Abstract
Purpose
The study examines the impact of digital platforms and supply chain capability on operational performance and tests the mediation effect of supply chain capability. Further, the purpose is to examine the moderating effect of digital culture and sharpen our knowledge of how organizational culture as a contextual factor affects the firm's digitalization.
Design/methodology/approach
The data were harvested from 194 Finnish manufacturing companies, and structural equation modeling was used to test the hypotheses.
Findings
The findings show that digital platforms positively and significantly affect supply chain capability. Moreover, supply chain capability mediates the relation between digital platforms and operational performance. Further, this study confirms that digital culture is a contextual factor that explains the differences in the effects of digital platforms on firm performance.
Originality/value
This study is one of the first attempts to examine the effect of digital culture in the context of digital platforms, supply chain capabilities, and operational performance.
Keywords
Citation
Hautala-Kankaanpää, T. (2022), "The impact of digitalization on firm performance: examining the role of digital culture and the effect of supply chain capability", Business Process Management Journal, Vol. 28 No. 8, pp. 90-109. https://doi.org/10.1108/BPMJ-03-2022-0122
Publisher
:Emerald Publishing Limited
Copyright © 2022, Tuire Hautala-Kankaanpää
License
Published by Emerald Publishing Limited. This article is published under the Creative Commons Attribution (CC BY 4.0) licence. Anyone may reproduce, distribute, translate and create derivative works of this article (for both commercial and non-commercial purposes), subject to full attribution to the original publication and authors. The full terms of this licence may be seen at http://creativecommons.org/licences/by/4.0/legalcode
1. Introduction
The fast pace of digitalization has altered the competitive logic of the industries, the value chains (Aaldering and Song, 2021; Ghobakhloo and Iranmanesh, 2021), and firms' internal and inter-organization processes (Holmström et al., 2019). Firms adopt digital technologies to manage their operations, supply chain activities, and real-time visibility (ArditoPetruzzelli et al., 2019); hence, businesses are increasingly connected (Seyedghorban et al., 2020). This phenomenon encompasses industries globally and is sometimes also referred to as Industry 4.0 (e.g. Bazan and Estevez, 2022; Bienhaus and Haddud, 2018; Wamba and Queiroz, 2020). It emphasizes increased vertical and horizontal integration of manufacturing processes (Dalenogare et al., 2018; Wagire et al., 2020). Digital technologies such as digital platforms (DPs) offer information integration (Li et al., 2020; Sedera et al., 2016), support visibility and decision making (Yang et al., 2013), and provide interoperability between different software and technologies (ArditoPetruzzelli et al., 2019). Thus, they are seen as an enabler of more digitalized supply chains (Gartner, 2018). Digital platforms, as a form of integration software, offer an opportunity for seamless information flow, communication, and connectivity in firms and in supply chains (Chi et al., 2018; Sedera et al., 2016).
In general, researchers have examined the relationship between digital technologies and firm performance, and it is observed that the research does not offer clear evidence about the benefits of digitalization on performance (Kohtamäki et al., 2020). Some studies report a weak or nonexistent role (AlMulhim, 2021; Lee et al., 2022; Liang et al., 2010); some research claims that digital technology directly supports performance (Eller et al., 2020; Li et al., 2020); and some claims that it undermines performance (Jeffers et al., 2008). Further, researchers have noticed that contextual and organizational factors complement Industry 4.0 technologies (Culot et al., 2020). Hence, how digital technology is used in a firm's operations is more likely to explain the different performance benefits gained from the use of digital technologies than the technology itself. This advancement has highlighted the role of firm capabilities, such as supply chain capabilities that use digital technologies to support firm performance (Wu et al., 2006). RBV-based digital-capability framework (Bharadwaj, 2000; Cho et al., 2017; Mithas et al., 2011; Rai et al., 2006) argues that a firm's operations must combine a range of digital resources such as DP and firm capabilities if the firm is to derive performance benefits.
In addition, the contingency perspective of the resource-based view is adopted in this study and argued that complementary organizational aspects might explain the differential result of using digital technology (Cao et al., 2011; Wiengarten et al., 2013). This study also investigates the moderating role of digital culture, referring to the openness and acceptance of digitalization-related technology (Blatz et al., 2018). Digital culture, like organizational culture, is identified as one of the causes preventing the change needed to become more digitalized (Fitzgerald et al., 2013; Hartl and Hess, 2017).
The empirical research on digital culture is limited and has focused on the data-driven culture (Yu et al., 2021), the use of IT (Leidner and Kayworth, 2006), the intention to adopt internet-enabled supply chain management systems (Liu et al., 2010), big data analytics (Dubey et al., 2019), and digital organizational culture (Martínez-Caro et al., 2020). Nevertheless, organizational digital cultural attributes are an underdeveloped aspect of digitalization research (Nadkarni and Prügl, 2020). Further, the current digitalization research does not isolate possible moderating factors (Kohtamäki et al., 2020), nor their role in the relation of DP and operational performance. Therefore, the key objective of this study is to examine the mediating effect of supply chain capability (SCC) between DP and operational performance; and the contextual role of digital culture. The present study used an empirical analysis of 194 firms to test the research hypotheses.
The remainder of this paper is organized as follows. The first section summarizes the relevant research on the digital-capability framework, DPs, SCC, and digital culture and introduces the study's hypotheses. The second presents the research methodology, data collection, measurement validation, and results. The final section incorporates a discussion of the analysis and contributions of the study and outlines its limitations.
2. Theoretical background and hypothesis development
2.1 RBV-based digital-capability framework and organizational context
RBV-based digital-capability research focuses on how digital technology creates value for firms (Barua et al., 2004; Dong et al., 2009; Zhu and Kraemer, 2002). It is notable that digital resources, such as digital software or digital technology, do not independently explain the performance effect of a firm (Bharadwaj, 2000; Mata et al., 1995; Mishra et al., 2007; Rai et al., 2006; Tippins and Sohi, 2003; Wu et al., 2006). That is especially the case if the resource is a common technology that competitors might mimic and adopt (Bi et al., 2013; Tippins and Sohi, 2003). When they are applied independently, digital resources have little direct influence on firm performance, which might explain why the potential of the value of digital resources seems to have faded (Dong et al., 2009; Wiengarten et al., 2013). Nevertheless, it is clear that digital resources impact other resources, capabilities, and processes that can enhance performance (Wade and Hulland, 2004). To continue, SCC describes the firm's ability to identify, leverage, and adapt its internal and external information and resources to manage activities related to the supply chain (Amit and Schoemaker, 1993; Collis, 1994; Wu et al., 2006; Yu et al., 2018). The combination of SCC and resources builds the main premise of performance advantages (Morash and Lynch, 2002).
The contingency perspective of the resource-based view suggests that complementary organizational aspects might explain the differential result of using digital technology (Cao et al., 2011; Wiengarten et al., 2013). Organizational culture is identified as one of the causes preventing the change needed to become more digitalized (Fitzgerald et al., 2013; Hartl and Hess, 2017). In general, an organization's culture influences how it conducts its business (Barney, 1986) and reflects the beliefs and values shared in the organization (Miller, 1993) and the ways we see the world (Davison and Martinsons, 2002). It is, therefore, possible to argue that a digital culture that reflects the beliefs and values connected to the use of digital technology influences how an organization conducts its business facilitated by digital technology. The common theme applied is that digital culture is more of an organizational-level critical competence that affects the use of DPs in a firm's operations.
2.2 Digital platforms
A digital platform is a form of software used to control production and logistics, manage the data, and support the integration of applications and processes between companies (Sedera et al., 2016). The software considered in inter-organizational integration is the Internet of things (IoT) platforms, integration platforms, and supply chain management platforms. These enabling technologies offer an opportunity to connect different forms of software and applications seamlessly and assure interoperability (ArditoPetruzzelli et al., 2019).
Although there are several possible positive outcomes of using DP, they are more likely to be accessed if firms have the capabilities to benefit from the technology and to use the information, visibility, and connectivity offered. Therefore, DPs per se may have limited positive performance effects as they do not offer opportunities to differentiate from competitors if competitors adopt the same general software (Bhatt et al., 2010; Tippins and Sohi, 2003). In addition, DPs can guide user firms to manage their processes in similar ways (Markus and Loebbecke, 2013). If competitors use similar software, none of them should be outperforming any others on account of the DP itself. Instead, differentiation is sought through other means, such as the combination of resources, capabilities, and organizational factors.
The meta-analysis by Liang et al. (2010) indicated that the direct connection between organizational digital resources and firm performance is weak or nonexistent. In addition, recent empirical research has found no relationship between digitalization and firm performance (AlMulhim, 2021; Lee et al., 2022). Nevertheless, empirical research also shows that digital technology can both support (Eller et al., 2020; Li et al., 2020; Rosenzweig, 2009) and undermine performance (Jeffers et al., 2008). However, it is expected that a DP will have a positive effect on a firm's operational performance because of its integrative nature that supports the visibility and the ability to share real-time information and data (e.g. ArditoPetruzzelli et al., 2019; Sedera et al., 2016) Accordingly, the first hypothesis is:
Digital platforms have a positive effect on operational performance
2.3 Supply chain capability
Supply chain capability forms the basis of a company's supply chain operations and is considered an explanatory factor in the success of a firm (Morash et al., 1996; Morash, 2001). Further, this capability reflects a firm's ability to conduct business activities internally and within the supply chains (Bi et al., 2013); and thus, it fosters business performance that is connected to the availability of products, convenience, and low level of distribution costs (Morash et al., 1996).
Supply chain capability has been defined in several ways in previous research, including as an aggregate construct that combines four different dimensions (Wu et al., 2006); as a construct where those dimensions are modeled independently (Yu et al., 2018); and as a combination of three independent dimensions (Bi et al., 2013). Moreover, independent dimension of coordination (Liao et al., 2017), agility and flexibility (Yusuf et al., 2004), integration (Rai et al., 2006; Leuschner et al., 2013; Rajaguru and Matanda, 2019; Yu et al., 2020) and collaboration (Fawcett et al., 2011) has been used as SCC. In this research, SCC should be read as including three different dimensions: information exchange, activity integration, and responsiveness. Those three are well-known and important activities in the supply chain process. Information exchange deals with effective and efficient knowledge sharing in supply chains (Wu et al., 2006). It is a vital part of various business activities, such as forecasting, inventory, and customer management (Wei et al., 2020), demand management, sales, production, and delivery processes (Rai et al., 2006). Activity Integration describes internal channel integration based on two premises, technology integration and activity integration (Wu et al., 2006). Further, responsiveness reflects the ability to respond to environmental transformation (Wu et al., 2006), compete effectively, and react to changes in demand and supply (Yu et al., 2018). Coordination deals with firm-internal and inter-organizational coordination that reflects the firm's ability to effectively coordinate activities relating to transactions, materials, and orders (Wu et al., 2006). These dimensions reflect a firm's ability to manage and interact with supply chains and, therefore, be seen as essential drivers for firm operations.
At a general level, SCCs are expected to positively influence firm performance (Ataseven and Nair, 2017; Chang et al., 2016; Leuschner et al., 2013; Rajaguru and Matanda, 2019; Wong et al., 2015; Yu et al., 2018). They offer information-related advantages derived from multiple sources (Wu et al., 2006) that support on-time delivery and help identify uncertainties (Wong et al., 2015); those advantages can also drive inventory reduction and cost-saving (Hau et al., 2000; Wong et al., 2011). Further, SCC increases responsiveness, which can improve operational performance (Bhatt et al., 2010). Digitally supported SCC is an inter-firm capability and, therefore, valuable to firms per se (Bi et al., 2013). This finding leads to the next hypothesis:
SCC has a positive effect on operational performance
2.4 The relation between DPs and SCC
Firms invest in digitalizing their supply chains and operations to achieve various effects, such as, enhanced delivery speed, flexibility, connectivity, on-time inventory, intelligence, transparency, and proactivity (Büyüközkan and Göçer, 2018). Digitally advanced supply chains differ from traditional vertical integration forms in being integrated via information flows rather than ownership (Dong et al., 2009), and digital technology is recognized as a key driver of this kind of integration (Yusuf et al., 2004). In addition, DPs facilitate sharing and analyzing information and deriving benefit from interactions in supply chains; therefore, they have a significant role in information exchange, especially in situations where supply chains are dispersed when they facilitate easy access to information and data. Digital platforms accelerate information exchange and can signal a need to respond to changes in the market.
Prior research indicates that using digital technologies in supply chains improves operational efficiency (Calatayud et al., 2019; Singh and El-Kassar, 2019; Yang et al., 2021; Zhu and Kraemer, 2002). It also positively influences differential SCCs, such as supply chain process integration (Rai et al., 2006; Rajaguru and Matanda, 2019; Yu et al., 2020) and collaboration capabilities (Fawcett et al., 2011). DPs support a firm's SCC and enable firms to share timely, appropriate, and confidential data within supply chains. Therefore, DPs can positively influence SCC by facilitating constant information sharing, supporting integration, and offering access to real-time information and a flow of data that enables firms to operate effectively in a timely manner and respond to changes.
Digital platforms have a positive effect on SCC
2.5 The mediating role of SCC
Previous research offers empirical support for SCCs' transformative role between digital resources and performance (Wu et al., 2006; Yu et al., 2020). Supply chain capability can be used as a mediator representing the value-creation mechanism between DPs and performance. Supply chain capability helps firms conduct business activities, in which DPs are used in organizational processes, and the use of the DPs is adapted to firm needs. In this case, the value-creation mechanism of SCC is the capacity of a firm to successfully use a DP to fit its own activities.
Previous research indicates that different digital resources and capabilities (Cámara et al., 2015; Chen et al., 2014; Hallikas et al., 2021; Mikalef et al., 2020; Yu et al., 2018) require mediation support from a firm's capabilities to deliver performance gains. Hence the next hypothesis is presented:
SCC positively mediates the relationship between DPs and operational performance.
2.6 Moderating effect of digital culture
Firms differ in how successfully they use digital technologies (Martínez-Caro et al., 2020). It is argued that digital resources alone affect firm performance in only a minor way and that significant improvement requires the presence of organizational factors such as culture (Wiengarten et al., 2013). Further, Melville et al. (2004) argue that organizational culture interacts with IT when value is generated. In digitalized context, digital culture relates to a firm's openness to and acceptance of digital technologies (Blatz et al., 2018), and openness to new thinking is a basic requirement relating to digital technologies (Witschel et al., 2019). If the organization is open and willing to use digital technologies (i.e. it has a supportive digital culture), it can more easily exploit DPs and apply them to its processes. Whereas if digital technologies are resisted by their user, it is more likely that the use of DPs remains at a lower level and will be rather ineffective. Consequently, the benefits derived are likely to be limited. In this sense, digital culture is seen as a factor that may affect the effectiveness of the DPs used in a firm's operations.
Previous research indicates that a data-driven culture moderates the effect of big data analytics on supply chain finance (Yu et al., 2021). Furthermore, digital organizational culture indirectly affects operational performance (Martínez-Caro et al., 2020); culture affects the use and adoption of digital technologies (Leidner and Kayworth, 2006; Liu et al., 2010). This study argues that the effect of DPs is related to the digital culture in those firms. Therefore, the following hypotheses are formulated:
Digital culture moderates the relationship between DPs and SCC
Digital culture moderates the relationship between the DPs and operational performance
2.7 Dependent variable: operational performance
This article includes operational performance as an aggregate construct that describes the firm's delivery performance, cost performance, quality performance, and production flexibility (Ward and Duray, 2000). Delivery performance is a combination of reliability and delivery speed; cost performance indicates a firm's ability to reduce production and inventory costs, whereas quality performance incorporates the ability to meet customer needs; production flexibility is the ability to change product features and product mix (Ward and Duray, 2000).
2.8 Control variables
This study uses firm size, industry, and age as its control variables. Firm size is included in the research model because larger firms may have more resources available to assign (Wu et al., 2006; Rueda-Manzanares et al., 2008; Chen et al., 2014), which may reflect on operational performance. In addition, the industry is controlled for as there may be industry-level differences between the firms (Capon et al., 1990; Melville et al., 2004; Jayaram et al., 2010). Firm age is included because younger firms may not have the same experience-related advantages as their older counterparts (Autio et al., 2000; Chen et al., 2014). The research model is presented in Figure 1.
3. Research methodology
3.1 Data collection and sample
The data were collected from firms operating in the manufacturing sector between December 2019 and April 2020. Firms with a general manufacturing category (C) and a turnover of between EUR 1.5 m and EUR 50 m were randomly selected from the Orbis database. When analyzing the data, one firm's turnover was below the threshold (EUR 0.9 m), but the firm's data were retained for inclusion. A mixed-methods approach comprising both an email invitation and direct calling was used to invite the firms to participate in the study. A total of 1,136 Finnish companies were contacted, 414 by telephone. Eventually, 194 acceptable responses were received, a result considered suitable for SEM (Wolf et al., 2013; Sideridis et al., 2014). Responses were received mostly as a result of the telephone calls; only 21 out of the firms responded to an email. The response rate was 17%, and the respondents mainly held managerial positions such as CEO (83%) and CFO (13%). The majority of the companies operate in the metal industry (32%), others in electric or electronic machinery (22.7%), food manufacturing (9.8%), leather, stone, clay, and glass production (3.6%), wood, furniture, and paper manufacturing (9.3%), and other manufacturing sectors (8.8%). Table 1. presents the sample demographics.
3.2 Measures
The 3-item scale labeled DP was a novel one; the items on the DP scale relate to the use of the general commercial platforms such as IoT platforms, integrative DP, and supply chain management platforms. The IoT supports visibility, data integration, and a constant flow of information (Yang et al., 2013). The typical background for these systems is that they support internal and inter-organizational integration, connectivity, and information and data availability (Sedera et al., 2016). Internet of Things platforms, integrative DPs, and supply chain management platforms are seen as future trends in supply chain management (Gartner, 2018; Kousiouris et al., 2019) and are therefore at the heart of this study. Prior to the survey, the three academics developed the construct used to measure DPs. The survey instrument was reviewed by a representative of a manufacturing firm and by an IT industry specialist. Explorative factor analysis tested the constructs and helped assess the validity and reliability of the construct, which led to one factor emerging.
The test then continued with analyzing the measures. A confirmatory factor analysis was used to test the validity of the scales. The reliability measures average variance extracted (AVE), composite reliability (CR), and Cronbach's alpha (α) were tested, and the results are reported in Appendix. All the scales were measured with a 7-point Likert-type scale anchored with completely disagree (1) and completely agree (7) and were estimated through the respondent's perceptual evaluation. All these scales were considered as reflective constructs.
The analysis started with the DPs scale, which loaded with good values (0.64–0.81), and reliability (CR = 0.78; AVE = 0.54; α = 0.77) was at an acceptable level.
The digital culture scale (a 5-item scale) was adopted from a previous study by Blatz et al. (2018). All items loaded above the 0.5 level (0.53–0.88) and showed good reliability (CR = 0.87; AVE = 0.57; α = 0.86).
Supply chain capability (a 15-item scale) was measured on a scale adapted from that of Wu et al. (2006) that included four different dimensions—information exchange (CR = 0.92, AVE = 0.75, α = 0.93), activity integration (CR = 0.89, AVE = 0.74, α = 0.89), and responsiveness (CR = 0.85, AVE = 0.60, α = 0.85) and coordination (CR = 0.66, AVE = 0.42 α = 0.69). However, one item related to a firm's ability to perform the business at less cost than its competitors was removed from the coordination dimension because of low loading (0.29) (Hair et al., 2019). The reliability of the coordination dimension was then acceptable, as the AVE value exceeded the critical level of 0.4, and the CR was more than 0.6 (Fornell and Larcker, 1981; Malhotra, 2010). All dimensions of SCC loaded at acceptable levels (CR = 0.83, AVE = 0.56, α = 0.90) indicating the SCC as aggregate construct could safely be used.
The 13-item operational performance scale was adapted from prior research (Ward and Duray, 2000; Wong et al., 2011) and measured delivery performance (CR = 0.86, AVE = 0.61, α = 0.85), cost performance (CR = 0.84, AVE = 0.56, α = 0.84), quality performance (CR = 0.87, AVE = 0.77, α = 0.87), and production flexibility (CR = 0.87, AVE = 0.78, α = 0.87). One item from the operational flexibility scale that measured a firm's ability to change production volumes was removed owing to a low loading (0.46). The test continued with an analysis of the operational performance construct, which established that the reliability was acceptable (CR = 0.72, AVE = 0.41, α = 0.86) (Fornell and Larcker, 1981; Malhotra, 2010). Table 2 presents the correlations, means, and standard deviations.
All control variables were measured with a single item. The largest industry sector was that of metals and metal products and was therefore controlled. The dummy variable was coded (Aiken and West, 1991) as 0 (other industries) and 1 (metal industry). Size is a continuous variable measured by a firm's turnover. Age is also a continuous variable.
In addition, the validity of the constructs was evaluated. Measuring the discriminant validity established that the square root of AVE-values was higher than the correlation of other constructs (Fornell and Larcker, 1981). The relevant values are shown in italicface on the diagonal in Table 2. The maximum shared squared variance (MSV) was assessed; all MSV values range from 0.193 to 0.330, indicating that the AVE value is higher on every construct measured. These results offer evidence of discriminant validity. MSV values can be found in Appendix. In addition, the heterotrait-monotrait ratio of correlations (HTMT) technique determined the discriminant validity between constructs. The results varied between 0.113 and 0.556 and stayed below the threshold value of 0.900 (e.g. Henseler et al., 2015).
A confirmatory factor analysis was conducted on the measurement model using Amos 26 software. The result indicated that the data have an acceptable fit to the overall model (x2/df; 1.78; CFI = 0.90; NFI = 0.80; IFI = 0.90; RMSEA = 0.06; TLI = 0.90). Further, skewness and kurtosis were used to estimate the multivariate normality. The skewness values ranged from −0.954 to 1.718, clearly between the −2 and +2 values that indicate acceptability (Brown, 2006; Collier, 2020). The kurtosis value ranged from −2.078 to 5.680, thus below 7.0 (Byrne, 2016) and within the higher criterion range of −10 to + 10 (Collier, 2020).
3.3 Common method bias
The data were collected from a single respondent from each firm, which prompted the testing of common method variance. Harman's single-factor test was conducted to examine the unrotated factors (Podsakoff and Organ, 1986; Podsakoff et al., 2003). The simple one-factor test with SPSS software was conducted, and the results indicate that the first factor explained 26.6% of the variance, well below the cut-off threshold value of 50%. The test then proceeded with Amos 26 software. The single-factor model produced an extremely poor fit to the data (x2/df = 5.76; CFI = 0.38; NFI = 0.34, RMSEA = 0.16; TLI = 0.34), these results indicate that common method bias is not a concern.
4. Analysis and results
The analysis continued with structural equation modeling. The results of the main research model indicated an acceptable model fit to the data (x2/df = 1.69; CFI = 0.94; NFI = 0.87; IFI = 0.94; RMSEA = 0.06; TLI = 0.89). The next step was a multicollinearity test. The variance inflation factor (VIF) was found to vary between 1.101 and 1.348, below the threshold value of >5 (Hair et al., 2011). The results relieved concerns over potential multicollinearity.
4.1 Direct relations
The test started with the analysis of direct relations. The results show that DPs (β = −0.148, p < 0.01) directly and negatively affect performance, which means H1 is not supported. Further, SCC (β = 0.397, p < 0.001) has a positive and significant direct effect on the firm's operative performance, thus supporting H2. DPs (β = 0.267, p < 0.001) directly and positively affect SCC, which supports H3. The control variables, size (β = −0.105, p = 0.054), and age (β = −0.046, p = 0.400), did not affect operational performance, whereas industry (β = −0.115, p < 0.05) had a negative and significant effect on operational performance. In addition, the effect of digital culture on operational performance was controlled for, the results showing a significant direct effect of digital culture on operational performance (β = 0.266, p < 0.001).
4.2 Mediation analysis
Zhao et al.'s (2010) steps procedure was followed to analyze the possibility of mediation. The indirect effect between a DP through SCC was tested first, thus removing the direct link from a DP to operational performance. A bootstrapping approach with 5,000 iterations and 95% confidence intervals was adopted to test the mediation effects (Hayes, 2018). The results show that the indirect effect of DPs (β = 0.098, p ≤ 0.001) on operational performance was positive and significant, which indicates a possible indirect relationship, where the mediation effect of these independent variables on performance is conveyed through SCC (James and Brett, 1984; James et al., 2006). The test process continued with an analysis of the direct relationship with the mediator removed from the model. Because the direct path from DPs (β = −0.047, p = 0.441) on operational performance was not significant, the study findings confirm full mediation (Zhao et al., 2010) between the DP and operational performance, which supports H4.
4.3 Moderation analysis
The constructs for digital culture and DP were multiplied to provide an interaction term to measure the effect of digital culture as a moderator (Bollen, 1989). The interaction term was treated as an independent variable in the model. The results show that digital culture does not moderate the relation between the DP and SCC (β = 0.088 p = 0.162), thus rejecting H5a. Instead, the digital culture significantly and positively moderates the relationship between a DP and operational performance (β = 0.223, p < 0.001), which supports H5b. The results are presented in Figure 2.
Figure 3 shows the effect of the interaction between DPs and operational performance. The results suggest that firms with high levels of digital culture are also those in which the use of a DP is more positively associated with operational performance. In contrast, firms with a low level of digital culture tend to report a negative association between a DP and operational performance.
This study presents several findings. The results show that using DPs positively impacts SCC. Further, SCC had a positive relationship with operational performance, which shows the importance of this capability to a firm's operations. A direct and negative relationship was established between DPs and operational performance. However, the mediation analysis shows that SCC positively mediates the effect of a DP on operational performance. A moderation analysis showed that digital culture dampened the negative relationship between a DP and operational performance. Surprisingly, digital culture did not moderate the relationship between DPs and SCC. As a result, firms are more likely to benefit from a DP if they have adequate capabilities and an organizational culture that supports digitalization.
5. Conclusion and limitations
5.1 Discussion and theoretical implications
As digitalization continues to increase in importance for firms and their supply chains, there is a significant opportunity for organizations to improve the use of DPs and harvest the benefits of technological development. Nevertheless, understanding of the effects of DPs on performance is limited (Cenamor et al., 2019), and more information would be required to explain the different performance outcomes. The purpose of this study was to examine the effect of DPs on SCC and operational performance; an additional purpose was to examine the role of SCC as a transformative mechanism through which the use of DBs creates value. This study shows that together these two concepts support firm performance.
This study confirms that DPs are basic digital resources that do not independently influence firm performance positively. A combination of DPs and SCC can, however, confer performance benefits. Further, SCC exerts a positive and significant mediation effect between DPs and operational performance. These results support the findings of Cámara et al. (2015), Chen et al. (2014), Hallikas et al. (2021), Mikalef et al. (2020) and Yu et al. (2018). More specifically, they offer new information about the performance effects of DPs. The results indicate that firms would benefit from having processes and capabilities designed to extract value from a DP. That suggestion supports the notion that DPs should be considered basic resources that positively influence firm performance when embedded in a firm's processes.
This study also examined the role of a digital culture that is open to and supportive of digital technologies. This study contributes by showing that digital culture moderates the relationship between a DP and operational performance. A firm lacking a supportive digital culture may be at risk in the digital era. The findings of this study extend the research of Wiengarten et al. (2013) and Yu et al. (2021) in showing that digital culture as an organizational factor plays a meaningful role in the context of a firm's digitalization and DPs.
The research of Nadkarni and Prügl (2020) showed that organizational culture is an underdeveloped topic in digitalization research. This study showed how digital culture is related to a firm's digitalization efforts and specifically to the use of DPs. This study also offers possible explanations for a recently witnessed differential performance effect of digitalization (e.g. AlMulhim, 2021; Eller et al., 2020; Lee et al., 2022). This study addresses the need highlighted in the research of Verhoef et al. (2021) to improve the understanding of digital resources and especially their role in the successful digital journey of companies. This study fills the gap by showing the effect of DPs and, more importantly, empirically demonstrates the importance of a supportive digital culture in the context of performance gains. It also shows why a digital culture is valuable to a firm's operations and performance.
To conclude, this study contributes by offering a coherent view of the effect of DPs and SCC on operational performance by including the digital culture as a contextual factor. It offers new information to inform the digitalization conversation by testing the effect of digital culture and showing that digitalization as a phenomenon is intertwined with the firm's capabilities and cultural attributes. Therefore, academics could widen their analysis related to digitalization to cover organizational aspects to increase the understanding of this complicated phenomenon.
5.2 Managerial implications
From the managerial point of view, the most important takeaway is that the cultural aspects of an organization may offer firms a productive path toward performance success when they support the use of DPs. By building a digital culture that supports different digital resources, firms are more likely to benefit from the investments associated with digitalization. While strategizing digitalization, managers could include the cultural aspects of their strategic planning to improve firms' acceptance and support for digital-related solutions. Furthermore, this study shows the importance of SCC to firm performance. That capability was highly relevant to the participating firms' operational performance in every situation measured, thus showing the importance of organizational-level capabilities using DPs to deliver performance outcomes.
5.3 Limitations and future research
There are some limitations to this research. The data informing this study were gathered from manufacturing firms, limiting its generalizability to firms outside the manufacturing field. Further, this study is limited by its Finnish context, which could complicate generalization across different countries. The data were also collected from a single managerial respondent in each firm, which may weaken the reliability of the findings.
This study focuses on digital platforms and their effect on supply chain capabilities and operational performance and the moderating role of digital culture. It seems important for future research to examine the role of digital culture more closely in the context of other digital resources and capabilities. Further, future studies might examine why the level of digital culture is higher in some firms than in others and what explains and supports the emergence of digital culture. Future research could combine case studies and surveys to offer supplemental insights into these issues.
Figures
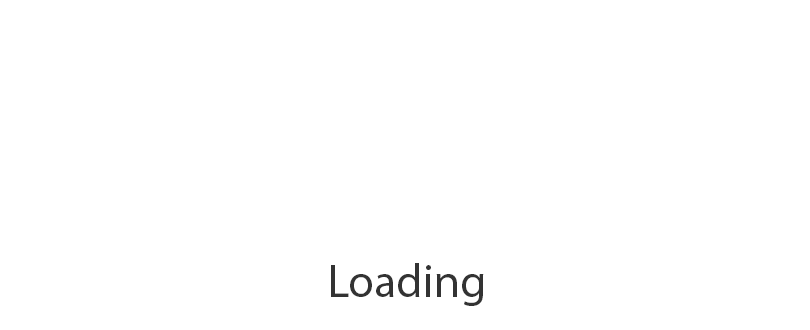
Figure 2
The results of the SEM are presented in Table 3
Correlations, means and standard deviations
Variable | Mean | SD | 1 | 2 | 3 | 4 | 5 | 6 | 7 |
---|---|---|---|---|---|---|---|---|---|
1. Company age | 27.02 | 16.23 | |||||||
2. Company size (Turnover) | 9,217 | 0.45 | 0.09 | ||||||
3. Metals and metal products | 0.32 | 0.47 | −0.15* | −0.14 | |||||
4. Digital platforms | 2.19 | 1.45 | −0.06 | 0.12 | 0.06 | 0.73 | |||
5. Digital culture | 5.18 | 1.06 | 0.02 | 0.16* | −0.14* | 0.09 | 0.76 | ||
6. SSC | 4.14 | 0.85 | −0.02 | 0.16* | 0.01 | 0.30*** | 0.44*** | 0.75 | |
7. Operational performance | 4.66 | 0.89 | −0.03 | 0.01 | −0.12 | −0.03 | 0.47*** | 0.49*** | 0.64 |
Note(s): *p ≤ 0.05; ***p ≤ 0.001
The results of the SEM
Control variables | |
Company age | −0.046 |
Metal industry | −0.115* |
Company size: turnover | −0.105 |
Direct effects | |
Digital platforms → SCC | 0.267*** |
Digital platforms → OP | −0.148** |
SCC → OP | 0.397*** |
Mediation effect | |
Digital platforms → SCC → OP | 0.098*** |
Moderation effects | |
Digital culture * Digital platforms → SCC | 0.088 |
Digital culture * Digital platforms → OP | 0.222*** |
R2 | 0.43** |
x2 | 1.69 |
x2/df | 25.30 |
Df | 15 |
CFI | 0.94 |
TLI | 0.89 |
NFI | 0.87 |
IFI | 0.94 |
RMSEA | 0.06 |
Note(s): *p ≤ 0.05; **p ≤ 0.01; ***p ≤ 0.001
Scale and item | Loadings | CR | AVE | α | MSV |
---|---|---|---|---|---|
Digital Platforms | 0.78 | 0.54 | 0.77 | 0.19 | |
IoT platforms for controlling production, logistics, or products and managing data (e.g. Microsoft Azure IoT Hub, IBM Watson IoT, IoT Ticket) | 0.64 | ||||
Integration platforms for enterprise application integration (e.g. Mulesoft, Jakamo, Liaison) | 0.81 | ||||
Supply chain management platforms for integration of processes between companies and multiplexing interactions (e.g. Pool for Tool, SAP Ariba, Jakamo, or firm SCM portal) | 0.74 | ||||
Digital Culture | 0.87 | 0.57 | 0.86 | 0.33 | |
Adapted from Blatz et al. (2018) | |||||
There is a positive attitude to digital technologies | 0.81 | ||||
Employees are ready to take advantage of new digital operations models | 0.83 | ||||
Employees see opportunities in digitalization | 0.88 | ||||
There is a positive attitude in a firm to remote working with digital technologies | 0.53 | ||||
There is a positive attitude to the training on digitalization | 0.71 | ||||
Supply Chain Capability (SCC) Adapted from Wu et al. (2006) and Yu et al. (2018) | 0.83 | 0.56 | 0.90 | 0.28 | |
Information Exchange (IE) | 0.92 | 0.75 | 0.93 | ||
Our company exchanges more information with its partners than our competitors do with their partners | 0.85 | ||||
Information flows more freely between our company and its partners than between our competitors and their partners | 0.88 | ||||
Our company benefits more from information exchange with its partners than our competitors do from exchanges with their partners | 0.90 | ||||
Our information exchange with our partners is superior to the information exchange of our competitors and their partners | 0.83 | ||||
Activity Integration (AI) | 0.89 | 0.74 | 0.89 | ||
Our company develops strategic plans in collaboration with its partners | 0.74 | ||||
Our company collaborates on forecasting and planning with its partners | 0.93 | ||||
Our company projects and plans future demand collaboratively with its partners | 0.90 | ||||
Responsiveness | 0.85 | 0.60 | 0.85 | ||
Compared to our competitors, our supply chain responds more quickly and effectively to changing customer and supplier needs | 0.78 | ||||
Compared to our competitors, our supply chain develops and markets new products more quickly and effectively | 0.70 | ||||
In most markets, our supply chain competes effectively | 0.88 | ||||
The relationship with our partners has increased our supply chain responsiveness to market changes through collaboration | 0.71 | ||||
Coordination | 0.66 | 0.42 | 0.69 | ||
Our company conducts transaction follow-up activities more efficiently with our partners than do our competitors with their own partners | 0.66 | ||||
Our company spends less time coordinating transactions with our partners than our competitors with their own partners | 0.60 | ||||
Our company has reduced partnering costs more than our competitors | 0.66 | ||||
Our company can perform the business at less cost than our competitors | Deleted | ||||
Operational Performance Adapted from Ward and Duray (2000); Wong et al. (2011) | 0.72 | 0.41 | 0.86 | 0.33 | |
Delivery performance | 0.86 | 0.61 | 0.85 | ||
Our delivery times are shorter than industry average | 0.59 | ||||
Our delivery punctuality is good or better than the industry average | 0.96 | ||||
The reliability of our delivery is good or better than industry average | 0.96 | ||||
We have been able to reduce the time it takes to process the order more than the industry average | 0.54 | ||||
Cost performance | 0.84 | 0.56 | 0.84 | ||
Our production costs are below industry average | 0.81 | ||||
The cost of storing our products is lower than industry average | 0.60 | ||||
Overheads of our products are lower than industry average | 0.78 | ||||
Price competitiveness of our products is better than industry average | 0.80 | ||||
Quality performance | 0.87 | 0.77 | 0.87 | ||
The quality of our products has been steady and quality deviations are less common than the industry average | 0.89 | ||||
Our products are reliable and match our customers' standards better than the industry average | 0.87 | ||||
Production flexibility | 0.87 | 0.78 | 0.87 | ||
Our ability to change production volume is better than industry average | Deleted | ||||
Our ability to customize products is better than industry average | 0.79 | ||||
Our ability to make rapid changes in product offering is better than industry average | 0.96 |
Sample demographics
n | % | |
---|---|---|
Industry | ||
Chemicals, petroleum, rubber and plastic | 27 | 13.9 |
Food manufacturing | 19 | 9.8 |
Industrial, electric and electronic machinery | 44 | 22.7 |
Leather, stone, clay and glass products | 7 | 3.6 |
Metals and metal products | 62 | 32 |
Wood, furniture and paper manufacturing | 18 | 9.3 |
Other manufacturing | 17 | 8.8 |
Number of employees | ||
<15 | 34 | 17.5 |
16–29 | 72 | 37.1 |
30–45 | 30 | 15.5 |
46–60 | 19 | 9.8 |
61–99 | 26 | 13.4 |
100–291 | 13 | 6.7 |
Turnover (thousand euros) | ||
<5 | 80 | 41.2 |
5–9.9 | 51 | 26.3 |
10–24.9 | 52 | 26.8 |
25–50 | 11 | 5.7 |
Firm age (years) | ||
<5 | 5 | 2.6 |
5–10 | 36 | 18.6 |
11–25 | 58 | 29.9 |
26–50 | 41.2 | |
<50 | 15 | 7.7 |
References
Aaldering, L.J. and Song, C.H. (2021), “Of leaders and laggards - towards digitalization of the process industries”, Technovation, Vol. 105, doi: 10.1016/j.technovation.2020.102211.
Aiken, L. and West, S. (1991), Multiple Regression: Testing and Interpreting Interactions, Sage Publications, California.
AlMulhim, A.F. (2021), “Smart supply chain and firm performance: the role of digital technologies”, Business Process Management Journal, Vol. 27 No. 5, pp. 1353-1372, doi: 10.1108/BPMJ-12-2020-0573.
Amit, R. and Schoemaker, P.J.H. (1993), “Strategic assets and organizational rent”, Strategic Management Journal, Vol. 14 No. 1, pp. 33-46, doi: 10.1002/smj.4250140105.
ArditoPetruzzelli, L.A., Panniello, U. and Garavelli, A. (2019), “Towards Industry 4.0: mapping digital technologies for supply chain management-marketing integration”, Business Process Management Journal, Vol. 25 No. 2, pp. 323-346, doi: 10.1108/BPMJ-04-2017-0088.
Ataseven, C. and Nair, A. (2017), Assessment of supply chain integration and performance relationships: a meta-analytic investigation of the literature, International Journal of Production Economics, Elsevier, Vol. 185, pp. 252-265, doi: 10.1016/j.ijpe.2017.01.007.
Autio, E., Sapienza, H.J. and Almeida, J.G. (2000), “Effects of age at entry, knowledge intensity, and imitability on international growth”, Academy of Management Journal, Vol. 43 No. 5, pp. 909-924, doi: 10.2307/1556419.
Barney, J. (1986), “Organizational culture: can it Be a source of sustained competitive advantage?”, Academy of Management Review, Vol. 11 No. 3, pp. 656-665, doi: 10.5465/amr.1986.4306261.
Barua, A., Konana, P., Whinston, A. and Yin, F. (2004), “An empirical investigation of net-enabled business value”, MIS Quarterly, Vol. 28 No. 4, pp. 585-620.
Bazan, P. and Estevez, E. (2022), “Industry 4.0 and business process management: state of the art and new challenges”, Business Process Management Journal, Vol. 28 No. 1, pp. 62-80, doi: 10.1108/BPMJ-04-2020-0163.
Bharadwaj, A.S. (2000), “A resource-based perspective on information technology capability and firm performance: an empirical investigation”, MIS Quarterly, Vol. 24 No. 1, pp. 169-196.
Bhatt, G., Wmdad, A., Roberts, N. and Grover, V. (2010), Building and leveraging information in dynamic environments: the role of IT infrastructure flexibility as enabler of organizational responsiveness and competitive advantage, Information and Management Vol. 47, No. 78 Elsevier B.V., pp. 341-349, doi: 10.1016/j.im.2010.08.001.
Bi, R., Davison, R., Kam, B. and Smyrnios, K. (2013), “Developing organizational agility through IT and supply chain capability”, Journal of Global Information Management, Vol. 21 No. 4, pp. 38-55, doi: 10.4018/jgim.2013100103.
Bienhaus, F. and Haddud, A. (2018), “Procurement 4.0: factors influencing the digitisation of procurement and supply chains”, Business Process Management Journal, Vol. 24 No. 4, pp. 965-984, doi: 10.1108/BPMJ-06-2017-0139.
Blatz, F., Bulander, R. and Dietel, M. (2018), “Maturity model of digitization for SMEs”, 2018 IEEE International Conference on Engineering, Technology and Innovation, ICE/ITMC 2018 - Proceedings, IEEE, pp. 1-9, doi: 10.1109/ICE.2018.8436251.
Bollen, K. (1989), Structural Equations with Latent Variables, John Wiley & Sons, New York.
Brown, T. (2006), Confirmatory Factor Analysis for Applied Research, Guilford Press cop, New York.
Büyüközkan, G. and Göçer, F. (2018), “Digital Supply Chain: literature review and a proposed framework for future research”, Computers in Industry, Vol. 97, pp. 157-177, doi: 10.1016/j.compind.2018.02.010.
Byrne, B. (2016), Structural Equation Modeling with AMOS: Basic Concepts, Applications, and Programming, 3rd ed., Routledge, New York.
Cámara, S.B., Fuentes, J.M. and Marín, J.M.M. (2015), “Cloud computing, Web 2.0, and operational performance: the mediating role of supply chain integration”, The International Journal of Logistics Management, Vol. 26, p. 3, doi: 10.1108/IJLM-07-2013-0085.
Calatayud, A., Mangan, J. and Christopher, M. (2019), “The self-thinking supply chain”, Supply Chain Management: An International Journal, Vol. 24 No. 1, pp. 22-38, doi: 10.1108/SCM-03-2018-0136.
Cao, G., Wiengarten, F. and Humphreys, P. (2011), “Towards a contingency resource-based view of IT business value”, Systemic Practice and Action Research, Vol. 24 No. 1, pp. 85-106, doi: 10.1007/s11213-010-9178-0.
Capon, N., Farley, J.U. and Hoenig, S. (1990), “Determinants of financial performance. A meta-analysis”, Management Science, Vol. 36 No. 10, pp. 1143-1158, doi: 10.1287/mnsc.36.10.1143.
Cenamor, J., Parida, V. and Wincent, J. (2019), “How entrepreneurial SMEs compete through digital platforms: the roles of digital platform capability, network capability and ambidexterity”, Journal of Business Research, Vol. 100, pp. 196-206, doi: 10.1016/j.jbusres.2019.03.035.
Chang, W., Ellinger, A., Kim, K. and Franke, K. (2016), “Supply chain integration and firm financial performance: a meta-analysis of positional advantage mediation and moderating factors”, European Management Journal, Vol. 34 No. 3, pp. 282-295, doi: 10.1016/j.emj.2015.11.008.
Chen, Y., Wang, Y., Nevo, S., Jin, J., Wang, L. and Chow, W. (2014), “IT capability and organizational performance: the roles of business process agility and environmental factors”, European Journal of Information Systems, Vol. 23 No. 3, pp. 326-342, doi: 10.1057/ejis.2013.4.
Chi, M., Wang, W., Lu, X. and George, J. (2018), “Antecedents and outcomes of collaborative innovation capabilities on the platform collaboration environment”, International Journal of Information Management, Vol. 43, pp. 273-283, doi: 10.1016/j.ijinfomgt.2018.08.007.
Cho, B., Ryoo, S.Y. and Kim, K.K. (2017), “Interorganizational dependence, information transparency in interorganizational information systems, and supply chain performance”, European Journal of Information Systems, Vol. 26 No. 2, pp. 185-205, doi: 10.1057/s41303-017-0038-1.
Collier, J. (2020), Applied Structural Equation Modeling Using Amos, 1st ed., Routledge, New York.
Collis, D.J. (1994), “Research note: how valuable are organizational capabilities?”, Strategic Management Journal, Vol. 15 No. 1, pp. 143-152, doi: 10.1002/smj.4250150910.
Culot, G., Nassimbeni, G., Orzes, G. and Sartor, M. (2020), “Behind the definition of Industry 4.0: analysis and open questions”, International Journal of Production Economics, Vol. 226, doi: 10.1016/j.ijpe.2020.107617.
Dalenogare, L.S., Benitez, G.B., Ayala, N.F. and Frank, A.G. (2018), “The expected contribution of Industry 4.0 technologies for industrial performance”, International Journal of Production Economics, Vol. 204, pp. 383-394, doi: 10.1016/j.ijpe.2018.08.019.
Davison, R. and Martinsons, M.G. (2002), “Empowerment or enslavement? A case of process-based organisational change in Hong Kong”, Information Technology and People, Vol. 15 No. 1, pp. 42-59, doi: 10.1108/09593840210421516.
Dong, S., Xu, S.X. and Zhu, K.X. (2009), “Information technology in supply chains: the value of IT-enabled resources under competition”, Information Systems Research, Vol. 20 No. 1, pp. 18-32, doi: 10.1287/isre.1080.0195.
Dubey, R., Gunsekaran, A., Childe, S., Doubaud, D., Wamba, S., Ginnakis, M. and Foropon, C. (2019), “Big data analytics and organizational culture as complements to swift trust and collaborative performance in the humanitarian supply chain”, International Journal of Production Economics, Vol. 210, pp. 120-136, doi: 10.1016/j.ijpe.2019.01.023.
Eller, R., Alford, P., Kallmünzer, A. and Peters, M. (2020), “Antecedents, consequences, and challenges of small and medium-sized enterprise digitalization”, Journal of Business Research, Vol. 112, pp. 119-127, doi: 10.1016/j.jbusres.2020.03.004.
Fawcett, S., Wallin, C., Allred, C., Fawcett, A. and Magnan, G. (2011), “Information technology as an enabler of supply chain collaboration: a dynamic-capabilities perspective”, Journal of Supply Chain Management, Vol. 47 No. 1, pp. 38-59, doi: 10.1111/j.1745-493X.2010.03213.x.
Fitzgerald, M., Kruschwitz, N., Bonne, D. and Welch, M. (2013), “Embracing digital technology: a new strategic imperative | Capgemini Consulting worldwide”, MIT Sloan Management Review, Vol. 55 No. 1, pp. 1-13.
Fornell, C. and Larcker, D.F. (1981), “Evaluating structural equation models with unobservable variables and measurement error”, Journal of Marketing Research, Vol. 18 No. 1, p. 39, doi: 10.2307/3151312.
Gartner (2018), Top 8 supply chain technology trends 2018, available at: https://www.gartner.com/smarterwithgartner/gartner-top-8-supply-chain-technology-trends-for-2018/ (accessed 18 June 2022).
Ghobakhloo, M. and Iranmanesh, M. (2021), “Digital transformation success under Industry 4.0: a strategic guideline for manufacturing SMEs”, Journal of Manufacturing Technology Management, Vol. 32 No. 8, pp. 1533-1556, doi: 10.1108/JMTM-11-2020-0455.
Hair, J., Black, W., Barry, B. and Anderson, R. (2019), Multivariate data Analysis, Cengage, Andover.
Hair, J.F., Ringle, C.M. and Sarstedt, M. (2011), “PLS-SEM: indeed a silver bullet”, Journal of Marketing Theory and Practice, Vol. 19 No. 2, pp. 139-152, doi: 10.2753/MTP1069-6679190202.
Hallikas, J., Immonen, M. and Brax, S. (2021), “Digitalizing procurement: the impact of data analytics on supply chain performance”, Supply Chain Management, Vol. 26 No. 5, pp. 629-646, doi: 10.1108/SCM-05-2020-0201.
Hartl, E. and Hess, T. (2017), “The role of cultural values for digital transformation: insights from a Delphi study”, AMCIS 2017 - America’s Conference on Information Systems: A Tradition of Innovation.
Hau, L., So, K. and Tang, C. (2000), “The value of information sharing in a Two-Level Supply Chain”, Management Science, Vol. 46 No. 5, pp. 626-643, doi: 10.1051/ro/2021090.
Hayes, A. (2018), Introduction to Mediation, Moderation, and Conditional Process Analysis. A Regression-Based Approach, The Guilford Press, New York.
Henseler, J., Ringle, C.M. and Sarstedt, M. (2015), “A new criterion for assessing discriminant validity in variance-based structural equation modeling”, Journal of the Academy of Marketing Science, Vol. 43 No. 1, pp. 115-135, doi: 10.1007/s11747-014-0403-8.
Holmström, J., Holweg, B., Lawson, P., Pil, F. and Wagner, S. (2019), “The digitalization of operations and supply chain management: theoretical and methodological implications”, Journal of Operations Management, Vol. 65 No. 8, pp. 728-734, doi: 10.1002/joom.1073.
James, L. and Brett, J. (1984), “Mediators, moderators, and tests for mediation”, Journal of Applied Psychology, Vol. 69 No. 2, pp. 307-321, doi: 10.1037/0021-9010.69.2.307.
James, L., Mulaik, S. and Brett, J. (2006), “A tale of two methods”, Organizational Research Methods, Vol. 9 No. 2, pp. 233-244, doi: 10.1007/s11229-008-9437-0.
Jayaram, J., Ahire, S.L. and Dreyfus, P. (2010), “Contingency relationships of firm size, TQM duration, unionization, and industry context on TQM implementation - a focus on total effects”, Journal of Operations Management, Vol. 28 No. 4, pp. 345-356, doi: 10.1016/j.jom.2009.11.009.
Jeffers, P.I., Muhanna, W.A. and Nault, B.R. (2008), “Information technology and process performance: an empirical investigation of the interaction between IT and non-IT resources”, Decision Sciences, Vol. 39 No. 4, pp. 703-735, doi: 10.1111/j.1540-5915.2008.00209.x.
Kohtamäki, M., Parida, V., Patel, P. and Gebauer, H. (2020), “The relationship between digitalization and servitization: the role of servitization in capturing the financial potential of digitalization”, Technological Forecasting and Social Change, Vol. 151, doi: 10.1016/j.techfore.2019.119804.
Kousiouris, G., Tsarsitalidis, S., Psomakelis, E., Koloniaris, S., Bardaki, C., Tserpes, K., Nikolaidou, M. and Anagnostopoulos, D. (2019), “A microservice-based framework for integrating IoT management platforms, semantic and AI services for supply chain management”, ICT Express, Vol. 5 No. 2, pp. 141-145, doi: 10.1016/j.icte.2019.04.002.
Lee, K.L., Azmi, N., Hanayasha, J., Alzoubi, H. and Alshurieh, M. (2022), “The effect of digital supply chain on organizational performance: an empirical study in Malaysia manufacturing industry”, Uncertain Supply Chain Management, Vol. 10 No. 2, pp. 495-510, doi: 10.5267/j.uscm.2021.12.002.
Leidner, D.E. and Kayworth, T. (2006), “Review: a review of culture in information systems research: toward a theory of information technology culture conflict”, MIS Quarterly: Management Information Systems, Vol. 30 No. 2, pp. 357-399, doi: 10.2307/25148735.
Leuschner, R., Rogers, D.S. and Charvet, F.F. (2013), “A meta-analysis of supply chain integration and firm performance”, Journal of Supply Chain Management, Vol. 49 No. 2, pp. 34-57, doi: 10.1111/jscm.12013.
Li, Y., Dai, J. and Cui, L. (2020), “The impact of digital technologies on economic and environmental performance in the context of industry 4.0: a moderated mediation model”, International Journal of Production Economics, Vol. 229, 107777, doi: 10.1016/j.ijpe.2020.107777.
Liang, T.P., You, J.J. and Liu, C.C. (2010), “A resource-based perspective on information technology and firm performance: a meta analysis”, Industrial Management and Data Systems, Vol. 110 No. 8, pp. 1138-1158, doi: 10.1108/02635571011077807.
Liao, S.H., Hu, D.C. and Ding, L.W. (2017), “Assessing the influence of supply chain collaboration value innovation, supply chain capability and competitive advantage in Taiwan's networking communication industry”, International Journal of Production Economics, Vol. 191, pp. 143-153, doi: 10.1016/j.ijpe.2017.06.001.
Liu, H., Ke, W., Wei, K., Gu, J. and Chen, H. (2010), “The role of institutional pressures and organizational culture in the firm's intention to adopt internet-enabled supply chain management systems”, Journal of Operations Management, Vol. 28 No. 5, pp. 372-384, doi: 10.1016/j.jom.2009.11.010.
Malhotra, N. (2010), Marketing Research an Applied Orientation, Pearson Publishing, London.
Markus, M.L. and Loebbecke, C. (2013), “Commoditized digital processes and business community platforms: new opportunities and challenges for digital business strategies”, MIS Quarterly Executive, Vol. 37, pp. 694-653.
Martínez-Caro, E., Cegarra-Navarro, J.G. and Alfonso-Ruiz, F.J. (2020), “Digital technologies and firm performance: the role of digital organisational culture”, Technological Forecasting and Social Change, Vol. 154 No. June, 119962, doi: 10.1016/j.techfore.2020.119962.
Mata, F.J., Fuerst, W.L. and Barney, J.B. (1995), “Information technology and sustained competitive advantage: a resource-based analysis”, MIS Quarterly: Management Information Systems, Vol. 19 No. 4, pp. 487-504, doi: 10.2307/249630.
Melville, N., Kraemer, K. and Gurbaxani, V. (2004), “Review: information technology and organizational performance: an integrative model of IT business value”, MIS Quarterly, Vol. 28 No. 2, pp. 283-322.
Mikalef, P., Krogstie, J., Pappas, I. and Pavlou, P. (2020), “Exploring the relationship between big data analytics capability and competitive performance: the mediating roles of dynamic and operational capabilities”, Information and Management, Vol. 57 No. 2, doi: 10.1016/j.im.2019.05.004.
Miller, D. (1993), “The architecture of simplicity”, Academy of Management Review, Vol. 18 No. 1, pp. 116-138, doi: 10.5465/amr.1993.3997509.
Mishra, A.N., Konana, P. and Barua, A. (2007), “Antecedents and consequences of Internet use in procurement: an empirical investigation of U.S. manufacturing firms”, Information Systems Research, Vol. 18 No. 1, pp. 103-120, doi: 10.1287/isre.1070.0115.
Mithas, S., Ramasubbu, N. and Sambamurthy, V. (2011), “How information management capability influences firm performance”, MIS Quarterly, Vol. 35 No. 1, pp. 237-256.
Morash, E. (2001), “Supply chain strategies, capabilities, and performance”, Transportation Journal, Vol. 41 No. 1, pp. 37-54.
Morash, E.A. and Lynch, D.F. (2002), “Public policy and global supply chain capabilities and performance: a resource-based view”, Journal of International Marketing, Vol. 10 No. 1, pp. 25-51, doi: 10.1509/jimk.10.1.25.19529.
Morash, E., Dröge, C. and Vickery, S. (1996), “Strategic logistics capabilities for competitive advantage and firm success”, Journal of Business Logistics, Vol. 17 No. 1, pp. 1-22.
Nadkarni, S. and Prügl, R. (2020), “Digital transformation: a review, synthesis and opportunities for future research”, Management Review Quarterly, Vol. 71, pp. 233-241, doi: 10.1007/s11301-020-00185-7.
Podsakoff, P.M., MacKenzie, S.B., Lee, J.Y. and Podsakoff, N.P. (2003), “Common method biases in behavioral research: a critical review of the literature and recommended remedies”, Journal of Applied Psychology, Vol. 88 No. 5, pp. 879-903, doi: 10.1037/0021-9010.88.5.879.
Podsakoff, P.M. and Organ, D.W. (1986), “Self-reports in organizational research: problems and prospects”, Journal of Management, Vol. 12 No. 4, pp. 531-544, doi: 10.1177/014920638601200408.
Rai, A., Patnayakuni, R. and Seth, N. (2006), “Firm performance impacts of digitally enabled supply chain integration capabilities”, MIS Quarterly: Management Information Systems, Vol. 30 No. 2, pp. 225-246, doi: 10.2307/25148729.
Rajaguru, R. and Matanda, M.J. (2019), “Role of compatibility and supply chain process integration in facilitating supply chain capabilities and organizational performance”, Supply Chain Management: An International Journal, Vol. 24 No. 2, pp. 315-330, doi: 10.1108/SCM-05-2017-0187.
Rosenzweig, E.D. (2009), “A contingent view of e-collaboration and performance in manufacturing”, Journal of Operations Management, Vol. 27 No. 6, pp. 462-478, doi: 10.1016/j.jom.2009.03.001.
Rueda-Manzanares, A., Aragón-Correa, J.A. and Sharma, S. (2008), “The influence of stakeholders on the environmental strategy of service firms: the moderating effects of complexity, uncertainty and munificence”, British Journal of Management, Vol. 19 No. 2, pp. 185-203, doi: 10.1111/j.1467-8551.2007.00538.x.
Sedera, D., Lokuge, S., Grover, V., Sarker, S. and Sarker, S. (2016), “Innovating with enterprise systems and digital platforms: a contingent resource-based theory view”, Information and Management, Vol. 53 No. 3, pp. 366-379, doi: 10.1016/j.im.2016.01.001.
Seyedghorban, Z., Tahernejad, H., Meriton, R. and Graham , G. (2020), “Supply chain digitalization: past, present and future”, Production Planning and Control, Vol. 31 Nos 2-3, pp. 96-114, doi: 10.1080/09537287.2019.1631461.
Sideridis, G., Simons, P., Papanicolaou, A. and Fletcher, J. (2014), “Using structural equation modeling to assess functional connectivity in the brain: power and sample size considerations”, Educational and Psychological Measurement, Vol. 74 No. 5, pp. 733-758, doi: 10.1177/0013164414525397.
Singh, S.K. and El-Kassar, A.N. (2019), “Role of big data analytics in developing sustainable capabilities”, Journal of Cleaner Production, Vol. 213, pp. 1264-1273, doi: 10.1016/j.jclepro.2018.12.199.
Tippins, M.J. and Sohi, R.S. (2003), “IT competency and firm performance: is organizational learning a missing link?”, Strategic Management Journal, Vol. 24 No. 8, pp. 745-761, doi: 10.1002/smj.337.
Verhoef, P., Broekhuizen, T., Bart, Y., Bhattacharya, A., Dong, J., Fabian, N. and Haenlein, M. (2021), “Digital transformation: a multidisciplinary reflection and research agenda”, Journal of Business Research, Vol. 122 November, pp. 889-901, doi: 10.1016/j.jbusres.2019.09.022.
Wade, M. and Hulland, J. (2004), “Review: the resource-based view and information systems research: review, extension, and suggestions for future research”, MIS Quarterly, Vol. 28 No. 1, pp. 107-142.
Wagire, A.A., Rathore, A.P.S. and Jain, R. (2020), “Analysis and synthesis of Industry 4.0 research landscape: using latent semantic analysis approach”, Journal of Manufacturing Technology Management, Vol. 31 No. 1, pp. 31-51, doi: 10.1108/JMTM-10-2018-0349.
Wamba, S.F. and Queiroz, M.M. (2020), “Industry 4.0 and the supply chain digitalisation: a blockchain diffusion perspective”, Production Planning and Control, Vol. 33 Nos 2-3, pp. 193-210, doi: 10.1080/09537287.2020.1810756.
Ward, P.T. and Duray, R. (2000), “Manufacturing strategy in context: environment, competitive strategy and manufacturing strategy”, Journal of Operations Management, Vol. 18 No. 2, pp. 123-138, doi: 10.1016/S0272-6963(99)00021-2.
Wei, S., Ke, W., Liu, H. and Wei, K. (2020), “Supply chain information integration and firm performance: are explorative and exploitative IT capabilities complementary or substitutive?”, Decision Sciences, Vol. 51 No. 3, pp. 464-499, doi: 10.1111/deci.12364.
Wiengarten, F., Humphreys, P., Cao, C. and McHugh, M. (2013), “Exploring the important role of organizational factors in IT business value: taking a contingency perspective on the resource-based view”, International Journal of Management Reviews, Vol. 15 No. 1, pp. 30-46, doi: 10.1111/j.1468-2370.2012.00332.x.
Witschel, D., Döhla, A., Kaiser, M., Voigt, K. and Pfletschinger, T. (2019), “Riding on the wave of digitization: insights how and under what settings dynamic capabilities facilitate digital-driven business model change”, Journal of Business Economics, Vol. 89 No. 8, pp. 1023-1095, doi: 10.1007/s11573-019-00950-5.
Wolf, E.J., Harrington, K., Clark, S. and Miller, M. (2013), “Sample size requirements for structural equation models: an evaluation of power, bias, and solution propriety”, Educational and Psychological Measurement, Vol. 73 No. 6, pp. 913-934, doi: 10.1177/0013164413495237.
Wong, C.Y., Boon-Itt, S. and Wong, C.W.Y. (2011), “The contingency effects of environmental uncertainty on the relationship between supply chain integration and operational performance”, Journal of Operations Management, Vol. 29 No. 6, pp. 604-615, doi: 10.1016/j.jom.2011.01.003.
Wong, C.W.Y., Lai, K.H. and Bernroider, E.W.N. (2015), “The performance of contingencies of supply chain information integration: the roles of product and market complexity”, International Journal of Production Economics, Vol. 165, pp. 1-11, doi: 10.1016/j.ijpe.2015.03.005.
Wu, F., Yeniurt, S., Kim, D. and Cavusigil, T. (2006), “The impact of information technology on supply chain capabilities and firm performance: a resource-based view”, Industrial Marketing Management, Vol. 35 No. 4, pp. 493-504, doi: 10.1016/j.indmarman.2005.05.003.
Yang, L., Yang, S.H. and Plotnick, L. (2013), “How the internet of things technology enhances emergency response operations”, Technological Forecasting and Social Change, Vol. 80 No. 9, pp. 1854-1867, doi: 10.1016/j.techfore.2012.07.011.
Yang, M., Fu, M. and Zhang, Z. (2021), “The adoption of digital technologies in supply chains: drivers, process and impact”, Technological Forecasting and Social Change, Vol. 169 No. 1, pp. 1-13, doi: 10.1016/j.techfore.2021.120795.
Yu, W., Chavez, R., Jacobs, M. and Feng, M. (2018), “Data-driven supply chain capabilities and performance: a resource-based view”, Transportation Research Part E: Logistics and Transportation Review, Vol. 114, pp. 371-385, doi: 10.1016/j.tre.2017.04.002.
Yu, Y., Huo, B. and Zhang, Z. (2020), “Impact of information technology on supply chain integration and company performance: evidence from cross-border e-commerce companies in China”, Journal of Enterprise Information Management, Vol. 34 No. 1, doi: 10.1108/JEIM-03-2020-0101.
Yu, W., Wong, C., Chavez, R. and Jacobs, M. (2021), “Integrating big data analytics into supply chain finance: the roles of information processing and data-driven culture”, International Journal of Production, Vol. 236 No. June, 108135, doi: 10.1016/j.ijpe.2021.108135.
Yusuf, Y., Gunasekaran, A., Adeleye, E. and Sivayoganathan, K. (2004), “Agile supply chain capabilities: determinants of competitive objectives”, European Journal of Operational Research, Vol. 159, pp. 379-392, doi: 10.1016/j.ejor.2003.08.022.
Zhao, X., Lynch, J. and Chen, Q. (2010), “Reconsidering baron and Kenny: myths and truths about mediation analysis”, Journal of Consumer Research, Vol. 37 No. August, pp. 197-206, doi: 10.1086/651257.
Zhu, K. and Kraemer, K.L. (2002), “e-Commerce metrics for net-enhanced organizations: assessing the value of e-commerce to firm performance in the manufacturing sector”, Information Systems Research, Vol. 13 No. 3, pp. 275-295, doi: 10.1287/isre.13.3.275.82.
Acknowledgements
The author gratefully acknowledges the financial support from the Foundation of Economic Education, Finnish Cultural fund and University of Vaasa. In addition, author wants to thank the reviewers for their suggested valuable and thorough feedback, which improved the contents of this paper.
Corresponding author
About the author
Tuire Hautala-Kankaanpää is a grant-funded researcher in Management at the University of Vaasa and an independent researcher funded by the Foundation of Economic Education. She is a member of the Strategic Business Development research group. Her research interest includes the impacts of digitalization at the firm and supply chain levels.