioners believe that investment appraisal models often have unrealistic assumptions, are somewhat difficult to apply and cannot be explained to top management. This should also be kept in mind when new appraisal tools are developed in the IS/IT research domain. In DSR, the consideration of the practical relevance of research through field testing (Hevner, 2007; Peffers et al., 2008) is a central question. Design artefacts should not only be empirically grounded, but also easy to understand and use in practice.
The foundation of RPA investment projects is the careful consideration of how the RPA deployment is supported with business process modelling, where consistent notations play an important role (Zhang and Liu, 2019). When modelling a business process, certain steps are required to find suitable candidates for robotization (Viehhauser and Doerr, 2021). Firstly, process mining supports the visualization of processes and defines how each one of them contributes to organizational value streams (Leno et al., 2021). According to Grisold et al. (2021), a key issue in process mining is process selection based on the unique properties processes exhibit, e.g. how much data is being handled and produced by each process, which affects automation feasibility.
Secondly, pipeline periodization considers which business processes are prioritized, what type of robots are employed (i.e. attended robots launched manually and/or unattended robots programmed to run at predetermined intervals on dedicated server machines) and who or which party will be responsible for developing the automation solution (Bourgouin et al., 2018). Complementary technologies, e.g. OCR, optical character recognition (Ribeiro et al., 2021), as well as NLP, natural language processing (Rizun et al., 2021), can be adopted on per-process basis to enable RPA to handle increasingly complex tasks. Once all processes with significant automation potential have been systematically mapped, they should be reviewed by experts and key users to obtain the final process versions for solution development. RPA should always be executed on a stable set of information systems without too much process variation, and the data employed should require minimal manual intervention (Gejke, 2018).
4. Deployment framework to support phasing, staging and tasking
During the DSR process, it was quickly discovered that a framework for RPA deployment is required. Having a deployment framework prior to modelling provides a frame of reference for allocating different types of investment costs and understanding how they emerge during RPA project realization. Despite the growing interest towards RPA, project deployment, especially project phasing, staging and tasking have received very little attention in the literature. The sole attempt thus far to provide guidelines on how to introduce RPA in an organization is the consolidated framework by Herm et al. (2020). Other valuable resources to consider in the implementation of RPA are the success criteria of Santos et al. (2020), the action principles of Lacity et al. (2021) and the coding guidelines of Rutschi and Dibbern (2020).
The consolidated framework of Herm et al. (2020) is extracted through a structured literature review and expert interviews. According to Herm et al. (2020), the term “consolidated” refers to the way they established the framework by means of first coding the empirical data, then connecting the literature to the practical perspectives and finally evaluating and iterating the framework through feedback. As illustrated in Figure 2, the consolidated framework comprises three phases (i.e. initialization, implementation and scaling) and a few stages, such as identification, screening, process selection and proof of concept. By comparison, the deployment framework that we established to support the investment modelling within the step-by-step method consists of two phases (i.e. ex ante and ex post deployment) and five consecutive stages (i.e. pre-feasibility planning, feasibility planning, development and hyper-care, operation and maintenance and substitution), as also shown in the figure. Unlike the consolidated framework of Herm et al. (2020), our deployment framework also recognizes several independent tasks, such as automation potential analysis, candidate process mapping and automation process engineering, which incur costs during the realization of each project stage.
In many ways, the consolidated framework of Herm et al. (2020) and our own deployment framework are similar, but they emphasize slightly different aspects of RPA deployment. The consolidated framework adopts a broader, organizational perspective that highlights a need for a centre of excellence for continuous support and solution scaling after the RPA rollout. The deployment framework is more project-centric in its outline of a “from cradle to the grave” type staging (i.e. from pre-feasibility to substitution) and finer project itemization that aims to identify the relevant cost-incurring project tasks. The itemization is also a necessity for investment appraisal modelling where the temporal zero position is determined as the point of deployment, and thus ex ante (before) and ex post (after) are adopted in the naming of the project phases.
A common element for both frameworks is the potential involvement of external help in RPA projects, be that a consultant or a service provider. The possibility to involve external consultants during initialization and especially implementation is recognized in the consolidated framework, and we do share a similar stand on the matter. Service provider tendering is thus a task for pre-feasibility planning, and after they are selected, this partner will be involved throughout the subsequent stages. Due to not having required automation expertise, it is very likely that many, if not most organizations, opt for external procurement (Kedziora et al., 2021), thus creating new business opportunities for service organizations in the field of business process outsourcing (Hallikainen et al., 2018). It is important though that the transaction costs, including, e.g. coordination and adaptation costs, of the customer-supplier relationship are accounted for when companies decide on the appropriate RPA sourcing model (Ge et al., 2021).
The strength of RPA is its flexibility to various sourcing models including, e.g. direct, in-house sourcing, hybrid sourcing and outsourcing where a partner supports the adopting company in various tasks including, e.g. coding, maintaining and hosting of software robots (Asatiani and Penttinen, 2016). The sourcing model affects whether the solution deployment is organized in a centralized or decentralized fashion (Noppen et al., 2020). In a decentralized implementation, the business units in question develop RPA independently, which gives them more breathing room also for adopting more extensive partnering arrangements.
5. Case company's journey towards automation
The case company, Estate Blend, is a construction, commercial property and infrastructure developer, and an established provider of real estate services in Northern Europe. While founded already in the early 1900s, these days Estate Blend is listed on the Nasdaq Nordic Stock Exchange, with hundreds of millions in annual revenue and thousands of employees. Active in several countries on three different continents, the company is continuously seeking innovative solutions and technologies to be able to serve its customer base with top-quality construction, property, infrastructure and real estate products and services at competitive prices.
Estate Blend has been undergoing a digital transformation for nearly 2 decades now. Most of the company's analogue documents have been converted to digital format and information systems migrated to the cloud. In the area of business process automation, its employees started to apply local scripts, macros and low-code automation 10 years ago. Then about 5 years ago, software robots entered the premises of the company. The spark to develop process automation further at Estate Blend originated from market pressure and a strategic imperative to improve the quality and efficiency of their back-office operations. One of the most important drivers of the automation transition was a reduction in capital and operational expenditure. Saving money was not the only objective though as they wanted to transform the employees' jobs by releasing them from the most time-consuming, repetitive and boring tasks.
Estate Blend's plan was to improve the quality of information processing throughout their business functions. Lower error rates become visible in repetitive tasks where humans tend to start making more and more mistakes the longer the process is continuously executed. Software robots' lead times should be shorter in comparison and the results much easier to predict as the process execution always takes the same time and brings deterministic results based on input quality. Automations are easier to manage, e.g. there are no attrition rates, stronger endurance to crises (transaction picks or drops), no emotionally justified underperformance, no inter-personal competition or conflicts between employees and simplified governance. As an additional bonus, automated reporting is more transparent and easier to follow and audit when necessary.
To bring RPA into fruition, Estate Blend appointed a manager who was responsible for establishing a project team that would comprise the required process owners and experts. Soon after the initiation, a first meeting was held with a service provider to lay the groundwork for a proof of concept. The service provider would help the company screen for an RPA software vendor and conduct necessary feasibility studies for initially selected processes. Seven candidate processes were chosen for automation soon after the meeting: three candidate processes from the area of Finance and Accounting (F&A), two from Customer Service (CS), one from HR and Payroll (HR&P) and one from Procurement (P), as shown in Table 1.
The workloads for each process were estimated with the service provider's experts in follow-up meetings. The essential information for assessing not only the workloads but also the costs were provided, e.g. how many hours each process takes to perform, how many persons do it, at which times of the day or week, what are the hourly rates and performance bonuses of these persons, their annual absences and leaves on average and how all the above are currently calculated in the company. The candidate processes were categorized into three types of business processes, each requiring either light, moderate or advanced workload in full-time equivalents (FTE). By automating these seven processes, Estate Blend releases a workload from manual processing, which is commensurate to five full-time employees (5 FTE) and is estimated to be around 315,000 EUR annually. Building a business case and demonstrating a proof of concept for RPA adoption with these seven processes was the starting point of Estate Blend's RPA journey.
6. Investment appraisal and sensitivity analyses
6.1 In preparation of modelling
When applying conventional investment appraisal techniques, such as discounted cashflow and present value methods, to an RPA investment project, we must, in preparation of modelling, establish how the concept of time (t) is going to be dealt with as it directly affects the compounding of the interest rate. The project phasing and staging in the case of Estate Blend's investment follows the deployment framework that was created to support the modelling process (see Figure 2). The temporal dimension, or simply time (t), is divided into several shorter periods of time within ex ante deployment and ex post deployment phases, as illustrated in Figure 3.
The three letters of the alphabet, “a”, “b” and “c”, mark the beginning of each period and corresponding project stage ex ante deployment. Pre-feasibility planning (a), which includes service provider tendering, is initiated at Estate Blend six months prior to the planned RPA deployment. Feasibility planning (b) commences a month later, which is the point from which onwards the service provider is heavily involved in the RPA solution development. Solutioning and vendor selection is one of the most important tasks in feasibility planning as it delineates the RPA software vendor and thus related RPA platform components that are available at later stages, including the cost-intensive, four-month-long development and hyper-care (c).
The deployment occurs when t = 0, denoting that the pre-feasibility planning (a), feasibility planning (b) and development and hyper-care (c) stages receive only negative numerical values in the calculations. On the ex post side of deployment, we have the operation and maintenance stage beginning at t = 1 and then ceasing when time reaches “x”. Estate Blend's operation and maintenance stage is hypothesized to span eight-quarters before extensive revisions to establish automation routines are required. Although it is regarded as a conservative estimate, there is no way of knowing how such an assumption eventually holds out in practice. Hence carrying out “what if” type of sensitivity analyses when conducting investment appraisals is very important.
6.2 Compound the interest rate
As the first step in modelling, we must decide how the interest rate is compounded. As RPA investments are extremely rapid by nature, the interest rate should be ideally compounded more frequently than annually, which is the standard practice in discounted cashflow and present value approaches in most investment appraisals. Our model is designed to be flexible, allowing the use of, e.g. monthly, quarterly or annual compounding as well as varying compounding frequencies ex ante deployment versus ex post deployment. The mathematical basis for determining periodic interest rates is presented in (1) and (2) in Appendix. The term “periodic” stands for the periodic nature of discounting, e.g. monthly compounding frequency introduces 12 compounding periods to the calculation from which the incurred costs are discounted to present-day values.
While the most appropriate approach to compounding is situational, it is important to choose the stated interest rate carefully to obtain credible results. The stated interest rate, which is the rate from which the periodic interest rate is calculated, can be founded on the weighted average cost of capital (WACC) in which the weight of each capital type (i.e. equity and long-term debt) is delineated by its average cost and total amount. It should be noted though that interest rates are ideally dynamic and target-oriented, thus reflecting the investment type and future expectations rather than the past alone in a mechanical fashion, which may often be the case with WACC.
The compounding frequency was selected to be asymmetrical for Estate Blend. The investment characteristics of RPA projects, such as a fast delivery cycle, are better captured with monthly and quarterly compounding compared to the annual alternative. As presented in Figure 4, a 10% stated interest rate is adopted at Estate Blend. After factoring in the compounding, the stated rate is translated to a monthly rate of 0.873% ex ante deployment and a quarterly rate of 2.595% ex post deployment. An argument for over-precision could be made against such fine compounding, which would likely be true in the scale of one investment alone. When Estate Blend later expands RPA to other business areas and a higher number of processes are automated, the multiplicative effects of the more accurate compounding start to show their significance.
6.3 Discount the investment costs
Once the periodic interest rates have been calculated ex ante and ex post, the second step in modelling is to first determine and then to discount the investment costs through the itemization of project tasks incurring costs within the five stages. Starting with the stages preceding deployment, i.e. pre-feasibility planning, feasibility planning and development and hyper-care, (3) gives the cumulative present value ex ante deployment, CPVea (see Appendix).
The way (3) can be implemented in a spreadsheet application, such as Excel, to discount the investment costs and to calculate CPVea is illustrated in Figure 5. The costs incurred periodically by each project task at the corresponding project stage ex ante deployment are systematically discounted to present values (t = 0) using the periodic interest rate (i.e. here the monthly rate, 0.873%). The yellow fields are model inputs that are used to calculate the outputs in the white fields consisting of total costs and present values. CPVea is the aggregated total of the three cumulative present values determined for each stage.
The numbers shown in Figure 5 represent Estate Blend's RPA investment costs, but they have been rounded to the closest hundred for clarity. The currency is thousand euro (TEUR). The costs incurred at the pre-feasibility planning stage comprise mainly working hours and indirect employee costs of Estate Blend's IT experts conducting preparatory tasks, such as assessing the automation potential of various business processes and selecting candidates for further analysis.
A great deal of the costs incurred later at the feasibility planning and development and hyper-care stages are invoicing from Estate Blend's service provider, who becomes involved when detailed automation requirement analysis and process mapping are initiated. During development and hyper-care, the service provider is responsible for engineering the automation solution and for rigorous testing and tweaking through continuous feedback by Estate Blend's end users.
Furthermore, for the stages succeeding deployment, i.e. operation and maintenance and substitution, (4) to (6) can be applied to determine the cumulative present value ex post deployment, CPVep (see Appendix 1). The implementation through (4) to (6) to discount the investment costs and to calculate CPVep is presented in Figure 6. The costs incurred periodically by licenses and project tasks ex post deployment are systematically discounted to present values (t = 0) using the periodic interest rate (i.e. here the quarterly rate, 2.595%). In the case of Estate Blend, there is no planned obsolescence for RPA routines being adopted, but such a scenario could be modelled in the fields relating to “robotic process substitution” if necessary.
As illustrated in Figure 6 and shown in (5) and (6), operation and maintenance can be further itemized to multiple subcomponents. The various licensing fees that Estate Blend is invoiced by the service provider are considered separately on a quarterly basis (each fee also includes the list price of the RPA vendor). The annual fees for each type of license (see Figure 4) are first divided by the number of employed compounding periods and then multiplied by periodic license quantities to arrive at the total cost of each license type for each quarter. The maintenance costs are crudely divided into hosting, bug-fixing and software updates, but this itemization can be more nuanced in practice depending on the service-level agreement.
As can be seen from the number of license types, Estate Blend's RPA platform for the seven processes to be automated consists of an orchestrator, two attended robots and two unattended robots. A studio license is acquired so that RPA routines can be later reviewed by Estate Blend's IT department, and minor revisions performed without external assistance from the service provider. The orchestrator component is responsible for controlling the unattended robots that perform the advanced and moderate processes based on a predetermined schedule. The four attended robots are activated by human operators ad hoc to perform the light processes.
6.4 Establish measures for comparison
The third and final step in modelling is to establish a measure for comparing the RPA investment costs against a known data point, which is the current cost of the processes to be automated. Cumulative present value, CPV, for the timespan of the investment is calculated as per (7) by summing up both CPVea and CPVep (see Appendix). As CPV represents only the aggregate investment costs, a measure that is directly comparable against the status quo is required for sound decision-making. CPV is, therefore, converted with (8) to an equivalent periodic cost, EPC. The periodic cost difference, PCD, can then be finally determined from (9). All these measures in Estate Blend's appraisal are illustrated in Figure 7.
The CPV for Estate Blend's RPA investment is around 270K, translating to an EPC of 38K when distributed to the hypothesized eight-quarter life of the investment. When EPC is compared against CPC, the cost of manual processing at Estate Blend, the difference between the two measures is 41K per quarter in favour of adopting RPA. It should be noted that despite its significantly shorter length, CPVea accounts for around 126K, which is nearly 47% of the total costs. Such front-end-weighted nature of RPA investments denotes that adhering to a pre-determined schedule should not be underestimated. Delays are likely to shift the EPC closer to the CPC.
High ROI numbers in the realm of 600–850% that we often see in the literature were not realized for Estate Blend, whose ROI is around 120%. It should be acknowledged that the investment is very much a proof of concept that is appraised using a conservatively short two-year life and only 5 FTEs worth of processes that are automated in the first phase of RPA transition. Most of the costs are allocated to the development of RPA infrastructure and routines scale with a higher number of processes. If the development costs (CPVea) are omitted from the appraisal, Estate Blend's ROI already increases to 290% with this small portfolio of business processes.
6.5 In conclusion of modelling
The reality of establishing an investment appraisal based on a business case is that it always captures the situation as it unfolded at a singular point in time. The results of the investment appraisal are heavily depended on the baseline values employed in modelling, including, e.g. the interest rate and the lengths of the ex ante deployment and ex post deployment phases. Sensitivity analysis was, therefore, conducted on Estate Blend's RPA investment to reveal how alternating circumstances and requirements would influence the economic reality of the investment. The influence of timing the ex ante deployment (a → 0) and ex post deployment phases (0 → x) was studied especially in the conducted sensitivity analysis. The project's timing effects on EPC at three moderate interest rates are illustrated in Figure 8.
The postponement of solution deployment combined with a short ex post deployment period seems to impact Estate Blend's investment costs significantly. If the deployment falls behind the schedule by four months (a = −10), and RPA is operational only four-quarters (x = 4) after deployment instead of the initial eight, the EPC reaches values between 76 and 92K. The illustrated reference line for CPC is surpassed at 20–30% interest rates, denoting a negative PCD in these extreme scenarios. The mean for EPC across the investigated scenarios is around 45K, which is safely below Estate Blend's CPC. As a short operation and maintenance stage increases cost levels regardless of the scenario, the processes to be automated with RPA should remain operationally stable in the short-to mid-term to ensure that the investment stays economically sound.
7. Discussion
It is time to summarize and discuss what was achieved in the paper. Firstly, we are going to reintroduce our step-by-step method to RPA investment appraisal (see section 7.1), a DSR artefact that was built around both mapping of processes (i.e. the deployment framework) and modelling the associated costs (i.e. the investment model). Secondly, we give our experience-based recommendations on how the developed method of investment appraisal can be employed in practice (see section 7.2). Thirdly, some of the lessons learned are discussed by reflecting on Estate Blend's case and our contributions to the literature underlined (see Section 7.3). Lastly, the evaluation of the DSR artefact is touched upon (see Section 7.4).
7.1 The step-by-step method to RPA investment appraisal
The objective of this study was to obtain a toolbox, “step-by-step method”, which could be employed universally to assess the economic feasibility of RPA investments. Based on the DSR process documented in the paper, we suggest the following step-by-step method to RPA investment appraisal. The steps can be modified to each organization's unique situation.
- (1)
Preparatory Step: Establish a consistent system of classification (i.e. apply a process framework to map the phases, stages, tasks and other project elements). Use and adapt a deployment/implementation framework to your phasing, staging and tasking needs.
- (2)
Modelling Steps: Track and trace all project costs and when they occur. Attach these costs to the phases, stages, tasks and other cost-incurring project elements. Model, assess and iterate when necessary. Use the following steps:
Step 1: Compound the Interest Rate
Step 2: Discount the Investment Costs
Step 3: Establish Measures for Comparison
- (3)
Concluding Step: Compare the investment against the status quo. Remember to also conduct sensitivity analyses, especially on variables that are the least conservative estimates.
- (4)
Optional Step: Recruit a service partner, such as a consultant or service provider, to assist in RPA solution development including e.g. the coding, maintaining and hosting of software robots.
7.2 Our recommendations to practice
When deploying RPA in an organization, the recommended preparatory step, based on our experience with developing the step-by-step method, is to plan the implementation and deployment with a framework to establish a system of classification using consistent notations. The systematic mapping of phases, stages, tasks and other potential cost-incurring elements was conducted by applying our deployment framework discussed in the section “Deployment Framework to Support Phasing, Staging, and Tasking”. Other frameworks can also be utilized as the process of mapping organizational workflows and processes is extremely context specific.
At the early stages of adoption, we recommend evaluating whether RPA is something that the IT department can handle on its own or whether an external service partner should perhaps be recruited to smooth the company's path to automation. Having a service partner, such as a consultant or a service provider, with a prior track record has many benefits. They can help select the best process candidates, decide on the most suitable RPA vendor and environment and get everything operational from coding through testing to tweaking the robots. Many service partners also provide after-sales services and continued support in the form of, e.g. bug-fixing services.
The modelling itself is divided into three consecutive steps discussed in the section “Investment Appraisal and Sensitivity Analyses” and elaborated on in Appendix 1: “How to Model RPA Investments?” Our recommendation is to conduct these three steps together with appropriate modelling techniques, such as discounted cash flow and present value methods. The equations shown in Appendix must be naturally adjusted according to the framework that is selected to illustrate the RPA project phases, stages, tasks and other cost-incurring project elements. We advise against not compounding the interest rate as the effects can accumulate with longer investment lifetimes and a greater number of projects and processes to be automated.
We highly recommend discerning between the ex ante deployment phase and the ex post deployment phase. Based on our experience, this makes investment comparisons easier by defining what the total initial investment to be recouped is and how that cost compares against the costs of alternatives, such as the manual processing of tasks. If a service partner is involved, it is recommended to share information for the sake of transparency. Benefit-sharing agreements may act as a motivational tool especially in longer, non-transactional business relationships.
After accounting for all the elements in the investment appraisal, it is important to conduct sensitivity analyses as a “sanity check” to avoid pitfalls later during or after RPA deployment. Virtually any variable and its value can be altered in the model, and the effects are recorded accordingly. In addition to avoiding pitfalls, sensitivity analyses are quick, yet powerful tools to challenge poor assumptions. Under the sub-section “In Conclusion of Modelling”, we exemplify how what-if type sensitivity analyses can be conducted by fitting multiple scenarios.
7.3 Lessons learned and contributions underlined
In the section “Introduction”, we mentioned that the economic benefits of RPA are based on anecdotal notions in the literature (see, e.g. Lacity and Willcocks, 2016; van der Aalst et al., 2018). As demonstrated with Estate Blend, the step-by-step method to RPA investment appraisal is a toolbox that can be employed to reveal these potential benefits in a systematic fashion. From a purely economic, “to invest or not” standpoint, the decision to invest in RPA seems trivial for Estate Blend as periodic investment costs (i.e. EPC) were notably lower than current processing costs (i.e. CPC). ROI was around 120% which is significantly lower than what has been previously reported at around 600–850% (Lacity and Willcocks, 2016). There are some caveats, e.g. Estate Blend having a small portfolio of processes and a relatively short investment life.
Estate Blend's case illustrates how resistant RPA investments are to changing circumstances. Reasonable project delays and normal postponements in the solution development are not detrimental from an investment economics point of view. RPA as a technology does not fit all types of organizational workflows, processes, or environments, but when it does, it is difficult to ignore its benefits. The EPC that measures Estate Blend's periodic investment costs relating to RPA deployment remained lower than current cost levels in most sensitivity scenarios tested.
The step-by-step method addresses prior shortcomings in the RPA literature, including methodological support for implementation, support for benefit realization and comprehensive metrics for benefits (Syed et al., 2020). The deployment framework (i.e. the process phasing, staging, tasking and recognition of other cost-incurring elements) contributes as new methodological support for RPA implementation. Both the deployment framework and the investment model provide new support for RPA benefit realization by together establishing a toolbox for systematically conducting RPA investment appraisal. The comprehensive metrics for benefits are outlined in this study as a handful of key performance measures (e.g. the EPC).
7.4 Evaluation of the DSR artefact
Finally, the evaluation of the DSR artefact should be briefly touched upon, which is a central question in DSR. Hevner (2007), for instance, states that while the design cycle (i.e. artefact development) is the heart of any DSR process, both the initial motivation for developing artefacts and the final evaluation in the application domain (i.e. field testing) are carried out in the relevance cycle. Peffers et al. (2008) have developed a design science research methodology (DSRM), which conveys a nominal process on how to conduct, and consequently evaluate, DSR. In DSRM, the process of evaluating a DSR artefact is divided into two components: demonstration and evaluation.
Demonstration denotes that the developed artefact is applied, e.g. by means of a case study, to solve at least one instance of the stated field problem. Evaluation, on the other hand, stands for an activity that involves comparing the objectives of the solution to actual observed results from using the developed artefact in the demonstration. According to Peffers et al. (2008), evaluation could include any appropriate empirical evidence or logical proof, such as quantitative performance measures or other quantifiable measures of system performance (e.g. time or availability).
In this study, the demonstration of the artefact was achieved with Estate Blend's case where the step-by-step method was successfully applied to the company's RPA investment appraisal. Evaluation of the artefact is embedded in the artefact's design as the modelling steps comprise several performance measures (e.g. PCD and EPC) and the concluding step directs the user to conduct sensitivity analysis to account for uncertainty in the baseline values. The experience with Estate Blend was that the step-by-step method and its measures provide actionable outputs.
Overall, the field testing of our step-by-step method seems to provide an adequate preliminary grounding for the artefact. We say “preliminary” as full verification of an artefact in the intended application domain can be a time-consuming and complex process in DSR (see, e.g. Johannesson and Perjons, 2014). Estate Blend is something that was available to us, but further field testing and possible reiterations of the artefact should be considered in other cases and domains. As pointed out by Peffers et al. (2008), the evaluation of DSR artefacts can be also founded on time-based measures. The current iteration of the model accounts only for direct, measurable benefits, but having, e.g. better process or service availability can be incredibly valuable to companies.
8. Conclusions
To continue from the ideas presented in the closing sentences of the Discussion, there are still multiple avenues for further research. The current iteration of the investment model concentrates exclusively on direct, measurable benefits, but RPA as a technology is often claimed to have numerous indirect effects, such as elevating work satisfaction by releasing employees to more meaningful tasks (Willcocks et al., 2017) and therefore impacting the customer experience (Hallikainen et al., 2018). The personnel's ability to perform alternative, value-adding tasks after RPA deployment could be included as a “profit” type element in the model. Other benefits to be accounted for are potential error reduction (Kokina and Blanchette, 2019) and also time savings (Aguirre and Rodriguez, 2017) in time-sensitive applications.
To maximize the benefits in the long term, RPA should be scaled once the proof of concept is demonstrated with a few successful deployments (Herm et al., 2020). Although the importance of automation scaling is recognized in the step-by-step method, the scaling effects would deserve even further attention and itemization to establish a holistic view of both the total cost of RPA ownership and the total value of RPA ownership in organizations. As it stands, the model is currently adaptable to an infinite number of RPA deployments, but the user must manually adjust for the reduced costs in subsequent projects and processes by, e.g. following an exponential reduction of costs (the learning curve effect) based on the number of repetitions (Lolli et al., 2016).
The economic strength of RPA, evidenced also in Estate Blend's investment case, can promote benefit-sharing arrangements and incentive schemes (win-win, open-book accounting) that have traditionally been challenging in inter-organizational relationships (Ylä-Kujala et al., 2018). Adding these kinds of elements to service contracts (e.g. gain sharing based on attained benefits) is not only an interesting managerial proposition, but also a potential research avenue, which has been touched on, e.g. by Lazzarotto et al. (2014) in the business process management context. Their analysis of contractual designs concluded that companies seem to use performance-based compensation but sharing performance gains with service providers to promote continuous improvement is non-existent. Lastly, but importantly, a larger investment portfolio should be addressed longitudinally as it is possible that, e.g. benefits start to decline on certain RPA investment types once an “X” amount of time has passed after deployment due to, e.g. technical limitations, process revisions, or other changes in the business environment over time.
Figures
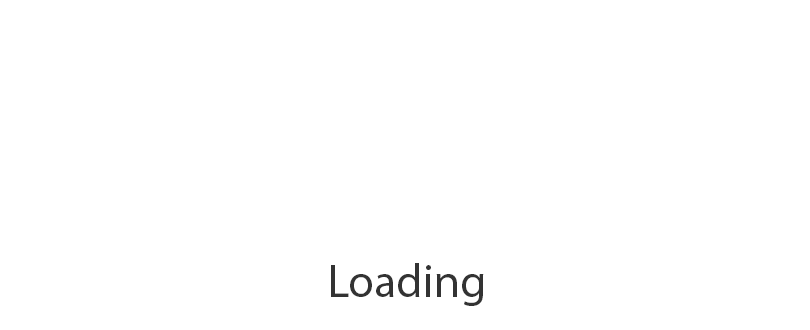
Figure 2
The consolidated framework (Herm et al., 2020) vs the deployment framework
Candidate process descriptions, types and FTEs by business function
Business function | Process description | Type | FTE |
---|---|---|---|
F&A | Matching mismatched invoices with work orders | Light | 0.25 |
F&A | Controlling of invoices currently in fluctuation | Moderate | 1.00 |
F&A | Reconciling travel expense and payroll accounts | Light | 0.25 |
CS | Distributing tasks among employees of customer care centre | Advanced | 2.00 |
CS | Chasing end users for answers to pending customer care tickets | Light | 0.25 |
HR&P | Calculating monthly salary proposals based on company records | Light | 0.25 |
P | Collecting data from five systems (order and asset mgmt., billing, invoicing and reporting) and distributing data to relevant units | Moderate | 1.00 |
Compound the Interest Rate
When more than one compounding period per annum is employed, the interest rate used in discounting various cashflows is altered. The effective annual interest rate (ie) that basically delineates the influence of compounding is determined from equation (1).
As the discounting of costs is performed in the present value method on a periodical basis (given that n > 1), the effective annual interest rate (ie) must be divided by the amount of “n” so that the periodic interest rate (r) used in the modelling can be determined as per equation (2).
The periodic interest rate (r) is thus dependent on the stated interest rate (is) and the number of compounding periods per annum (n).
Discount the Investment Costs
To begin with the stages preceding deployment, the cumulative present value ex ante deployment (CPVea) is given by equation (3).
Furthermore, for the stages succeeding deployment, the cumulative present value ex post deployment (CPVep) is given by equation (4).
OMt, which is typically the longest stage in RPA projects, can be further itemized to subcomponents according to equation (5).
Establish Measures for Comparison
The cumulative present value (CPV) for the entire timespan of the RPA investment is calculated by summing up CPVea and CPVep as shown in equation (7).
As CPV only reveals the overall costs of the investment, a measure that is comparable against the status quo (when t = 0) is required so that an informed investment decision can be made. Thus, CPV needs to be converted to an equivalent periodic cost (EPC), which, in terms of conventional investment calculus, is the annuity of CPV given by equation (8).
References
Aguirre, S. and Rodriguez, A. (2017), “Automation of a business process using robotic process automation (RPA): a case study”, in Figueroa-García, J., López-Santana, E., Villa-Ramírez, J. and Ferro-Escobar, R. (Eds), Communications in Computer and Information Science, Springer, Heidelberg, Vol. 742, pp. 65-71, WEA 2017.
Anandarajan, A. and Wen, J. (1999), “Evaluation of information technology investment”, Management Decision, Vol. 37 No. 4, pp. 329-337.
Asatiani, A. and Penttinen, E. (2016), “Turning robotic process automation into commercial success: case OpusCapita”, Journal of Information Technology Teaching Cases, Vol. 6, pp. 67-74.
Badakhshan, P., Conboy, K., Grisold, T. and vom Brocke, J. (2020), “Agile business process management: a systematic literature review and an integrated framework”, Business Process Management Journal, Vol. 26 No. 6, pp. 1505-1523.
Berghout, E. and Tan, C.-W. (2013), “Understanding the impact of business cases on IT investment decisions: an analysis of municipal e-government projects”, Information and Management, Vol. 50 No. 7, pp. 489-506.
Berghout, E., Nijland, M. and Powell, P. (2011), “Management of lifecycle costs and benefits: lessons from information systems practice”, Computers in Industry, Vol. 62 No. 7, pp. 755-764.
Bourgouin, A., Leshob, A. and Renard, L. (2018), “Towards a process analysis approach to adopt robotic process automation”, ICEBE 2018-15th International Conference on e-Business Engineering, pp. 46-53.
Bygstad, B. (2017), “Generative innovation: a comparison of lightweight and heavyweight IT”, Journal of Information Technology, Vol. 32 No. 2, pp. 180-193.
Carden, L., Maldonado, T., Brace, C. and Myers, M. (2019), “Robotics process automation at TECHSERV: an implementation case study”, Journal of Information Technology Teaching Cases, Vol. 9 No. 2, pp. 72-79.
Choi, T.-M., Cheng, T.C.E. and Zhao, X. (2016), “Multi-methodological research in operations management”, Production and Operations Management, Vol. 25 No. 3, pp. 379-389.
Cooper, L.A., Holderness, D.K., Sorensen, T.L. and Wood, D.A. (2019), “Robotic process automation in public accounting”, Accounting Horizons, Vol. 33 No. 4, pp. 15-35.
Dunlap, R. and Lacity, M. (2017), “Resolving tussles in service automation deployments: service automation at Blue Cross Blue Shield North Carolina (BCBSNC)”, Journal of Information Technology Teaching Cases, Vol. 7, pp. 29-34.
Frisk, J.E., Bannister, F. and Lindgren, R. (2015), “Evaluation of information system investments: a value dials approach to closing the theory-practice gap”, Journal of Information Technology, Vol. 30 No. 3, pp. 276-292.
Gartner (2022), “Gartner forecasts worldwide IT spending to grow 5.1% in 2023”, available at: https://www.gartner.com/en/newsroom/press-releases/2022-10-19-gartner-forecasts-worldwide-it-spending-to-grow-5-percent-in-2023 (accessed 8 November 2022).
Ge, L., Wang, X. and Yang, Z. (2021), “The strategic choice of contract types in business process outsourcing”, Business Process Management Journal, Vol. 27 No. 5, pp. 1569-1589.
Gejke, C. (2018), “A new season in the risk landscape: connecting the advancement in technology with changes in customer behaviour to enhance the way risk is measured and managed”, Journal of Risk Management in Financial Institutions, Vol. 11 No. 2, pp. 148-155.
Ghauri, P. and Grønhaug, K. (2002), Research Methods in Business Studies: A Practical Guide, 2nd ed., Pearson Education, Essex, Great Britain, p. 212.
Götze, U., Northcott, D. and Schuster, P. (2008), Investment Appraisal: Methods and Models, Springer-Verlag, Berlin, Germany, p. 391.
Grisold, T., Mendling, J., Otto, M. and vom Brocke, J. (2021), “Adoption, use and management of process mining in practice”, Business Process Management Journal, Vol. 27 No. 2, pp. 369-387.
Grover, V., Chiang, R.H.L., Liang, T.-P. and Zhang, D. (2018), “Creating strategic business value from big data analytics: a research framework”, Journal of Management Information Systems, Vol. 35 No. 2, pp. 388-423.
Hallikainen, P., Bekkhus, R. and Pan, S.L. (2018), “How OpusCapita used internal RPA capabilities to offer services to clients”, MIS Quarterly Executive, Vol. 17 No. 1, pp. 41-52.
Herm, L.-V., Janiesch, C., Helm, A., Imgrund, F., Fuchs, K., Hofmann, A. and Winkelmann, A. (2020), “A consolidated framework for implementing robotic process automation projects”, in Fahland, D., Ghildini, C., Becker, J. and Dumas, M. (Eds), LNCS 12168 Business Process Management, Springer, Cham, pp. 471-488.
Hevner, A.R. (2007), “A three cycle view of design science research”, Scandinavian Journal of Information Systems, Vol. 19 No. 2, pp. 87-92.
Hevner, A.R., March, S.T., Park, J. and Ram, S. (2004), “Design science in information systems research”, MIS Quarterly, Vol. 28 No. 1, pp. 75-105.
Hofmann, P., Samp, C. and Urbach, N. (2020), “Robotic process automation”, Electronic Markets, Vol. 30 No. 1, pp. 99-106.
Huang, F. and Vasarhelyi, M.A. (2019), “Applying robotic process automation (RPA) in auditing: a framework”, International Journal of Accounting Information Systems, Vol. 35, pp. 1-11.
Hynek, J., Janecek, V., Lefley, F., Puzova, K. and Nemecek, J. (2014), “An exploratory study investigating the perception that ICT capital projects are different – evidence from the Czech Republic”, Management Research Review, Vol. 37 No. 10, pp. 912-927.
Irani, Z. (2002), “Information systems evaluation: navigating through the problem domain”, Information and Management, Vol. 40 No. 1, pp. 11-24.
Irani, Z. and Love, P.E.D. (2002), “Developing a frame of reference for ex-ante IT/IS investment evaluation”, European Journal of Information Systems, Vol. 11 No. 1, pp. 74-82.
Johannesson, P. and Perjons, E. (2014), An Introduction to Design Science, Springer International Publishing, Switzerland, p. 197.
Joshi, K. and Pant, S. (2008), “Development of a framework to assess and guide IT investments: an analysis based on a discretionary-mandatory classification”, International Journal of Information Management, Vol. 28 No. 3, pp. 181-193.
Kedziora, D. and Penttinen, E. (2020), “Governance models for robotic process automation: the case of Nordea Bank”, Journal of Information Technology Teaching Cases, Vol. 11 No. 1, pp. 1-10.
Kedziora, D., Leivonen, A., Piotrowicz, W. and Öörni, A. (2021), “Robotic process automation (RPA) implementation drivers: evidence of selected Nordic companies”, Issues in Information Systems, Vol. 22 No. 2, pp. 21-40.
Knudsen, D.-R. (2020), “Elusive boundaries, power relations, and knowledge production: a systematic review of the literature on digitalization in accounting”, International Journal of Accounting Information Systems, Vol. 36 March 2020, 100441.
Kokina, J. and Blanchette, S. (2019), “Early evidence of digital labor in accounting: innovation with robotic process automation”, International Journal of Accounting Information Systems, Vol. 35, pp. 1-13.
Lacity, M. and Willcocks, L. (2016), “Robotic process automation at Telefónica O2”, MIS Quarterly Executive, Vol. 15 No. 1, pp. 21-35.
Lacity, M. and Willcocks, L. (2021), “Becoming strategic with intelligent automation”, MIS Quarterly Executive, Vol. 20 No. 2, pp. 1-14.
Lacity, M., Willcocks, L. and Gozman, D. (2021), “Influencing information systems practice: the action principles approach applied to robotic process and cognitive automation”, Journal of Information Technology, Vol. 36 No. 3, pp. 216-240.
Lazzarotto, B.O., Borchardt, M., Pereira, G. and Almeida, C. (2014), “Analysis of management practices in performance-based outsourcing contracts”, Business Process Management Journal, Vol. 20 No. 2, pp. 178-194.
Lefley, F. (2013), “The appraisal of ICT and non-ICT capital projects – a study of the current practices of large UK organisations”, International Journal of Managing Projects in Business, Vol. 6 No. 3, pp. 505-533.
Leno, V., Polyvyanyy, A., Dumas, M., La Rosa, M. and Maria Maggi, F. (2021), “Robotic process mining: vision and challenges”, Business and Information Systems Engineering, Vol. 63 No. 3, pp. 301-314.
Lolli, F., Messori, M., Gamberini, R., Rimini, B. and Balugani, E. (2016), “Modelling production cost with the effects of learning and forgetting”, IFAC – Papers Online, Vol. 49 No. 12, pp. 503-508.
Love, P.E.D., Irani, Z. and Ghoneim, A. (2006), “An exploratory study of indirect ICT costs using the structured case method”, International Journal of Information Management, Vol. 26 No. 2, pp. 167-177.
Mendling, J., Decker, G., Hull, R., Reijers, H.A. and Weber, I. (2018), “How do machine learning, robotic process automation, and blockchains affect the human factor in business process management?”, Communications of the Association for Information Systems, Vol. 43, pp. 297-320.
Mikalef, P., Pappas, I.O., Krogstie, J. and Giannakos, M. (2017), “Big data analytics capabilities: a systematic literature review and research agenda”, Information Systems and E-Business Management, Vol. 16, pp. 547-578.
Murray-Smith, D.J. (2015), Testing and Validation of Computer Simulation Models: Principles, Methods and Applications, Springer International Publishing, p. 252.
Ng, K.K.H., Chen, C.-H., Lee, C.K.M., Jiao, J. and Yang, Z.-X. (2021), “A systematic literature review on intelligent automation: aligning concepts from theory, practice, and future perspectives”, Advanced Engineering Informatics, Vol. 47 January 2021, 101246.
Noppen, P., Beerepoot, I., van der Weerd, I., Jonker, M. and Reijers, H.A. (2020), “How to keep RPA maintainable?”, in Fahland, D., Ghildini, C., Becker, J. and Dumas, M. (Eds), LNCS 12168 Business Process Management, Springer, Cham, pp. 453-470.
Peacock, E. and Tanniru, M. (2005), “Activity-based justification of IT investments”, Information and Management, Vol. 42 No. 3, pp. 415-424.
Peffers, K., Tuunanen, T., Rothenberger, M.A. and Chatterjee, S. (2008), “A design science research methodology for information systems research”, Journal of Management Information Systems, Vol. 24 No. 3, pp. 45-77.
Ribeiro, J., Lima, R. and Paiva, S. (2021), “Document classification in robotic process automation using artificial intelligence – a preliminary literature review”, Lecture Notes in Networks and Systems, Vol. 204, pp. 211-221.
Rizun, N., Revina, A. and Meister, V.G. (2021), “Assessing business process complexity based on textual data: evidence from ITIL IT ticket processing”, Business Process Management Journal, Vol. 27 No. 7, pp. 1966-1998.
Rutschi, C. and Dibbern, J. (2020), “Towards a framework of implementing software robots: transforming human-executed routines into machines”, The Data Base for Advances in Information Systems, Vol. 51 No. 1, pp. 104-128.
Santos, F., Pereira, R. and Braga Vasconcelos, J. (2020), “Toward robotic process automation implementation: an end-to-end perspective”, Business Process Management Journal, Vol. 26 No. 2, pp. 405-420.
Schmitt, A. and Hörner, S. (2021), “Systematic literature review – improving business processes by implementing agile”, Business Process Management Journal, Vol. 27 No. 3, pp. 868-882.
Syed, R., Suriadi, S., Adams, M., Bandara, W., Leemans, S.J.J., Ouyang, C., ter Hofstede, A.H.M., van de Weerd, I., Thandar Wynn, M. and Reijers, H.A. (2020), “Robotic process automation: contemporary themes and challenges”, Computers in Industry, Vol. 115 No. 1, pp. 1-15.
Team IM (2021), “Measuring the ROI of robotic process automation (“RPA”) implementation”, available at: https://www.teamim.com/blog/measuring-roi-for-rpa/ (accessed 25 August 2022).
van Aken, J.E. (2004), “Management research based on the paradigm of the design sciences: the quest for field-tested and grounded technological rules”, Journal of Management Studies, Vol. 41 No. 2, pp. 219-246.
van Aken, J.E. (2005), “Management research as a design science: articulating the research products of mode 2 knowledge production in management”, British Journal of Management, Vol. 16 No. 1, pp. 19-36.
van der Aalst, W.M.P., Bichler, M. and Heinzl, A. (2018), “Robotic process automation”, Business and Information Systems Engineering, Vol. 60, pp. 269-272.
Viehhauser, J. (2020), “Is robotic process automation becoming intelligent? Early evidence of influences of artificial intelligence on robotic process automation”, in Asatiani, A., García, J. M., Helander, N., Jiménez-Ramírez, A., Koschmider, A., Mendling, J., Meroni, G. and Reijers, H.A. (Eds), BPM Blockchain and RPA Forum 2020 Proceedings, LNBIP, Vol. 393, pp. 101-115, Springer AG.
Viehhauser, J. and Doerr, M. (2021), “Digging for gold in RPA projects – a quantifiable method to identify and prioritize suitable RPA process candidates”, in La Rosa, M., Sadiq, S. and Teniente, E. (Eds), Advanced Information Systems Engineering, CAiSE 2021, Springer, Cham, pp. 313-327.
Ward, J., Daniel, E. and Peppard, J. (2008), “Building better business cases for IT investments”, MIS Quarterly Executive, Vol. 7 No. 1, pp. 1-15.
Willcocks, L., Lacity, M. and Craig, A. (2015), “The IT function and robotic process automation”, The Outsourcing Unit Working Research Paper Series, Paper 15/05, October 2015, available at: http://eprints.lse.ac.uk/64519/1/OUWRPS_15_05_published.pdf (accessed 25 August 2022).
Willcocks, L., Lacity, M. and Craig, A. (2017), “Robotic process automation: strategic transformation lever for global business services?”, Journal of Information Technology Teaching Cases, Vol. 7, pp. 17-28.
Ylä-Kujala, A., Marttonen-Arola, S. and Kärri, T. (2018), “Finnish ‘state of mind’ on inter-organizational integration: a cost accounting and cost management perspective”, The IMP Journal, Vol. 12 No. 1, pp. 171-191.
Zhang, N. and Liu, B. (2019), “Alignment of business in robotic process automation”, International Journal of Crowd Science, Vol. 3 No. 1, pp. 26-35.